A Recurrent Neural Network Optimization Method for Anticipation of Hierarchical Human Activity
IEEE TRANSACTIONS ON AUTOMATION SCIENCE AND ENGINEERING(2023)
摘要
Human activity anticipation is a pillar of new interactive multimedia technologies. It enables better human-computer interaction and creative sensory experience in all types of virtual reality-based multimedia applications. Human behaviors are hierarchically constituted in terms of low-level and high-level activities. Fine-grained activities play a substantial role in the early recognition of simple and complex human movements in real-time with low latency, allowing longer predictions in the future. In this paper, a new objective function is proposed to anticipate fine-grained human activities using IMU data, which still suffers from an imbalance problem. Four customized objective surrogate functions are applied in unidirectional and bidirectional recurrent neural networks and compared to efficiently optimize the model considering the loss of individual classes. The experiments on five datasets across proposed loss functions show that the proposed model significantly outperforms the counterpart methods and advances the state-of-the-art. The proposed model shows accuracy scores of up to 98% and 96% for high-level and low-level activities, respectively. Note to Practitioners-There are a large number of methods that can be used for the recognition of human activity in assisted living, smart home technologies, and pervasive healthcare applications. However, studies on these methods tend to focus on recognition rather than anticipation of human activity. Furthermore, they use either vision-based or egocentric video data, which can make it challenging to collect and evaluate in virtual reality-based scenarios. To enable more natural interaction with physical and virtual reality environments, virtual reality-based technologies require built-in algorithms capable of consistently identifying complex and varied human movements. Fine-grained activities are paramount in predicting activities at an early stage to adequately characterize human behaviors. In this paper, a study is conducted to anticipate fine-grained human activity based on IMU (Inertial Measurement Unit) data, which are still subject to the negative effects of an imbalanced sample distribution. The proposed model is evaluated on different benchmarks and compared with baseline methods. The results show that the proposed model outperforms all baselines. These results have important implications for human activity prediction problems.
更多查看译文
关键词
hierarchical human activity,anticipation,neural network
AI 理解论文
溯源树
样例
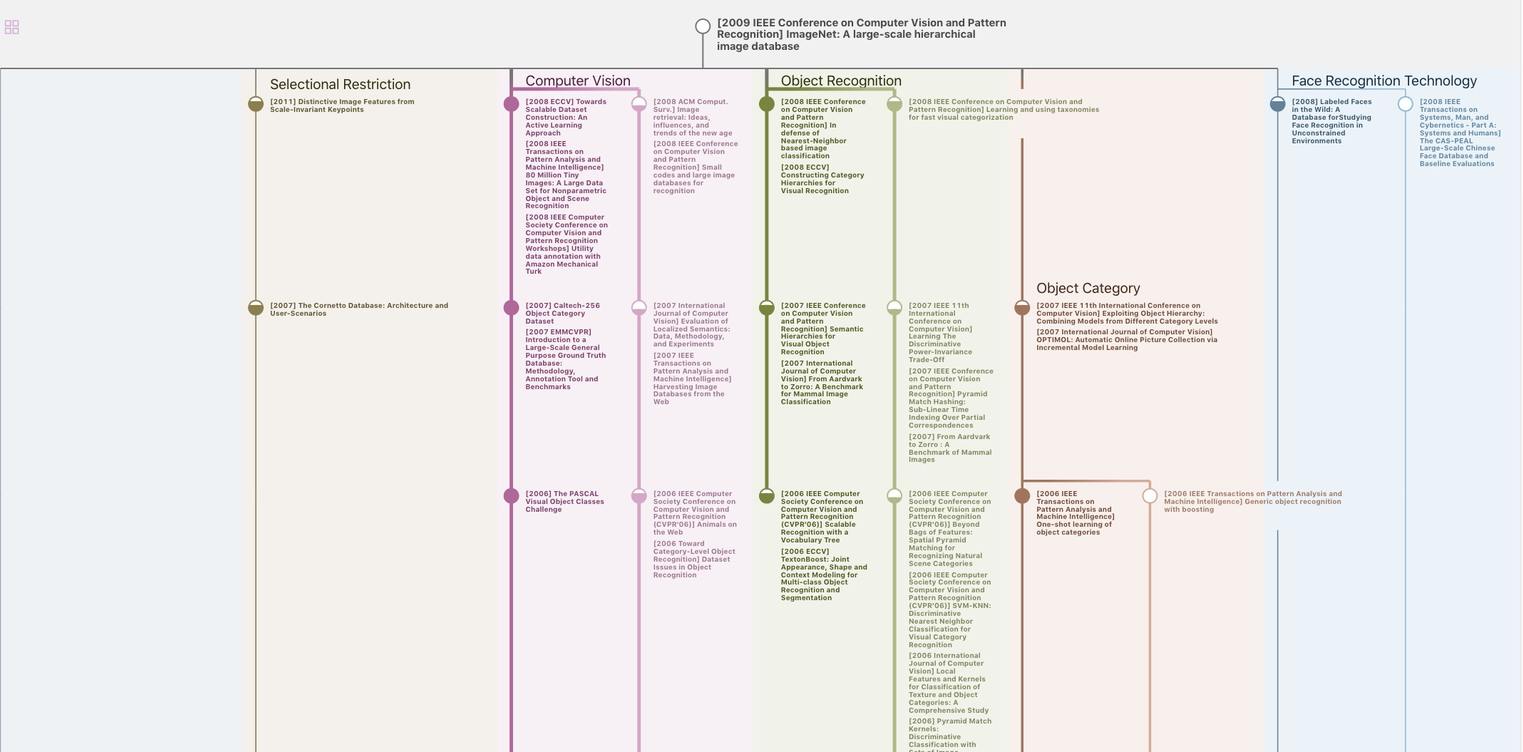
生成溯源树,研究论文发展脉络
Chat Paper
正在生成论文摘要