Decomposing and recovering airborne radiometric data through principal component analysis applied on flight-line data: An alternative to reduce noise
INTERPRETATION-A JOURNAL OF SUBSURFACE CHARACTERIZATION(2023)
摘要
Airborne gamma-ray spectrometry (AGRS) data provide valuable information about the distribution of radiometric elements on earth's surface. However, the presence of noise can hinder the interpretation or the identification of subtle variations of radioelement concentrations that can be economically attractive. Previous research has demonstrated that techniques based on matrix factorization, such as noise-adjusted singular value decomposition (NASDV) and minima noise fraction (MNF), can reduce noise when applied to AGRS raw spectra. Nevertheless, the raw spectra often are unavailable for end users, limiting the widespread adoption of such techniques. In this context, we use principal component analysis with the flight-line data before interpolating the data onto a regular grid as a means to reduce noise when the raw spectra are no longer available. We use our approach on two AGRS data sets located in Brazil and one in the United States. For Brazil's AGRS data, results indicate that noise can be attenuated through eigendecomposition projection and recovery of the radiometric variables. Furthermore, our technique can highlight some geologic features dependent on the number of eigenvectors used to reconstruct the database. For the U.S. AGRS data set previously filtered with NASDV, our methodology seems to produce only marginal improvement. Therefore, our methodology might be particularly successful for AGRS data whose acquisitions were conducted before NASDV and MNF were proposed as radiometric data processing techniques.
更多查看译文
关键词
airborne radiometric data,principal component analysis,flight-line
AI 理解论文
溯源树
样例
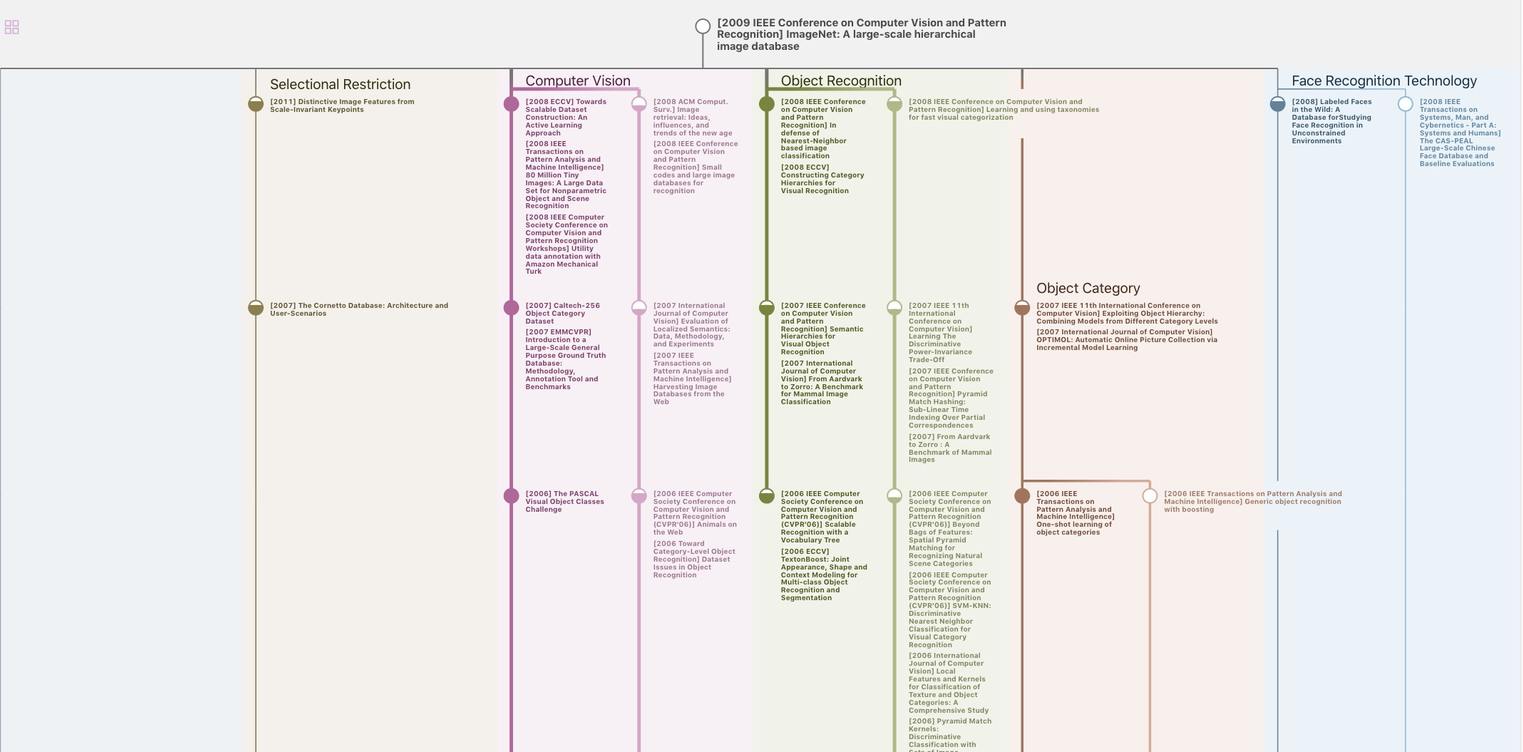
生成溯源树,研究论文发展脉络
Chat Paper
正在生成论文摘要