The Development of a Consensus Machine Learning Model for Hurricane Rapid Intensification Forecasts with Hurricane Weather Research and Forecasting
WEATHER AND FORECASTING(2023)
摘要
This study focused on developing a consensus machine learning (CML) model for tropical cyclone (TC) intensity-change forecasting, especially for rapid intensification (RI). This CML model was built upon selected classical machine learning models with the input data extracted from a high-resolution hurricane model, the Hurricane Weather Research and Forecasting (HWRF) system. The input data contained 21 or 34 RI-related predictors extracted from the 2018 version of HWRF (H218). This study found that TC inner-core predictors can be critical for improving RI predictions, especially the inner-core relative humidity. Moreover, this study emphasized the importance of performing resampling on an imbalanced input dataset. Edited nearest-neighbor and synthetic minority oversampling techniques improved the probability of detection (POD) by -10% for the RI class. This study also showed that the CML model has satisfactory performance on RI predictions compared to the operational models. CML reached 56% POD and 46% false alarm ratio (FAR), while the operational models had only 10%-30% POD but 50%-60% FAR. The CML performance on the non-RI classes was comparable to the operational models. The results indicated that, with proper and sufficient training data and RI-related predictors, CML has the potential to provide reliable probabilistic RI forecasts during a hurricane season.
更多查看译文
关键词
Hurricanes, typhoons, Machine learning, Regression, Intensification, Data mining
AI 理解论文
溯源树
样例
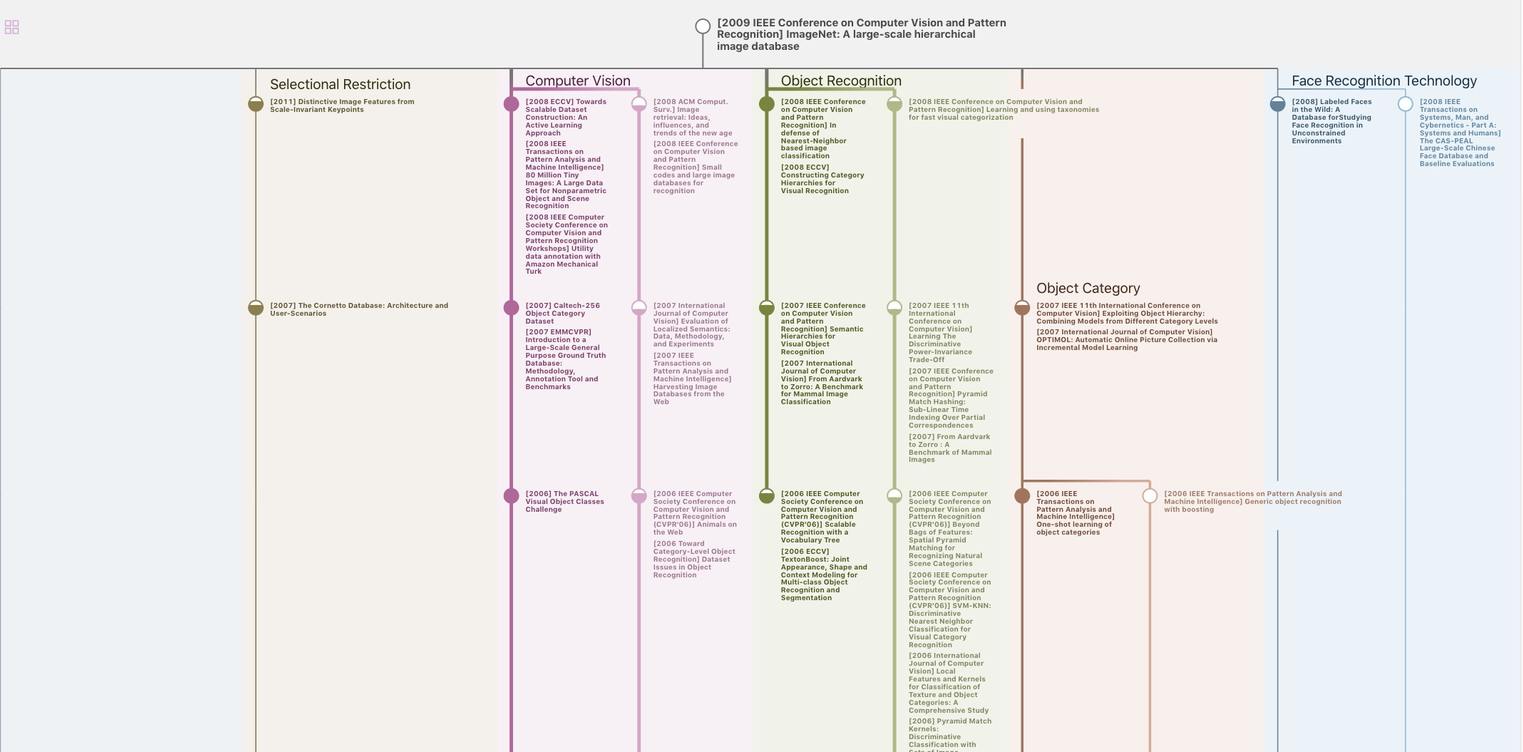
生成溯源树,研究论文发展脉络
Chat Paper
正在生成论文摘要