Improving Atmospheric Models by Accounting for Chaotic Physics
JOURNAL OF CLIMATE(2023)
摘要
It is well known that randomly perturbing an atmospheric model's diabatic tendencies can increase its prob-abilistic forecast skill, mainly by increasing the spread of ensemble forecasts and making it more consistent with the errors of ensemble-mean forecasts. Less obvious and less well established is that such perturbations can also reduce the errors of the ensemble-mean forecasts and improve the model's mean climate, variability, and sensitivity to forcing. A clear reduc-tion in ensemble-mean forecast errors is demonstrated here in large ensembles of 15-day forecasts made with NOAA's Global Forecast System model. The nearly ubiquitous reduction around the globe, obtained throughout the forecast range, is interpreted as arising in effect from a modification of the model's deterministic evolution operator by a stochastic noise-induced drift. The effect is general in systems with state-dependent noise, and occurs even if the noise is not white. In the atmospheric context considered here, the effect is suggested to arise largely from noise-induced reductions of mechanical and thermal damping by chaotic boundary layer and cloud-radiative processes, which also tend to increase model sensitiv-ity to forcing. The results presented here are consistent with many previous studies performed with models ranging from simple stochastically forced models to comprehensive global weather and climate models. They suggest that the diabatic in-teractions in most current global atmospheric models may not be sufficiently chaotic and this deficiency could be partly remedied by specifying additional stochastic terms. Using some empirical guidance in such specifications may be unavoid-able, given the generally intractable complexity of the diabatic interactions.
更多查看译文
关键词
atmospheric models,chaotic physics
AI 理解论文
溯源树
样例
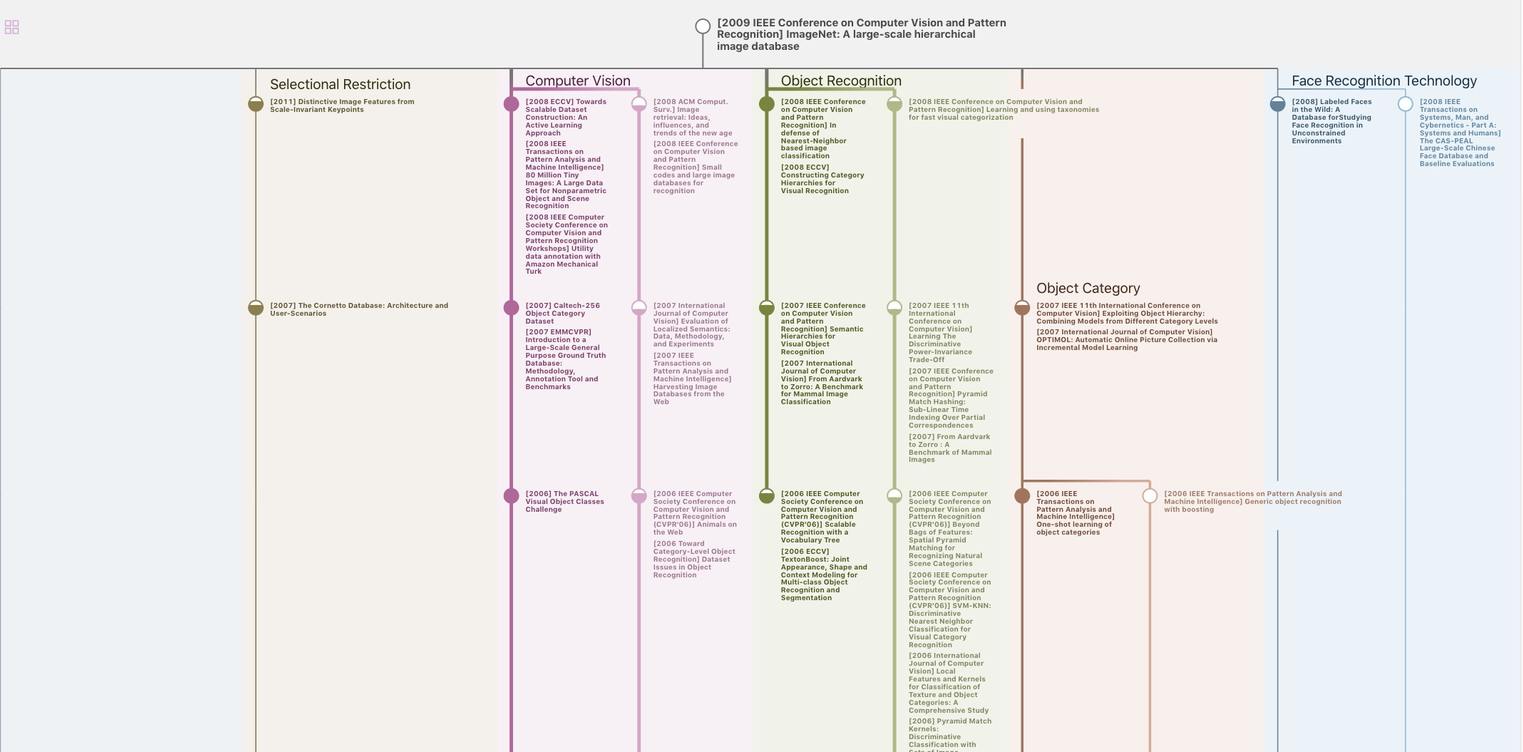
生成溯源树,研究论文发展脉络
Chat Paper
正在生成论文摘要