Dual-kernel echo state network for nonlinear time series prediction
PROCEEDINGS OF THE ROMANIAN ACADEMY SERIES A-MATHEMATICS PHYSICS TECHNICAL SCIENCES INFORMATION SCIENCE(2023)
摘要
An echo state network (ESN) is a recurrent neural network (RNN) often applied to nonlinear time series prediction. The traditional ESN randomly generates the weights of the input layer and the reservoir layer and does not change these weights, and generally only learns the weights of the output layer through linear regression, so the training speed is very fast. In this work, we propose a novel kernel echo state network (KESN). In KESN, the random weights of the input layer are removed and a series of gaussian kernels are used to replace the neurons in the input layer. Similar to radial basis function (RBF) neural networks, KESN can use the k-means algorithm to generate the kernel center and estimate the bandwidth of the kernel function. We prove that a KESN has echo state property, which is an important factor of KESN that can normally work. Furthermore, kernel ridge regression (KRR) is used to learn the weights of the output layer instead of a simple linear model. Finally, to obtain the optimal parameters of the model, the tree-structured parzen estimator approach (TPE) is used to optimize the hyperparameters of the model. In a time series prediction experiment, it is proved that KESN is more stable and performs better than the echo state network which randomly generates weights and trains output weights using linear models. We found that the reservoir layer weights are equivalent to a dropout operation, and the KESN is inherently equivalent to a regularized neural network. We call the KRR-based KESN dual-kernel echo state network (DKESN).
更多查看译文
关键词
recurrent neural network,Kernel echo state network,Kernel ridge regression,time series prediction,tree-structured Parzen estimator
AI 理解论文
溯源树
样例
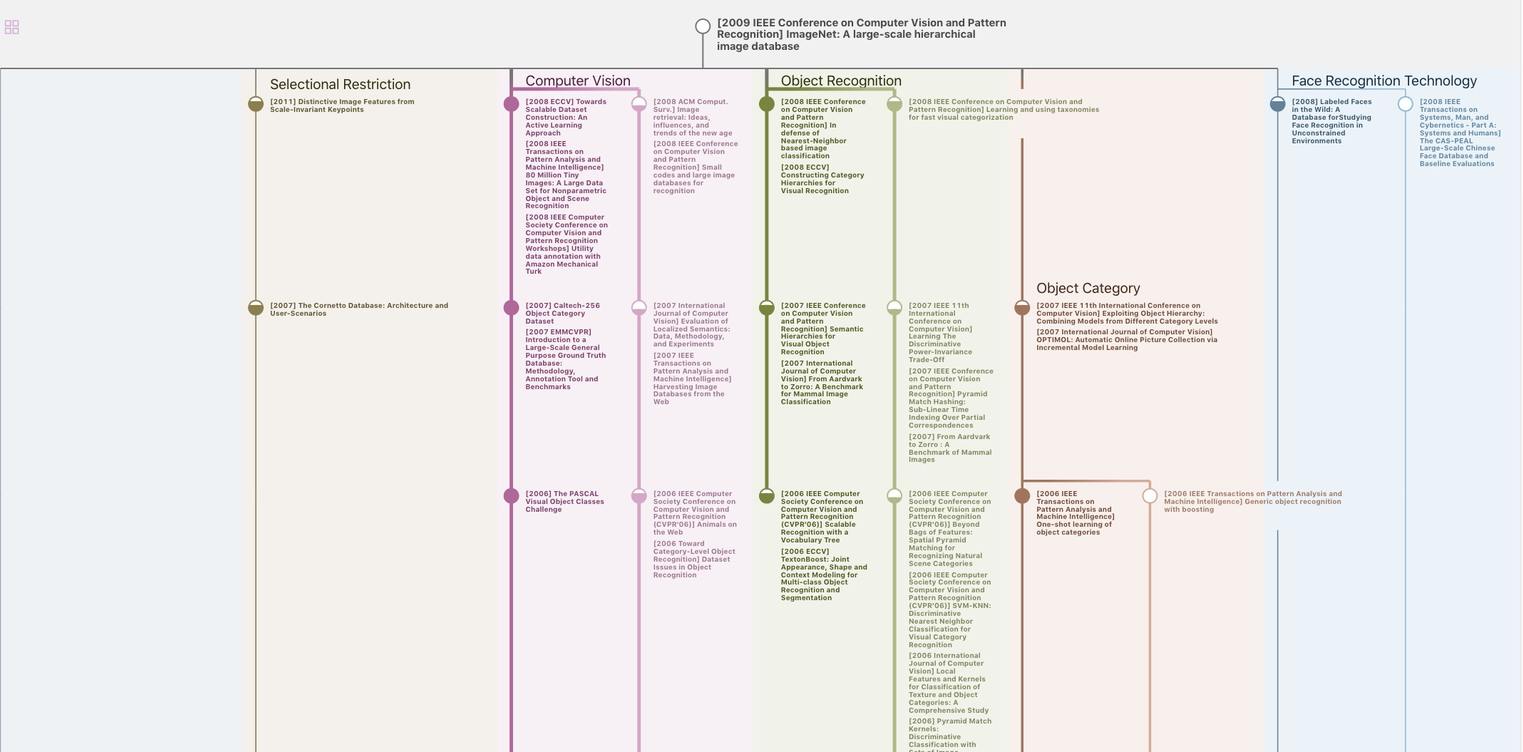
生成溯源树,研究论文发展脉络
Chat Paper
正在生成论文摘要