Optimizing Regression Models for Predicting Noise Pollution Caused by Road Traffic
SUSTAINABILITY(2023)
摘要
The study focuses on addressing the growing concern of noise pollution resulting from increased transportation. Effective strategies are necessary to mitigate the impact of noise pollution. The study utilizes noise regression models to estimate road-traffic-induced noise pollution. However, the availability and reliability of such models can be limited. To enhance the accuracy of predictions, optimization techniques are employed. A dataset encompassing various landscape configurations is generated, and three regression models (regression tree, support vector machines, and Gaussian process regression) are constructed for noise-pollution prediction. Optimization is performed by fine-tuning hyperparameters for each model. Performance measures such as mean square error (MSE), root mean square error (RMSE), and coefficient of determination (R-2) are utilized to determine the optimal hyperparameter values. The results demonstrate that the optimization process significantly improves the models' performance. The optimized Gaussian process regression model exhibits the highest prediction accuracy, with an MSE of 0.19, RMSE of 0.04, and R-2 reaching 1. However, this model is comparatively slower in terms of computation speed. The study provides valuable insights for developing effective solutions and action plans to mitigate the adverse effects of noise pollution.
更多查看译文
关键词
noise pollution,regression models
AI 理解论文
溯源树
样例
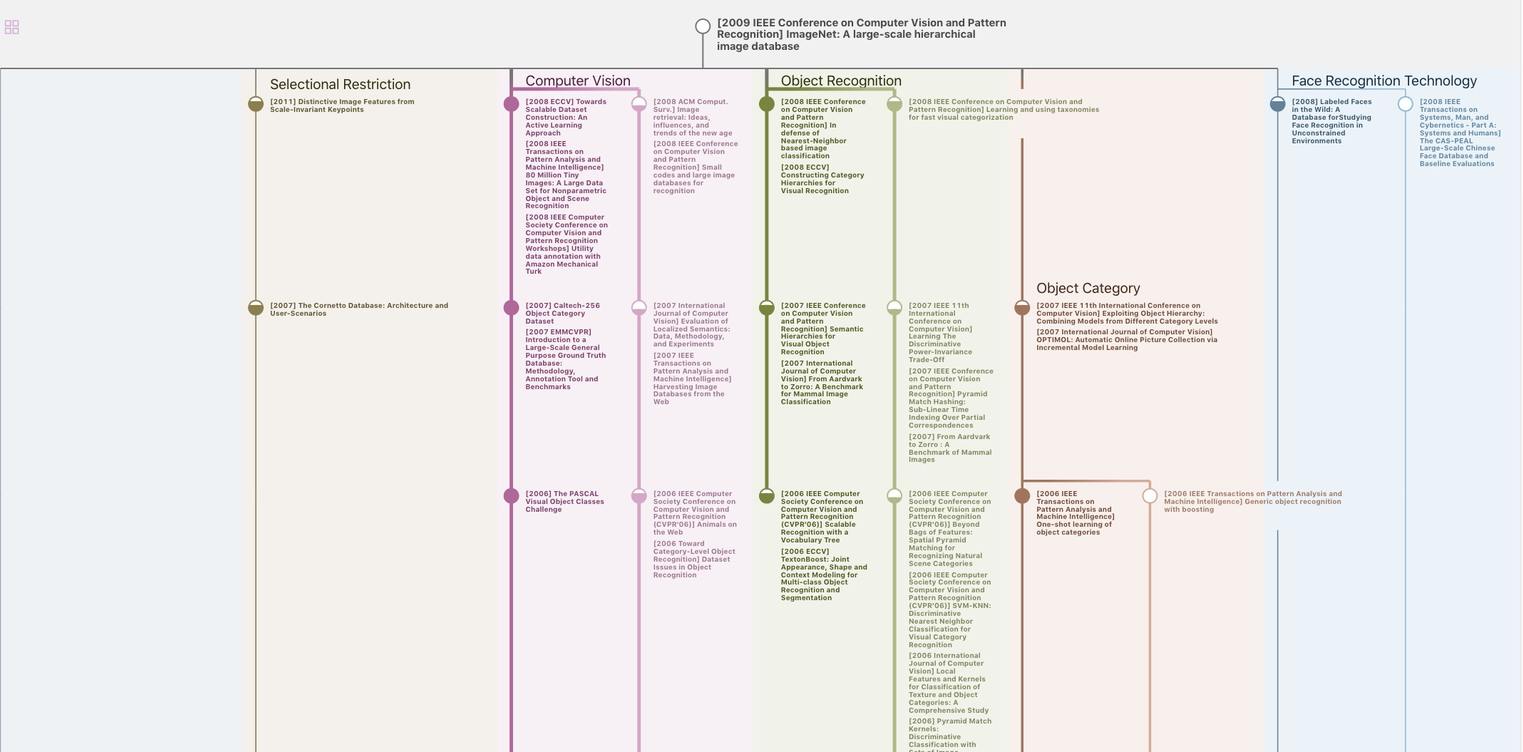
生成溯源树,研究论文发展脉络
Chat Paper
正在生成论文摘要