Machine learning (ML) model for prediction of pneumonitis using real-world data (RWD) on immune checkpoint inhibitor (ICI)-treated advanced non-small cell lung cancer (aNSCLC)
CANCER RESEARCH(2023)
摘要
Abstract Background: Immune-related pneumonitis (IRP) is a rare adverse event (AE) associated with ICIs that may result in treatment interruption or death if not managed properly. IRP prediction is critical to identify at-risk patients and estimate background IRP risk for single-arm trials. ML modeling was used on electronic health records (EHR) to predict IRP risk in patients with aNSCLC using various IRP definitions. Methods: Patients with curated aNSCLC treated with ICIs between Jan 2015 - May 2022 were identified in a US-based oncology EHR database (ConcertAI®, Cambridge, MA). Survival XGBoost models were trained with 4-fold cross-validation. Due to lack of consensus on the IRP definition using RWD, putative IRPs were derived from a mix of human-curated AEs and ICD diagnosis (dx) codes (preexisting [<90 days of ICI start] codes excluded). Three IRP definitions were used: expert-curated (IRP1, n=237 IRP events), literature-derived (IRP2, n=445), and a broad definition (IRP3, n=1213). Risk factors were identified using SHapley Additive exPlanations (SHAP) values and marginal hazard ratios. Results: The study cohort had 8928 patients. Validation cumulative/dynamic area under the ROC curve for IRP1-3 was 0.68, 0.70, and 0.71, respectively. Common across IRP definitions were predictors related to underlying lung conditions, comorbidities, polypharmacy, systemic inflammation, and de novo metastases (Table 1). Chemoradiation was a risk factor for the narrow definition (IRP1), while labs suggestive of organ dysfunction were predictive for broader definitions (IRP2-3). Unsupervised clustering of SHAP resulted in 4 patient clusters. Higher-risk clusters were driven by polypharmacy (IRP1-3), reduced platelets (IRP1), and comorbidities (IRP2-3). Conclusions: ML on different IRP definitions from RWD differentiated risk factors of pneumonitis from those inherent to lung disease among aNSCLC patients. Table 1. Risk factors for the 3 different IRP definitions as identified by ML models IRP1: Mean HR (95% CI) IRP2: Mean HR (95% CI) IRP3: Mean HR (95% CI) Comorbidities: Number of comorbidities ≥4 (vs 0) 1.17 (1.00, 1.49) 1.51 (1.30, 1.77) 1.73 (1.36, 2.22) Comorbidities: BMI ≥29.07 (vs ≤21.95) 1.25 (1.13, 1.45) 1.27 (1.17, 1.38) 1.27 (1.06, 1.53) Polypharmacy: Number of concomitant meds ≥13 (vs ≤5) 1.45 (1.17, 1.84) 1.62 (1.19, 2.11) 1.29 (1.06, 1.69) Polypharmacy: Local anesthesia (vs no) 1.12 (1.0, 1.23) 1.02 (1.00, 1.06) 1.13 (1.04, 1.21) Polypharmacy: Anti-arrhythmia agents (vs no) 1.07 (0.99, 1.14) 1.00 (1.00, 1.03) 1.00 (0.97, 1.02) Polypharmacy: Anticoagulants (vs no) 1.02 (1.00, 1.10) 1.12 (1.06, 1.21) 1.19 (1.03, 1.34) History of chemoradiation: Durvalumab line of therapy (vs no) 1.18 (1.00, 1.41) 1.00 (1.00, 1.02) 0.98 (0.89, 1.00) History of chemoradiation: Baseline thoracic radiation (vs no) 1.08 (1.00, 1.35) 1.01 (1.00, 1.03) 1.00 (0.97, 1.00) History of chemoradiation: Prior chemotherapy (vs no) 1.06 (0.99, 1.19) 1.00 (0.99, 1.01) 0.91 (0.86, 0.98) History of chemoradiation: Platelets count (103/mL) ≥343 (vs ≤203) 0.79 (0.67, 0.90) 0.97 (0.91, 1.06) 0.96 (0.86, 1.07) De novo metastasis diagnosis: M0 stage at initial diagnosis (vs M1) 1.08 (1.00, 1.21) 1.11 (1.00, 1.24) 1.08 (1.02, 1.15) De novo metastasis diagnosis: Lactate dehydrogenase (IU/L) ≥323.5 (vs ≤165) 0.87 (0.73, 0.96) 0.88 (0.75, 1.00) 0.88 (0.73, 1.02) Underlying lung conditions: Oxygen saturation (%) ≥98 (vs ≤94) 0.89 (0.72, 1.0) 1.00 (0.93, 1.05) 0.96 (0.83, 1.05) Underlying lung conditions: Respiratory rate (breaths/min) ≥18 (vs ≤16) 1.09 (0.97, 1.31) 1.08 (1.02, 1.17) 1.12 (0.99, 1.32) Organ dysfunction: Blood urea nitrogen (mg/dL) ≥20 (vs ≤11.7) 1.01 (0.86, 1.19) 1.13 (0.9, 1.58) 1.11 (0.96, 1.46) Organ dysfunction: Albumin (g/dL) ≥4 (vs ≤3.35) 0.97 (0.85, 1.11) 0.95 (0.90, 0.99) 0.83 (0.72, 0.94) Organ dysfunction: Serum protein (g/dL) ≥7.4 (vs ≤6.5) 1.06 (0.95, 1.18) 1.09 (1.02, 1.17) 1.22 (1.02, 1.44) Systemic inflammation: Neutrophils-lymphocytes ratio ≥8.59 (vs ≤3.12) 1.13 (0.99, 1.07) 1.18 (1.04, 1.30) 1.20 (1.00, 1.44) Systemic inflammation: WBC counts (103/mL) ≥10.88 (vs ≤6.10) 1.01 (0.88, 1.14) 1.05 (0.99, 1.15) 1.10 (0.95, 1.35) Footnote: BMI, body mass index; CI, confidence interval; HR, hazard ratio; IRP1 expert-curated definition, n=237; IRP2, literature-derived definition, n=445; IRP3, a broad IRP definition, n=1213; WBC, white blood cell. Citation Format: Deep K. Hathi, Heather Hall, Judith Mueller, Mona H. Cai, Yangyang Liu, Alexander Liede, Rahul K. Das. Machine learning (ML) model for prediction of pneumonitis using real-world data (RWD) on immune checkpoint inhibitor (ICI)-treated advanced non-small cell lung cancer (aNSCLC) [abstract]. In: Proceedings of the American Association for Cancer Research Annual Meeting 2023; Part 1 (Regular and Invited Abstracts); 2023 Apr 14-19; Orlando, FL. Philadelphia (PA): AACR; Cancer Res 2023;83(7_Suppl):Abstract nr 851.
更多查看译文
关键词
immune checkpoint inhibitor,machine learning,lung cancer,pneumonitis,prediction,real-world,non-small
AI 理解论文
溯源树
样例
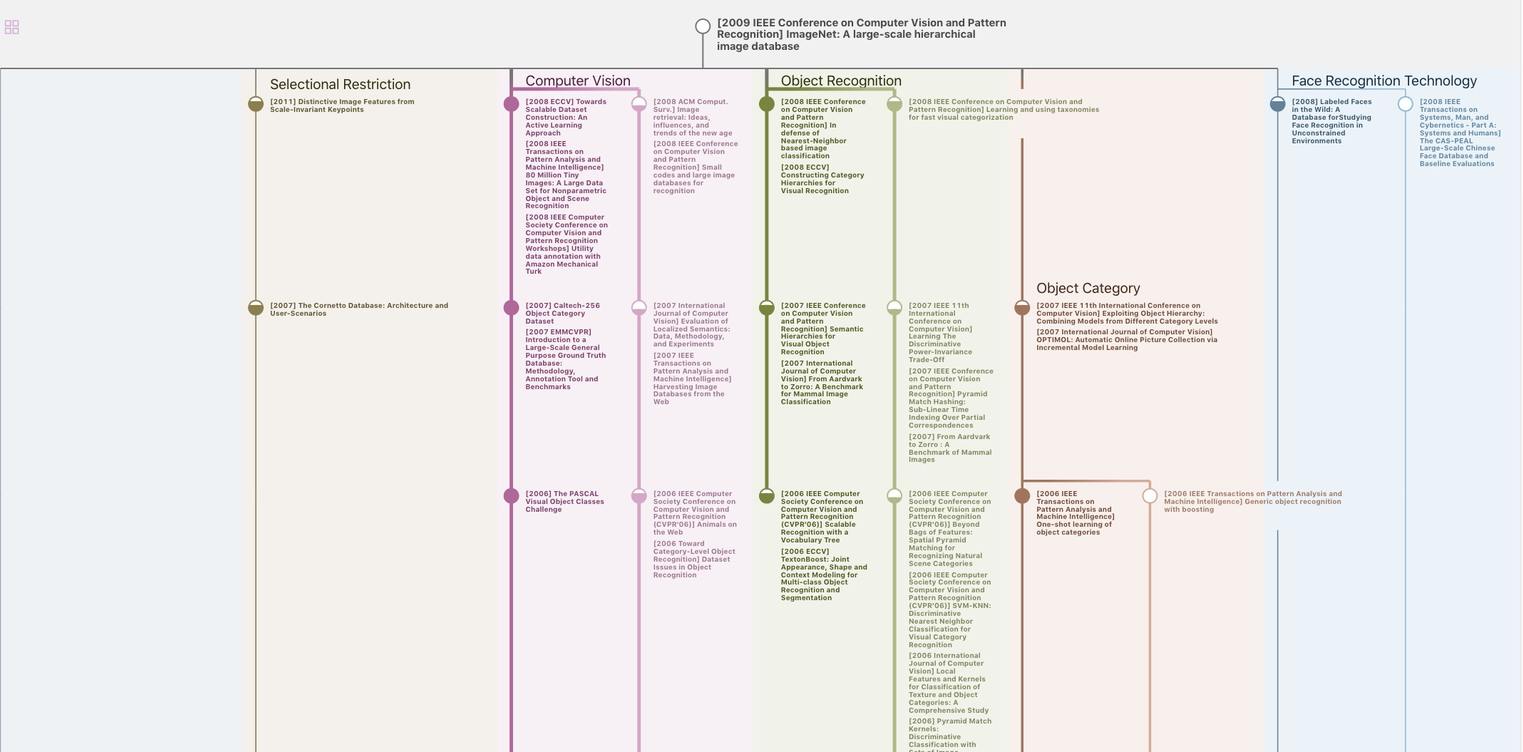
生成溯源树,研究论文发展脉络
Chat Paper
正在生成论文摘要