Remaining useful life distribution prediction framework for lithium-ion battery fused prior knowledge and monitoring data
MEASUREMENT SCIENCE AND TECHNOLOGY(2023)
摘要
Remaining useful life (RUL) prediction is the main approach to guarantee the reliability of lithium-ion batteries. This paper proposes an interpretable hybrid method to predict the RUL distribution with changeable form. The method integrates prior knowledge from the statistical model and regular patterns learned from monitoring data based on the data-driven model. The predicted compound distribution provides more information compared to point estimation and distribution with fixed form. The general hybrid framework contains a component learner, a fusion model with a stacking strategy, and a prognostic distribution algorithm with adaptive sampling weights. The stacking fusion model is implemented by a one-dimensional convolution neural network. The sampling weights are estimated by optimal estimation. The statistical model describes the individual capacity degradation path based on the Wiener process. The data-driven model learns the degradation process from historical data based on convolutional gated recurrent neural network (CNN-GRU) and Monte Carlo dropout simulation. The comparative experiments between the proposed method and existing methods were carried out. The experiment results show that the proposed hybrid method performs well.
更多查看译文
关键词
prior knowledge,battery,lithium-ion
AI 理解论文
溯源树
样例
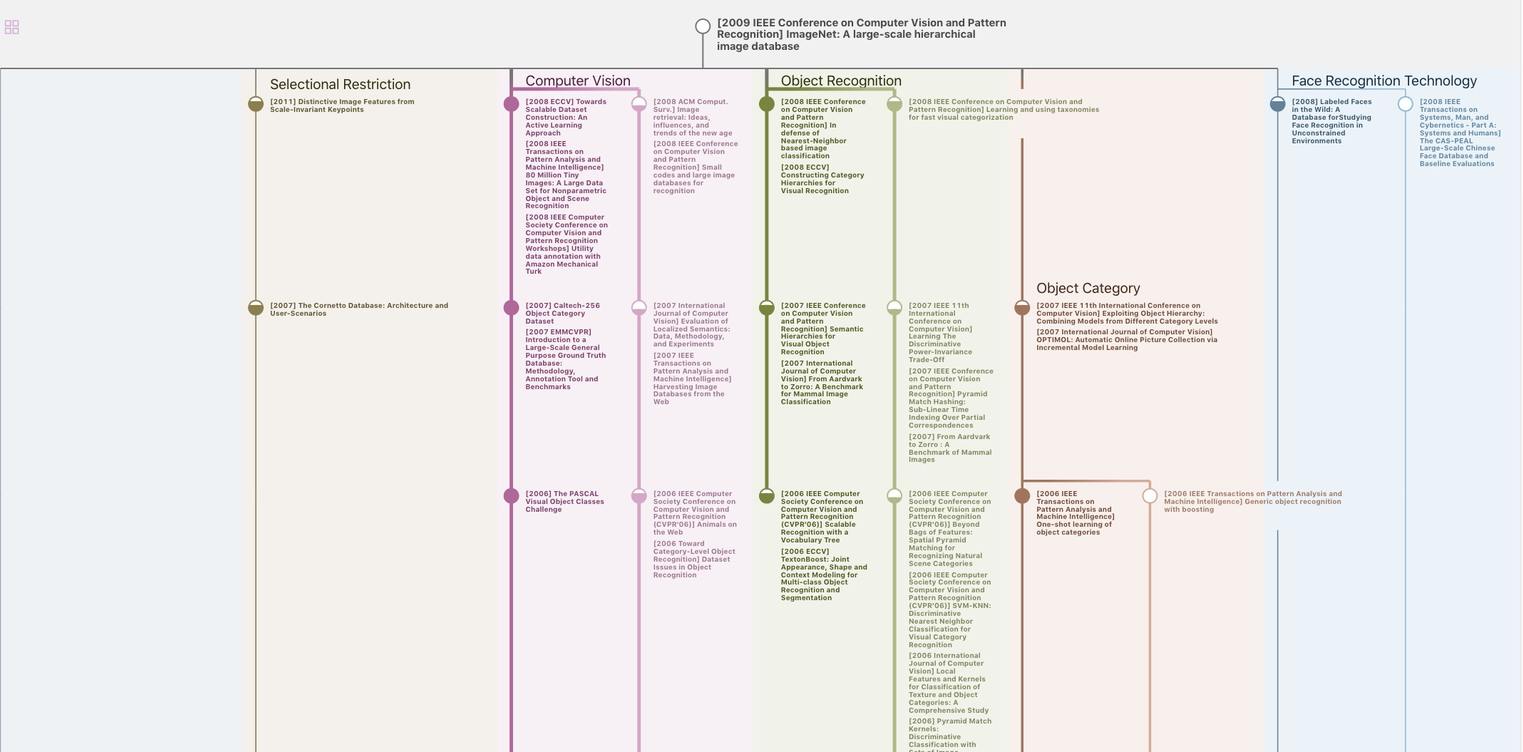
生成溯源树,研究论文发展脉络
Chat Paper
正在生成论文摘要