A novel reinforcement learning strategy for sequential detection of steady-state visual evoked potential-based bci
JOURNAL OF NONLINEAR AND CONVEX ANALYSIS(2023)
摘要
Steady-state visual evoked potential (SSVEP) had been widely ap-plied for brain computer interfaces (BCI) control. However, low-frequency stimu-lation might induce excessive visual discomfort and photosensitive epilepsy in sub-jects. In this paper, we proposed a novel self-adaptive algorithm combined rein -forcement learning strategy and sequential detection-based canonical correlation analysis (SDCCA) for SSVEP detection. On the independent dataset, our pro-posed method achieved a mean classification accuracy of 85.12% and information transmission rate of 8.86 bpm, which was 2.86%-9.17% and 0.95bpm-3.64bpm higher than those of state-of-the-art algorithms, respectively. It was validated that reinforcement learning strategy was more robust and applicable for sequen-tial detection of non-stationary time-series signals.
更多查看译文
关键词
SSVEP, reinforcement learning, sequential detection, canonical correlation analysis, BCI
AI 理解论文
溯源树
样例
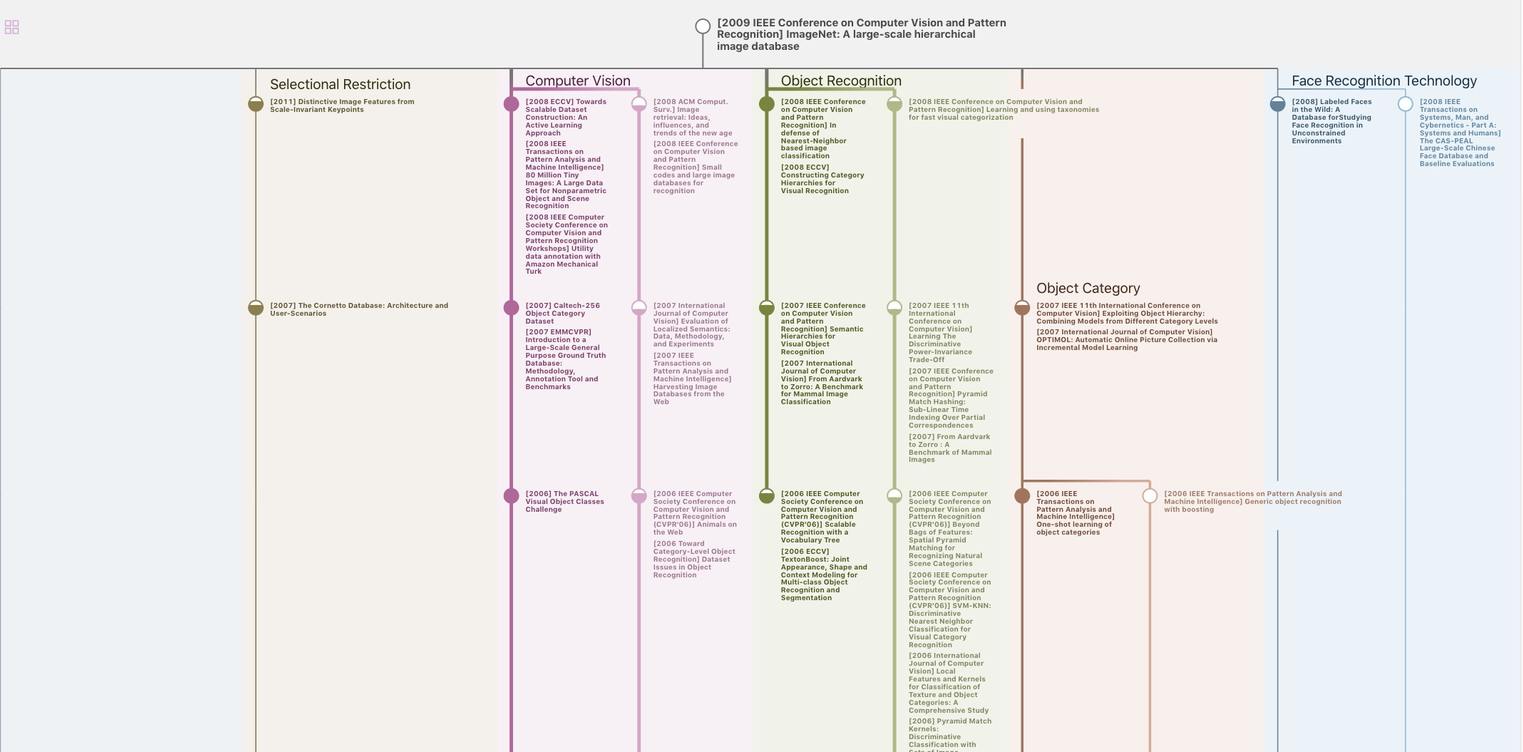
生成溯源树,研究论文发展脉络
Chat Paper
正在生成论文摘要