Multivariate statistical algorithms for landslide susceptibility assessment in Kailash Sacred landscape, Western Himalaya
GEOMATICS NATURAL HAZARDS & RISK(2023)
摘要
Landslide susceptibility mapping plays an imperative role in mitigating hazards and determining the future direction of developmental activities in mountainous regions. Here, we used 518 landslide occurrences and nine landslide-conditioning parameters to build landslide vulnerability models in the Kailash Sacred Landscape (KSL), India. Four multivariate statistical models were applied, namely the generalized linear model (GLM), maximum entropy (MaxEnt), Mahalanobis D-2 (MD), and support vector machine (SVM), to calibrate and compare four maps of landslide susceptibility. The results demonstrated the outperformance of Mahalanobis D-2 for predictability compared to other models obtained from the area under the receiver operating characteristic curve (ROC). The ensemble model data shows that 10.5% of the landscape has susceptible conditions for future landslides, whereas 89.50% of the landscape falls under the safe zone. The occurrence of landslides in the KSL is linked to the middle elevations, vicinity to water bodies, and the motorable roads. Furthermore, the observed patterns and the resulting models exhibit the major variables that cause landslides and their respective significance. The current modelling approach could provide baseline data at the regional scale to improve the developmental planning in the KSL.
更多查看译文
关键词
Landslide susceptibility modelling, landslide conditioning, landscape vulnerability, Boyce Index, risk assessment, Himalaya
AI 理解论文
溯源树
样例
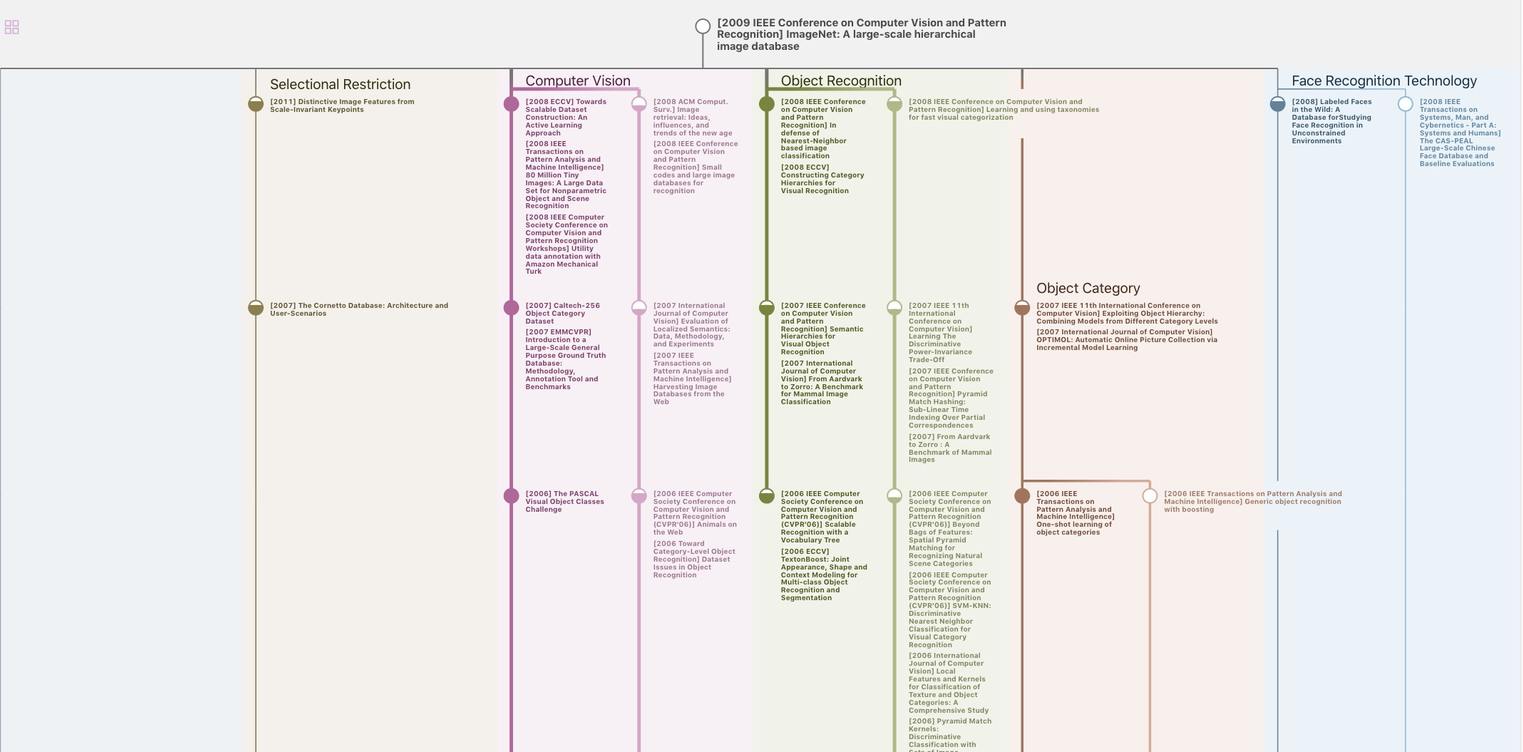
生成溯源树,研究论文发展脉络
Chat Paper
正在生成论文摘要