Multiclass Transportation Safety Hardware Asset Detection and Segmentation Based on Mask-RCNN with RoI Attention and IoMA-Merging
JOURNAL OF COMPUTING IN CIVIL ENGINEERING(2023)
摘要
Transportation assets, including retaining walls, noise barriers, rumble strips, guardrails, guardrail anchors, and central cable barriers, are important roadside safety hardware and geotechnical structures. They need to be inventoried accurately to support asset management and ensure roadway safety. There are detection methods for some of these transportation assets, which however lack flexibility and multiclass abilities. Moreover, the potential of neural networks has not been fully utilized although some detection methods have used deep learning. This paper for the first time proposes a multiclass transportation asset detection and pixel-wise segmentation model on two-dimensional images, based on region-based convolutional neural network (Mask-RCNN) with a feature pyramid network (FPN). The scale diversity and intensive continual appearance of transportation assets are identified as the main challenges, tending to result in numerous false-positive detections. A methodology with self-attention mechanisms based on the generic region of interest extractor (GRoIE) model along with the intersection over the minimum area merging (IoMA-Merging) postprocessing algorithm was then proposed. The evaluation outcomes demonstrated that our proposed methodology, including GRoIE-global context (GRoIE-GC) with IoMA-Merging achieves the best performance with a significant improvement over baseline. The precision increased by 10.0% on detection and 10.7% on segmentation. This proposed methodology will consequently improve the accuracy of asset inventory.
更多查看译文
关键词
segmentation,roi attention,detection,mask-rcnn,ioma-merging
AI 理解论文
溯源树
样例
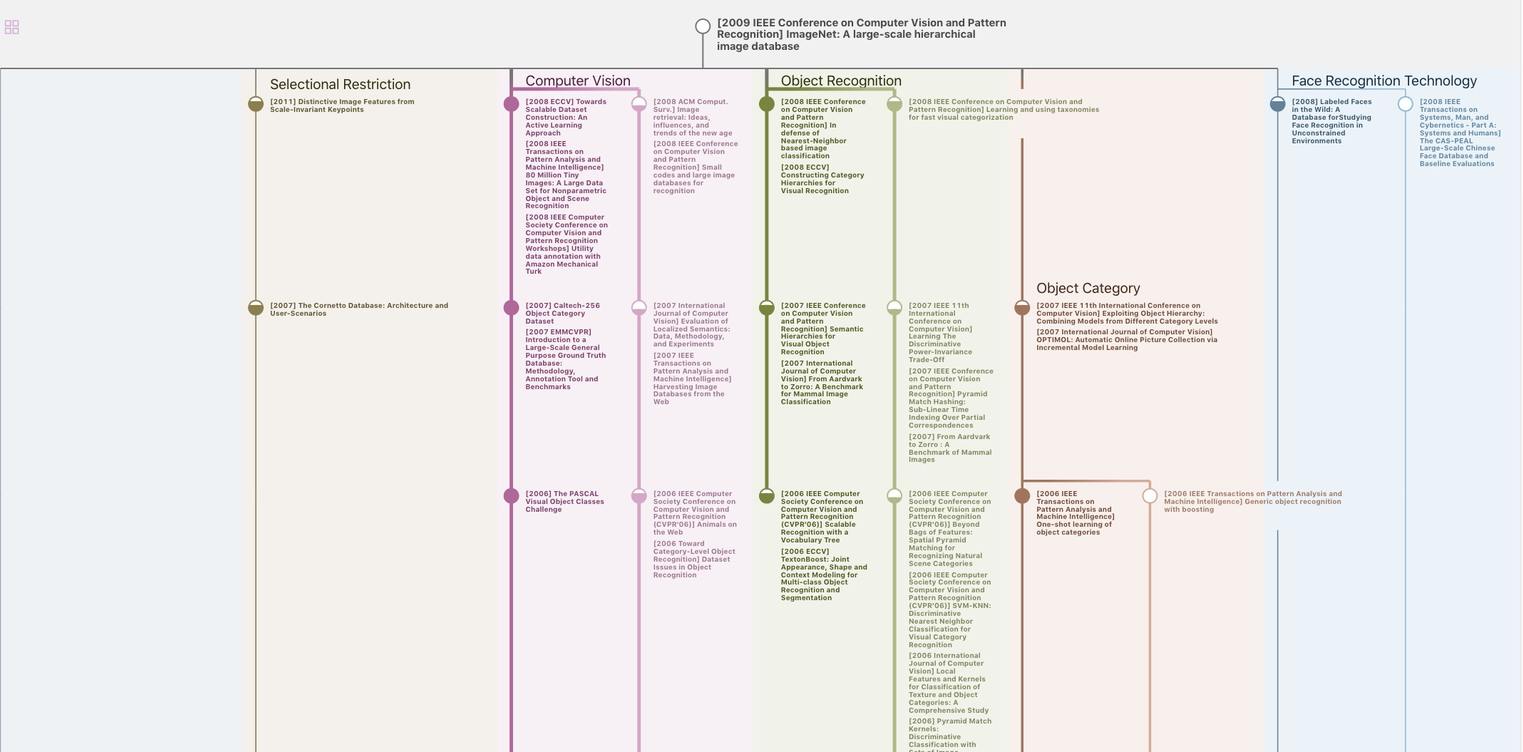
生成溯源树,研究论文发展脉络
Chat Paper
正在生成论文摘要