GRSR-a guideline for reporting studies results for machine learning applied to Electroencephalogram data
REVISTA BRASILEIRA DE COMPUTACAO APLICADA(2023)
摘要
The last decade was marked by increased neuroscience research involving machine Learning (ML) and medical images such as electroencephalogram (EEG). Since ML models tend to be sensitive to the input data, different strategies for experiment design significantly impact the results achieved. Therefore, the suppression of information about design and results makes comparing works challenging. On average, 53% of critical data was missing from the papers retrieved, making it hard to produce a fair comparison and results analysis; all papers retrieved would be considered with a high "risk of bias" and as having "concerns regarding applicability" by a Quadas-2 analysis. This corroborates the lack of a guideline to provide a standard model for data reports on the field. This work presents the GRSR, a guideline protocol to support primary studies covering critical data for studies to demonstrate when using EEG and ML to address neurological disorders. Using GRSR can reduce the chance of being evaluated as having a high risk of bias and having concern regarding applicability based on the metrics of Quadas-2. This improves the research field by allowing real comparison between reported results, narrowing the search for the best methods for neural disorders diagnoses using ML and EEG.
更多查看译文
关键词
Machine Learning, Electroencephalogram, standard presentation, ML, EEG
AI 理解论文
溯源树
样例
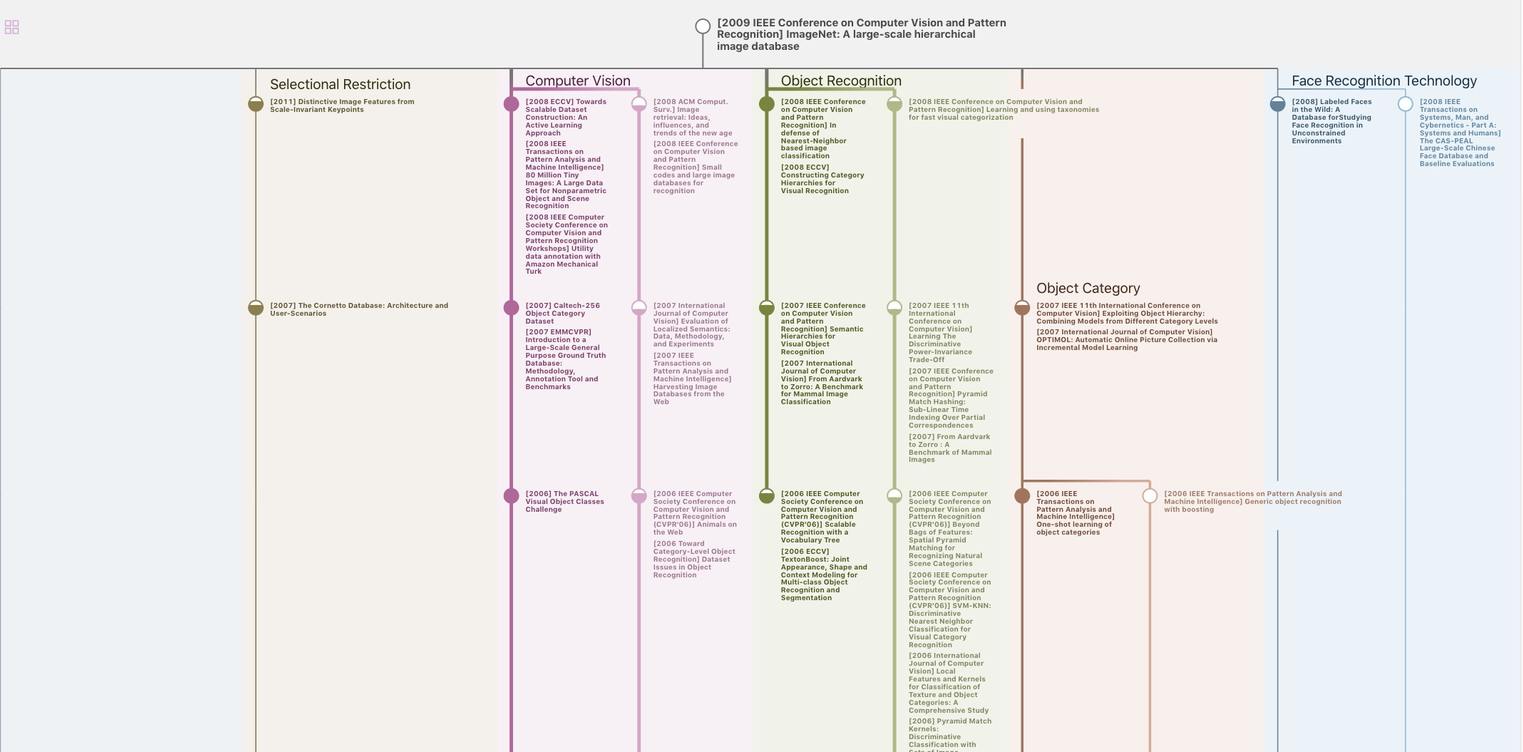
生成溯源树,研究论文发展脉络
Chat Paper
正在生成论文摘要