Transformer Architecture-Based Transfer Learning for Politeness Prediction in Conversation
SUSTAINABILITY(2023)
摘要
Politeness is an essential part of a conversation. Like verbal communication, politeness in textual conversation and social media posts is also stimulating. Therefore, the automatic detection of politeness is a significant and relevant problem. The existing literature generally employs classical machine learning-based models like naive Bayes and Support Vector-based trained models for politeness prediction. This paper exploits the state-of-the-art (SOTA) transformer architecture and transfer learning for respectability prediction. The proposed model employs the strengths of context-incorporating large language models, a feed-forward neural network, and an attention mechanism for representation learning of natural language requests. The trained representation is further classified using a softmax function into polite, impolite, and neutral classes. We evaluate the presented model employing two SOTA pre-trained large language models on two benchmark datasets. Our model outperformed the two SOTA and six baseline models, including two domain-specific transformer-based models using both the BERT and RoBERTa language models. The ablation investigation shows that the exclusion of the feed-forward layer displays the highest impact on the presented model. The analysis reveals the batch size and optimization algorithms as effective parameters affecting the model performance.
更多查看译文
关键词
politeness prediction,transfer learning,conversation,architecture-based
AI 理解论文
溯源树
样例
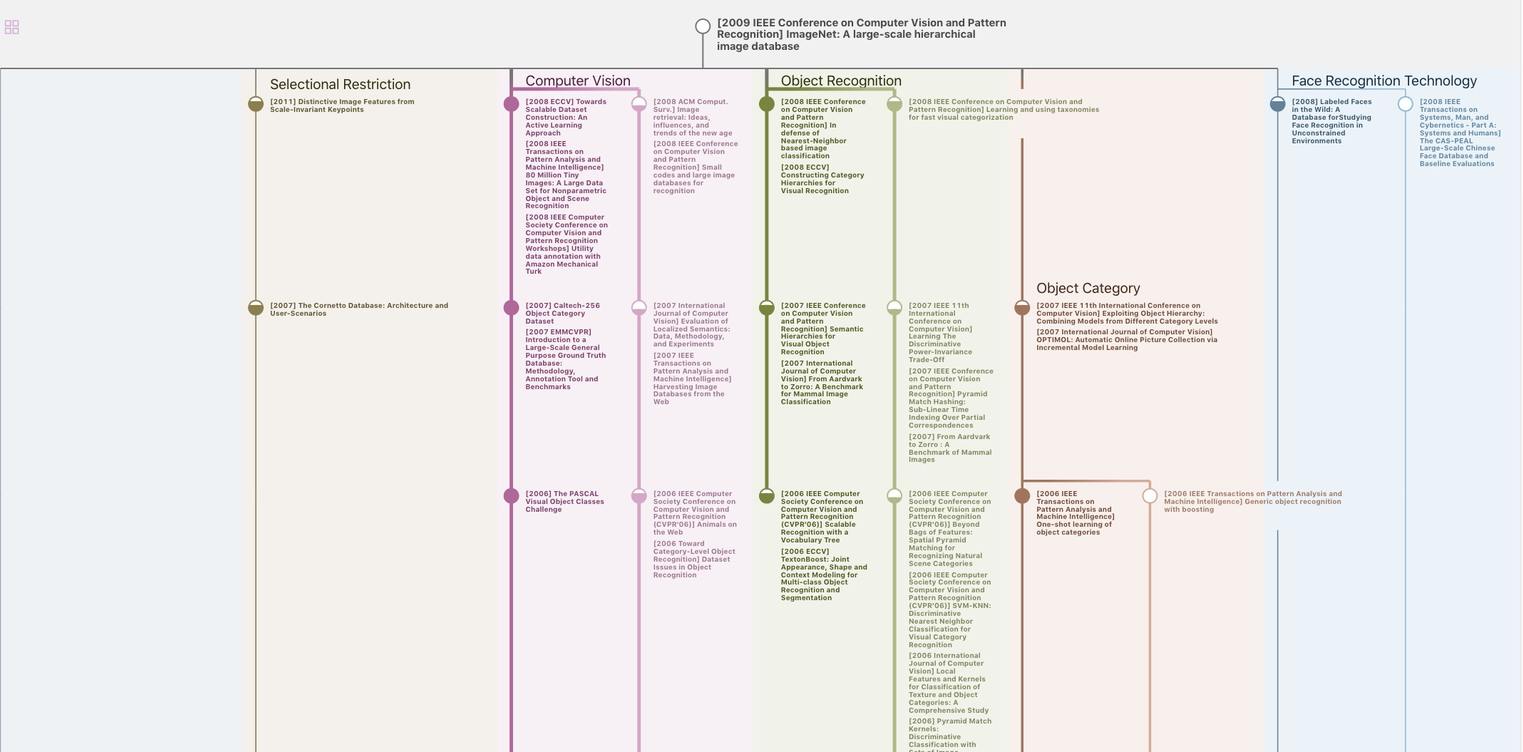
生成溯源树,研究论文发展脉络
Chat Paper
正在生成论文摘要