A Physics-Informed Generative Car-Following Model for Connected Autonomous Vehicles
ENTROPY(2023)
摘要
This paper proposes a novel hybrid car-following model: the physics-informed conditional generative adversarial network (PICGAN), designed to enhance multi-step car-following modeling in mixed traffic flow scenarios. This hybrid model leverages the strengths of both physics-based and deep-learning-based models. By taking advantage of the inherent structure of GAN, the PICGAN eliminates the need for an explicit weighting parameter typically used in the combination of traditional physics-based and data-driven models. The effectiveness of the proposed model is substantiated through case studies using the NGSIM I-80 dataset. These studies demonstrate the model's superior trajectory reproduction, suggesting its potential as a strong contender to replace conventional models in trajectory prediction tasks. Furthermore, the deployment of PICGAN significantly enhances the stability and efficiency in mixed traffic flow environments. Given its reliable and stable results, the PICGAN framework contributes substantially to the development of efficient longitudinal control strategies for connected autonomous vehicles (CAVs) in real-world mixed traffic conditions.
更多查看译文
关键词
autonomous,vehicles,model,physics-informed,car-following
AI 理解论文
溯源树
样例
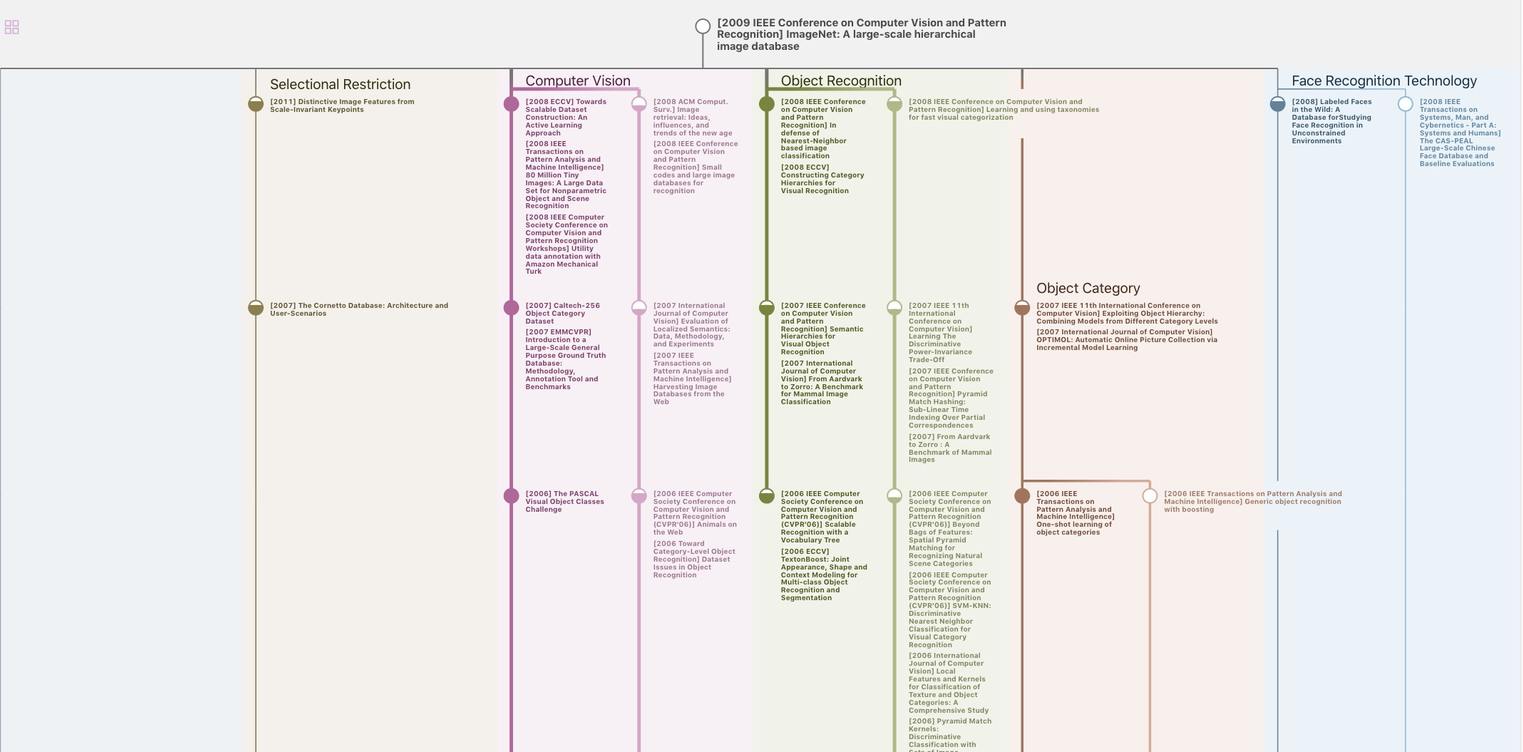
生成溯源树,研究论文发展脉络
Chat Paper
正在生成论文摘要