Deep Reinforcement Meta-Learning and Self-Organization in Complex Systems: Applications to Traffic Signal Control
ENTROPY(2023)
摘要
We studied the ability of deep reinforcement learning and self-organizing approaches to adapt to dynamic complex systems, using the applied example of traffic signal control in a simulated urban environment. We highlight the general limitations of deep learning for control in complex systems, even when employing state-of-the-art meta-learning methods, and contrast it with self-organization-based methods. Accordingly, we argue that complex systems are a good and challenging study environment for developing and improving meta-learning approaches. At the same time, we point to the importance of baselines to which meta-learning methods can be compared and present a self-organizing analytic traffic signal control that outperforms state-of-the-art meta-learning in some scenarios. We also show that meta-learning methods outperform classical learning methods in our simulated environment (around 1.5-2x improvement, in most scenarios). Our conclusions are that, in order to develop effective meta-learning methods that are able to adapt to a variety of conditions, it is necessary to test them in demanding, complex settings (such as, for example, urban traffic control) and compare them against established methods.
更多查看译文
关键词
complex systems,control,meta-learning,self-organization
AI 理解论文
溯源树
样例
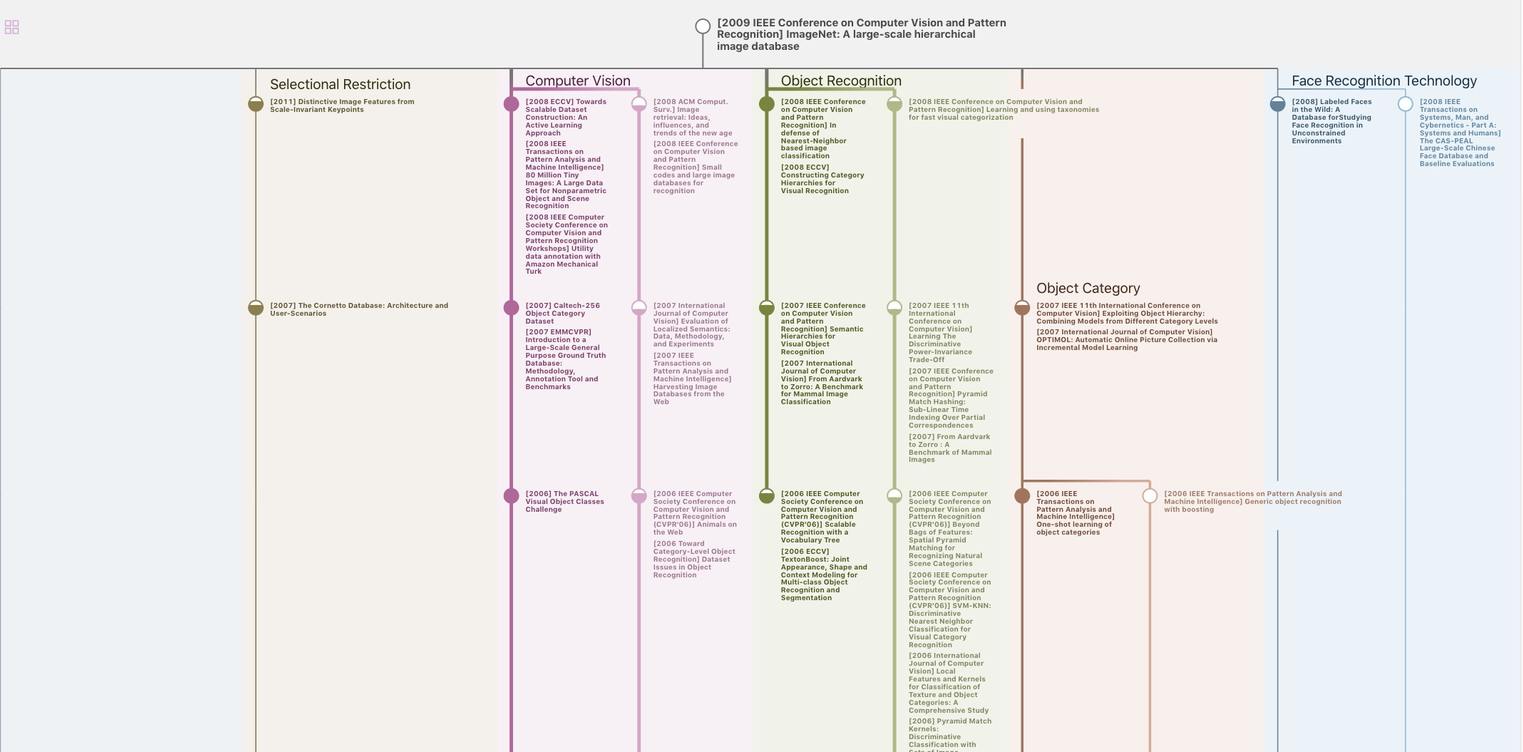
生成溯源树,研究论文发展脉络
Chat Paper
正在生成论文摘要