Predicting the length-of-stay of pediatric patients using machine learning algorithms
INTERNATIONAL JOURNAL OF PRODUCTION RESEARCH(2023)
摘要
The management of hospitals' resource capacity has a strong impact on the quality of care, and the length-of-stay (LOS) of patients is an indicator that reflects its efficiency and effectiveness. This study aims at predicting the LOS of pediatric patients (LOS-P) in hospitals to assist in decision-making regarding resource utilisation. LOS-P forecasting presents additional challenges to the analyst compared to other medical specialties since Pediatrics comprises several other subspecialties (e.g. pediatric oncology and traumatology). Pediatric patients within subspecialties compete for the same hospital resources, and aggregate LOS-P predictions are more useful for resource planning. However, aggregate pediatric LOS datasets are harder to model and result in lower forecasting accuracy. To address that problem, we propose a forecasting model based on Machine Learning algorithms. The method for LOS-P forecasting comprises five steps (data visualisation, data pre-processing, sample partitioning, model testing, and model definition through parameter setting and variable selection) and is tested using a dataset of hospitalisations of pediatric patients from a large Brazilian University hospital. Multiple linear regression, random forest, support vector regression, ridge regression, and partial least squares algorithms are applied and compared to determine the best forecasting model. Results indicate that all forecasting models yield satisfactory accuracy, with the best algorithms being random forest and support vector regressor. After refining the model through variable selection and using a Grid Search to find the best parameters, the random forest algorithm yielded an R-2 of 65.67%, with an average absolute error of 3.51 days.
更多查看译文
关键词
machine learning algorithms,machine learning,pediatric patients,length-of-stay
AI 理解论文
溯源树
样例
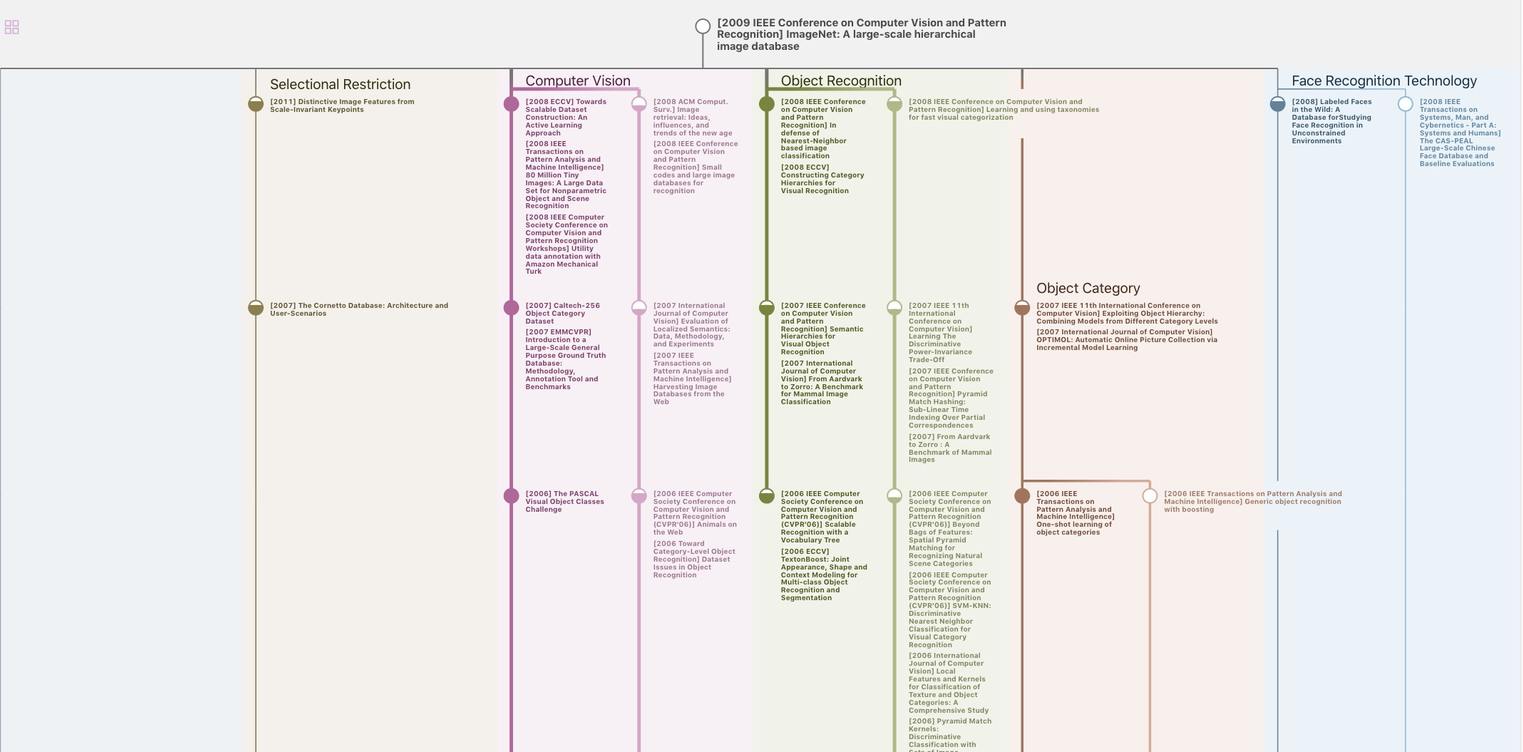
生成溯源树,研究论文发展脉络
Chat Paper
正在生成论文摘要