CFGN: A Lightweight Context Feature Guided Network for Image Super-Resolution
IEEE TRANSACTIONS ON EMERGING TOPICS IN COMPUTATIONAL INTELLIGENCE(2024)
摘要
Convolutional neural networks (CNNs) have recently been widely and successfully applied in the image computer vision community, and obtained great advances in single image super-resolution (SR). However, most of the existing SR methods focus on designing networks with deeper or wider structures for better performance and suffer from the problem of heavy computational costs. To address this problem, we propose a novel Context Feature Guided Network (CFGN), which is an efficient and effective lightweight SR method. Specifically, To capture semantic features effectively, we propose a novel block, called context feature guided convolution (CFGC), to capture more discriminative features while enlarging the receptive field. Moreover, we design a novel context feature guided group (CFGG) to exploit the multi-scale context information. Extensive experiments demonstrate the effectiveness of our method with a good trade-off between performance and computational efficiency, compared with the proposed method over the state-of-the-art lightweight SR methods.
更多查看译文
关键词
Image super resolution,convolutional neural networks,feature aggregation
AI 理解论文
溯源树
样例
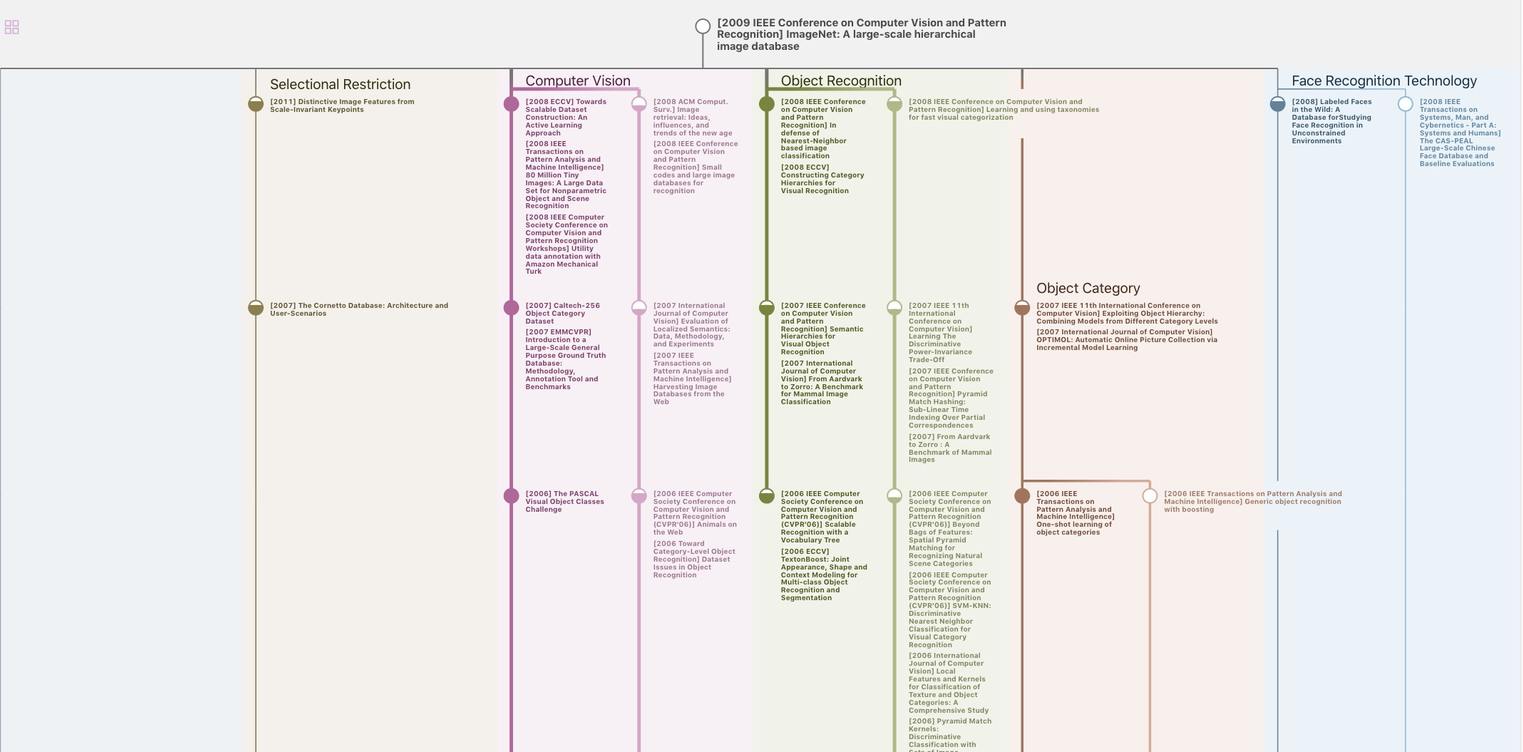
生成溯源树,研究论文发展脉络
Chat Paper
正在生成论文摘要