Multi-perspective contrastive learning framework guided by sememe knowledge and label information for sarcasm detection
INTERNATIONAL JOURNAL OF MACHINE LEARNING AND CYBERNETICS(2023)
摘要
Sarcasm is a prevailing rhetorical device that intentionally uses words that literally meaning opposite the real meaning. Due to this deliberate ambiguity, accurately detecting sarcasm can encourage the comprehension of users’ real intentions. Therefore, sarcasm detection is a critical and challenging task for sentiment analysis. In previous research, neural network-based models are generally unsatisfactory when dealing with complex sarcastic expressions. To ameliorate this situation, we propose a multi-perspective contrastive learning framework for sarcasm detection, called SLGC , which is guided by sememe knowledge and label information based on the pre-trained neural model. For the in-instance perspective, we leverage the sememe, the minimum meaning unit, to guide the contrastive learning to produce high-quality sentence representations. For the between-instance perspective, we utilize label information to guide contrastive learning to mine potential interaction relationships between sarcastic expressions. Experiments on two public benchmark sarcasm detection dataset demonstrate that our approach significantly outperforms the current state-of-the-art model.
更多查看译文
关键词
sememe knowledge,learning,multi-perspective
AI 理解论文
溯源树
样例
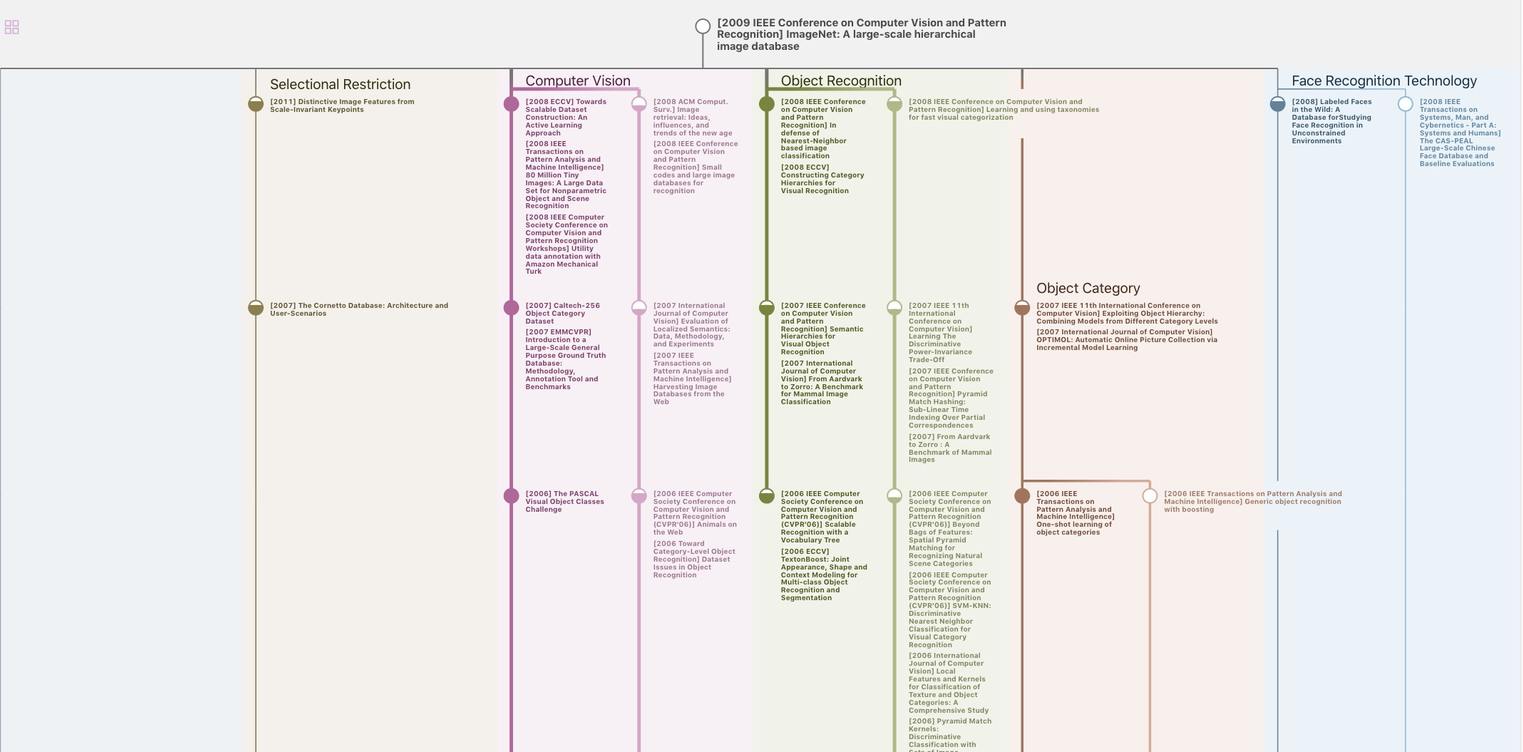
生成溯源树,研究论文发展脉络
Chat Paper
正在生成论文摘要