Hierarchical Reinforcement Learning Integrating With Human Knowledge for Practical Robot Skill Learning in Complex Multi-Stage Manipulation
IEEE TRANSACTIONS ON AUTOMATION SCIENCE AND ENGINEERING(2023)
摘要
This paper proposes a novel hierarchical reinforcement learning (HRL) framework of complex manipulation tasks which integrates the human prior knowledge. The framework involves the following steps: $ \textbf{1)} $ The manipulation process is divided into several stages based on human prior knowledge. $ \textbf{2)} $ Transition conditions between stages are determined in the form of "if-then" rules. $ \textbf{3)} $ The key features of each stage are selected, and the corresponding control policies are designed via human knowledge. $ \textbf{4)} $ The policy gradient with parameter-based exploration (PGPE) method is employed to optimize the policy parameters because it does not require the policy to be derivable for the parameters. To increase the convergence speed of this framework, importance sampling and adaptive adjustment of exploration variance are employed to improve it. $ \textbf{5)} $ On this basis, to facilitate the transfer of simulation results to practical experiments, half sim-to-real method is presented, which fully utilizes the simulation results, and the differences between simulation and experimental environments are considered. Simulation and experimental studies show that our framework can deal with the peg-hole-insertion task with a high quality in less than 1600 episodes and can safely adapt the skill into the practical scene with little iterations, which verify the efficiency of the presented method. Note to Practitioners-Intelligent robots will become the right assistants of human beings in the future, especially in various areas of complex manipulation occasions. The important premise is that the robots should have certain ability of complex manipulation skill learning. Complex manipulation tasks can be decomposed into multiple stages, and HRL is a suitable and efficient method for solving this kind of problems. This paper proposes a novel HRL framework which can better integrate the human prior knowledge. In addition, improved PGPE method is proposed to obtain the optimized policy parameters more quickly. More importantly, a novel half sim-to-real transfer method is presented to better integrate the simulation and experiment results. This provides a common paradigm, which leverages the simulation results to reduce the interaction between robot and practical environment and then utilizes the experiment results to further optimize the policy parameters.
更多查看译文
关键词
Hierarchical reinforcement learning,improved PGPE,human knowledge,robot manipulation skill learning,half sim-to-real
AI 理解论文
溯源树
样例
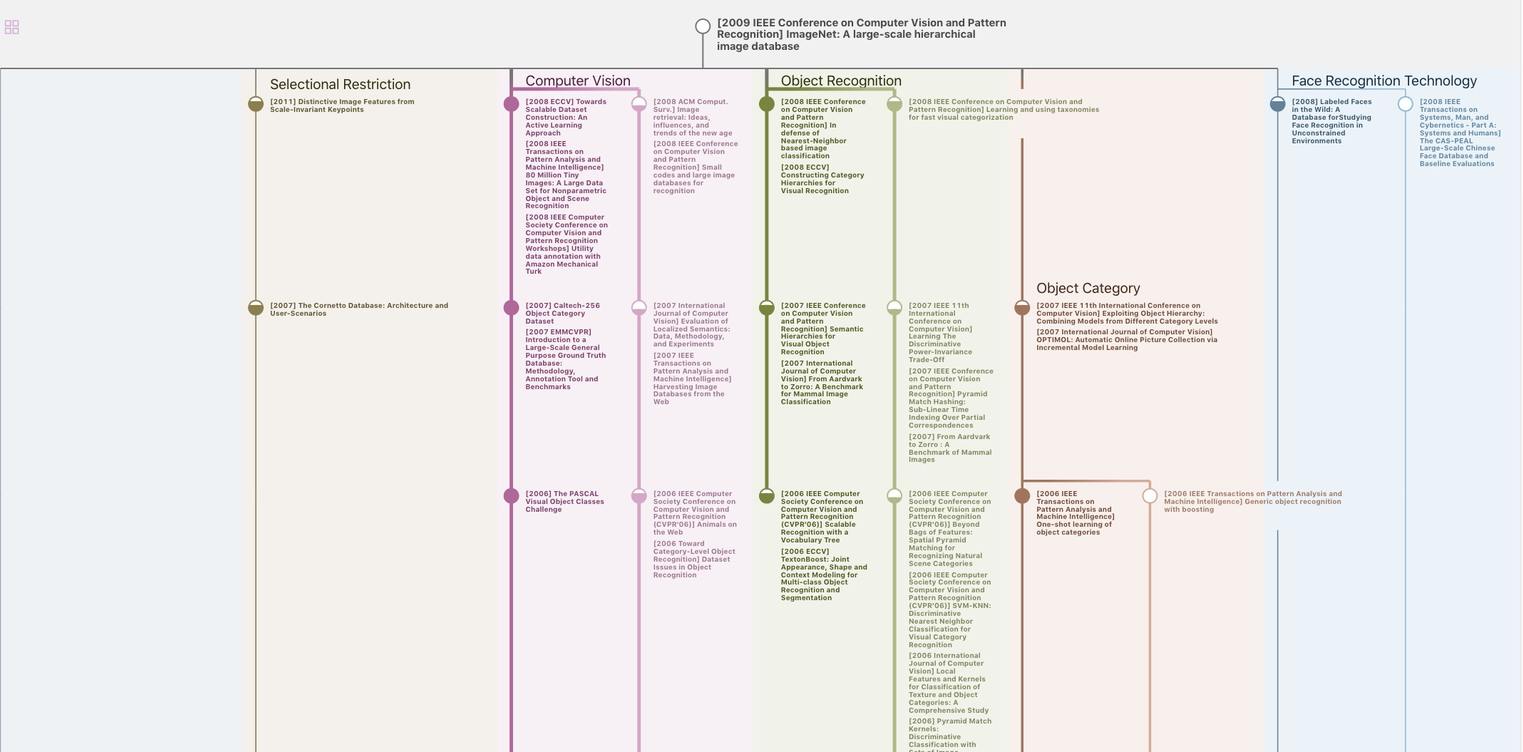
生成溯源树,研究论文发展脉络
Chat Paper
正在生成论文摘要