A partially inexact generalized primal-dual hybrid gradient method for saddle point problems with bilinear couplings
JOURNAL OF APPLIED MATHEMATICS AND COMPUTING(2023)
摘要
One of the most popular algorithms for saddle point problems is the so-named primal-dual hybrid gradient method, which have been received much considerable attention in the literature. Generally speaking, solving the primal and dual subproblems dominates the main computational cost of those primal-dual type methods. In this paper, we propose a partially inexact generalized primal-dual hybrid gradient method for saddle point problems with bilinear couplings, where the dual subproblem is solved approximately with a relative error strategy. Our proposed algorithm consists of two stages, where the first stage yields a predictor by solving the primal and dual subproblems, and the second procedure makes a correction on the predictor via a simple scheme. It is noteworthy that the underlying extrapolation parameter can be relaxed in a larger range, which allows us to have more choices than a fixed setting. Theoretically, we establish some convergence properties of the proposed algorithm, including the global convergence, the sub-linear convergence rate and the Q -linear convergence rate. Finally, some preliminary computational results demonstrate that our proposed algorithm works well on the fused Lasso problem with synthetic datasets and a pixel-constrained image restoration model.
更多查看译文
关键词
Saddle point problem,Primal-dual hybrid gradient algorithm,Prediction-Correction,Linear convergence,Error bound
AI 理解论文
溯源树
样例
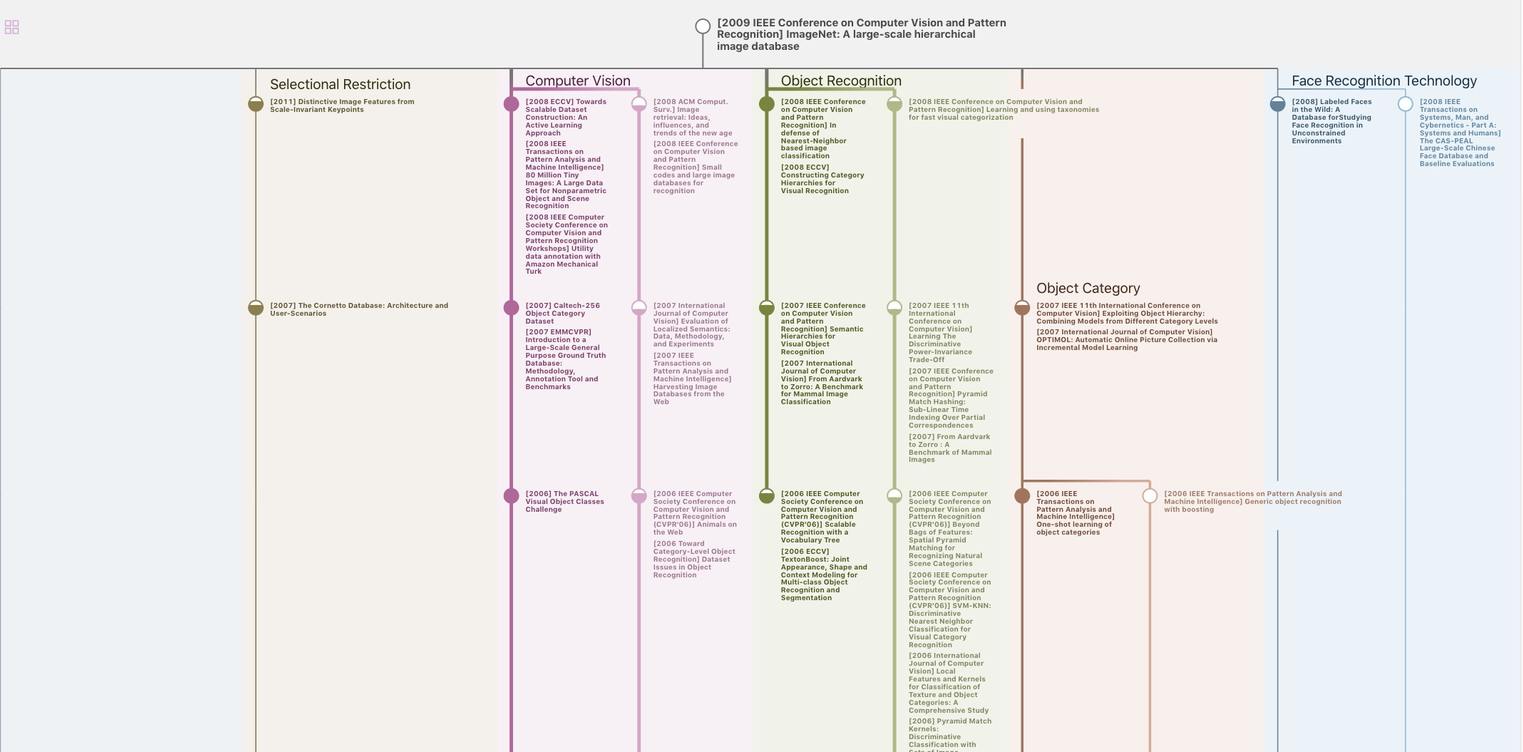
生成溯源树,研究论文发展脉络
Chat Paper
正在生成论文摘要