Deep Learning Peephole LSTM Neural Network-Based Channel State Estimators for OFDM 5G and Beyond Networks
MATHEMATICS(2023)
摘要
This study uses deep learning (DL) techniques for pilot-based channel estimation in orthogonal frequency division multiplexing (OFDM). Conventional channel estimators in pilot-symbol-aided OFDM systems suffer from performance degradation, especially in low signal-to-noise ratio (SNR) regions, due to noise amplification in the estimation process, intercarrier interference, a lack of primary channel data, and poor performance with few pilots, although they exhibit lower complexity and require implicit knowledge of the channel statistics. A new method for estimating channels using DL with peephole long short-term memory (peephole LSTM) is proposed. The proposed peephole LSTM-based channel state estimator is deployed online after offline training with generated datasets to track channel parameters, which enables robust recovery of transmitted data. A comparison is made between the proposed estimator and conventional LSTM and GRU-based channel state estimators using three different DL optimization techniques. Due to the outstanding learning and generalization properties of the DL-based peephole LSTM model, the suggested estimator significantly outperforms the conventional least square (LS) and minimum mean square error (MMSE) estimators, especially with a few pilots. The suggested estimator can be used without prior information on channel statistics. For this reason, it seems promising that the proposed estimator can be used to estimate the channel states of an OFDM communication system.
更多查看译文
关键词
channel state estimators,ofdm,5g,deep learning,network-based
AI 理解论文
溯源树
样例
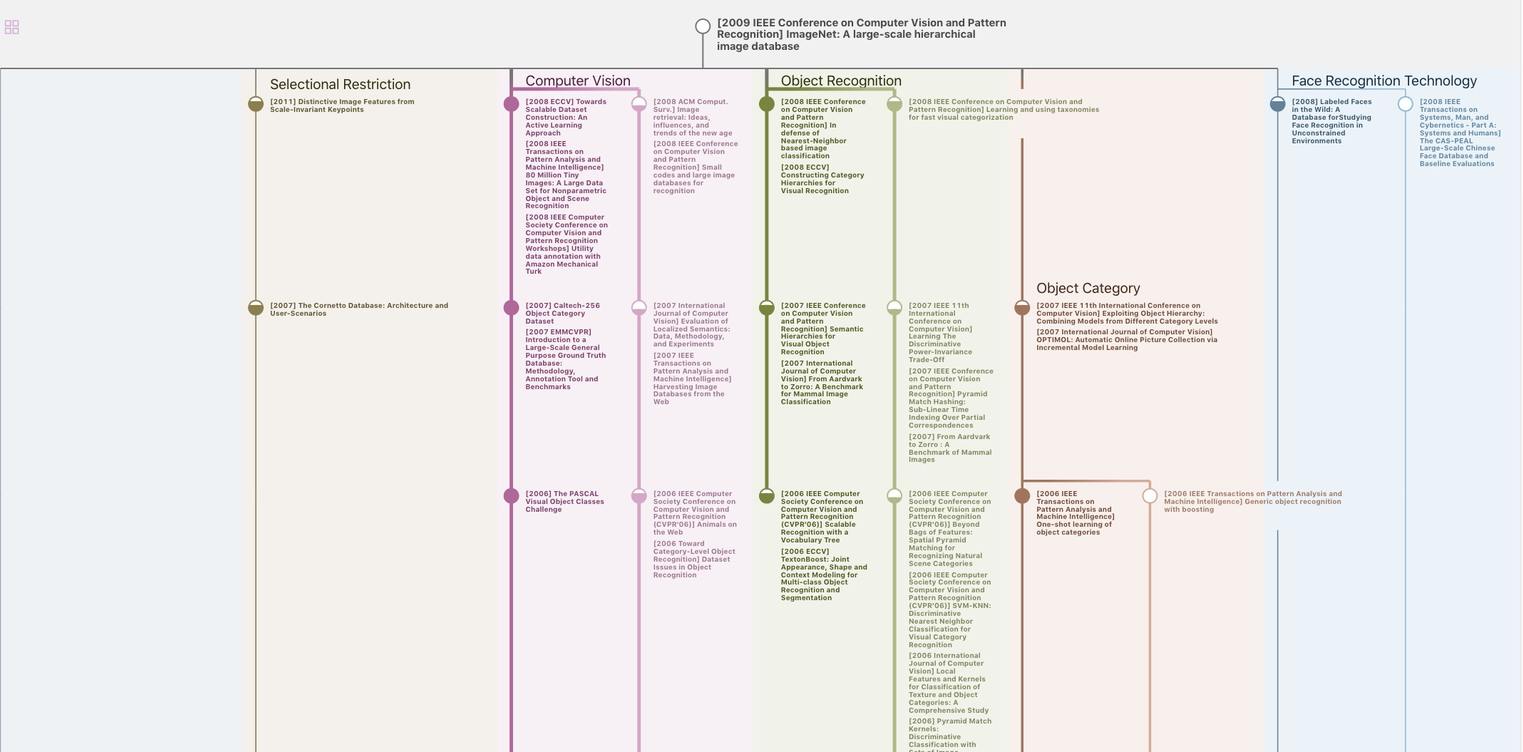
生成溯源树,研究论文发展脉络
Chat Paper
正在生成论文摘要