A two-stage framework for credit scoring based on feature augmentation and dimension reduction
COMMUNICATIONS IN STATISTICS-SIMULATION AND COMPUTATION(2023)
摘要
Credit scoring is an important area for financial risk management, where a small improvement in prediction performance will save significant losses for financial institutions. This article proposes a two-stage framework for credit scoring based on feature augmentation and dimension reduction to improve the model performance. In this framework, the logarithm marginal density ratios transformation is employed to provide more prominent features for credit scoring, fully utilizing original data information. For the dimension reduction process, we apply three classifiers for L-1 penalized logistic regression (add a sum of the parameter absolute value to the criterion function), extreme gradient boosting with L-1 regularization term, and k-nearest neighbor with sequential forward selection to two different credit data sets. The results indicate that this two-stage framework can improve the performance of credit scoring models.
更多查看译文
关键词
Credit scoring, Dimension reduction, Feature augmentation, Two stage framework
AI 理解论文
溯源树
样例
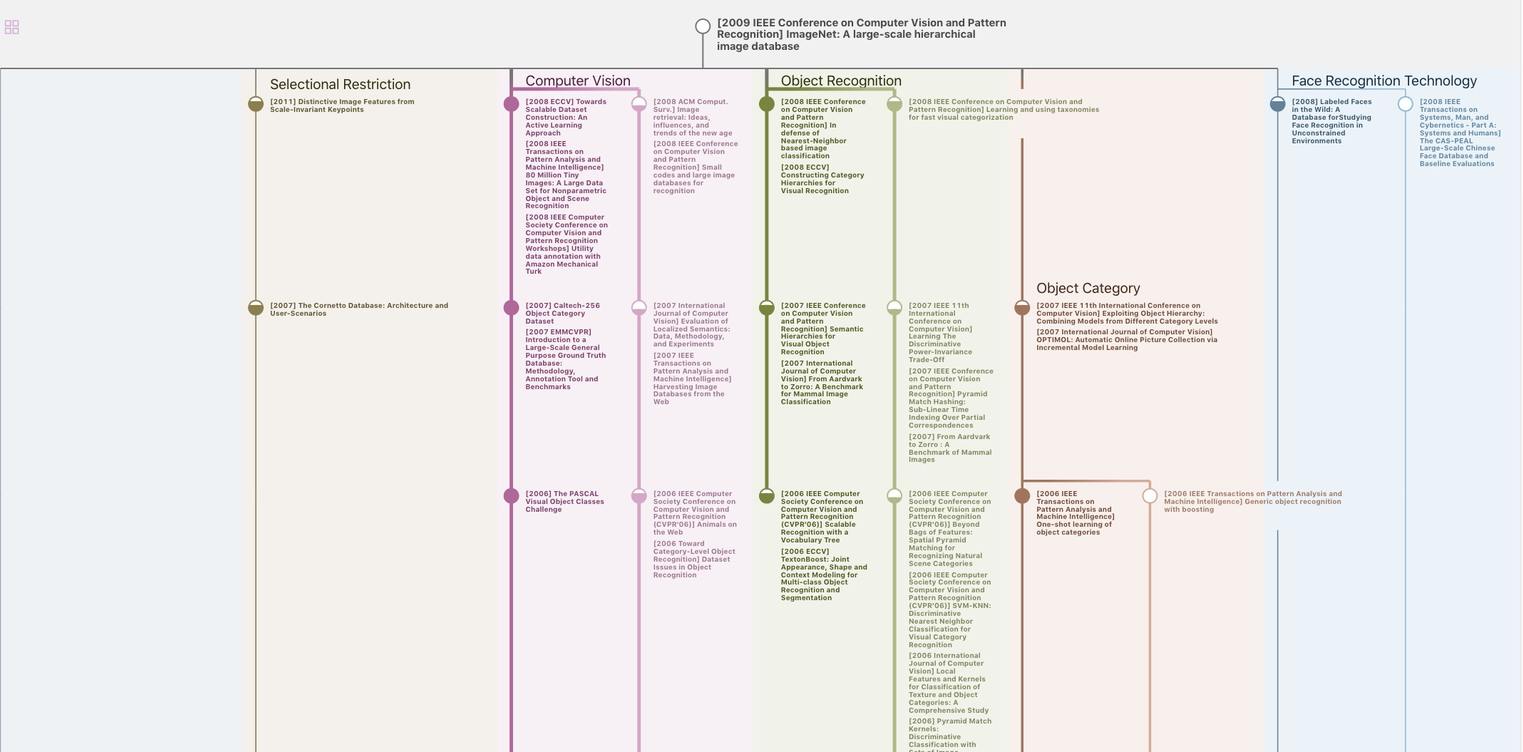
生成溯源树,研究论文发展脉络
Chat Paper
正在生成论文摘要