Distributed accelerated nash equilibrium learning for two-subnetwork zero-sum game with bilinear coupling
KYBERNETIKA(2023)
摘要
This paper proposes a distributed accelerated first-order continuous-time algorithm for O(1/t2) convergence to Nash equilibria in a class of two-subnetwork zero-sum games with bi-linear couplings. First-order methods, which only use subgradients of functions, are frequently used in distributed/parallel algorithms for solving large-scale and big-data problems due to their simple structures. However, in the worst cases, first-order methods for two-subnetwork zero-sum games often have an asymptotic or O(1/t) convergence. In contrast to existing time -invariant first-order methods, this paper designs a distributed accelerated algorithm by combin-ing saddle-point dynamics and time-varying derivative feedback techniques. If the parameters of the proposed algorithm are suitable, the algorithm owns O(1/t2) convergence in terms of the duality gap function without any uniform or strong convexity requirement. Numerical simulations show the efficacy of the algorithm.
更多查看译文
关键词
nash equilibrium,learning,two-subnetwork,zero-sum
AI 理解论文
溯源树
样例
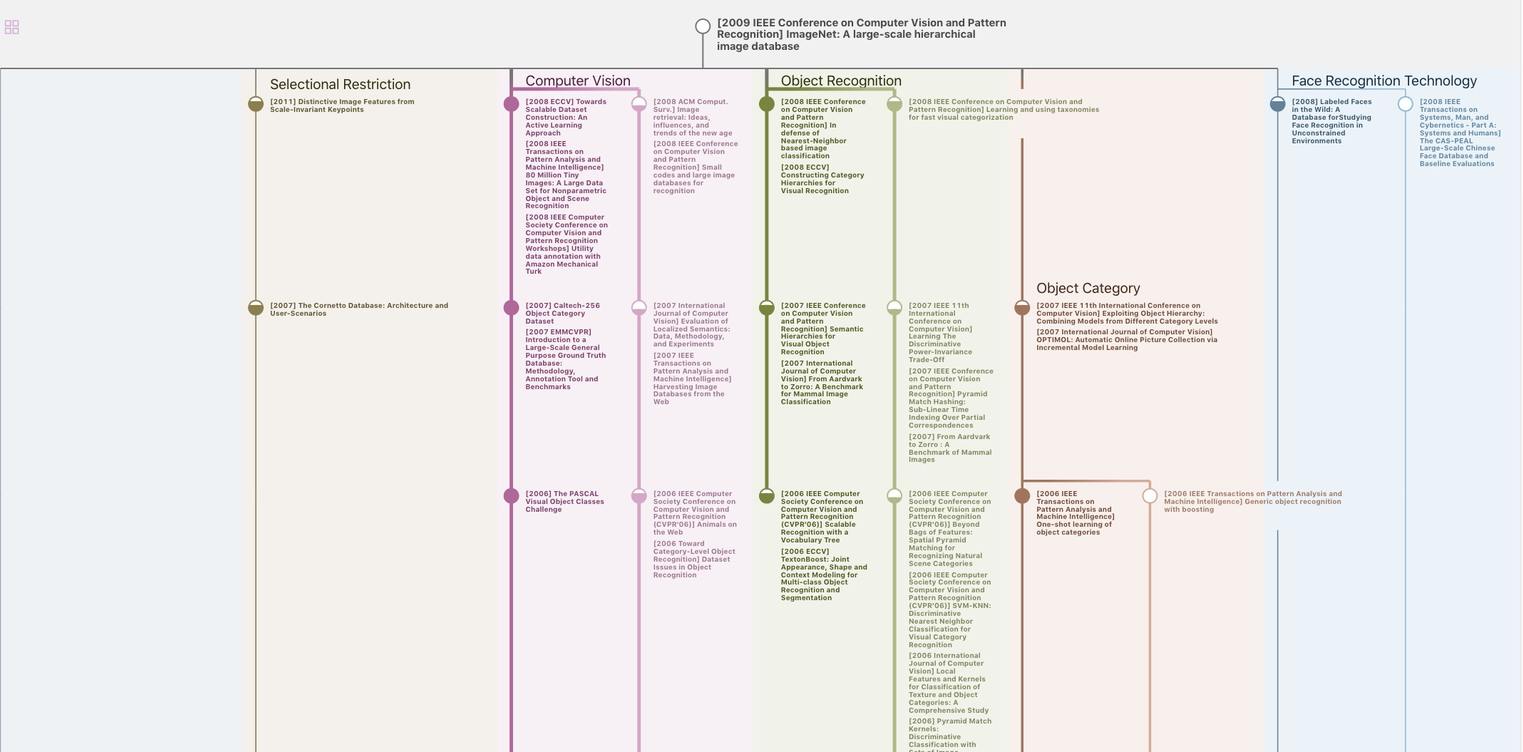
生成溯源树,研究论文发展脉络
Chat Paper
正在生成论文摘要