3D facial attractiveness prediction based on deep feature fusion
COMPUTER ANIMATION AND VIRTUAL WORLDS(2024)
摘要
Facial attractiveness prediction is an important research topic in the computer vision community. It not only contributes to the development of interdisciplinary research in psychology and sociology, but also provides fundamental technical support for applications like aesthetic medicine and social media. With the advances in 3D data acquisition and feature representation, this paper aims to investigate the facial attractiveness from deep learning and three-dimensional perspectives. The 3D faces are first processed to unwrap the texture images and refine the raw meshes. The feature extraction networks for texture, point cloud, and mesh are then delicately designed, considering the characteristics of different types of data. A more discriminative face representation is derived by feature fusion for the final attractiveness prediction. During network training, the cyclical learning rate with an improved range test is introduced, so as to alleviate the difficulty in hyperparameter setting. Extensive experiments are conducted on a 3D FAP benchmark, where the results demonstrate the significance of deep feature fusion and enhanced learning rate in cooperatively facilitating the performance. Specifically, the fusion of texture image and point cloud achieves the best overall prediction, with PC, MAE, and RMSE of 0.7908, 0.4153, and 0.5231, respectively.
更多查看译文
关键词
facial attractiveness prediction,3D face,deep feature learning,feature fusion,cyclical learning rate
AI 理解论文
溯源树
样例
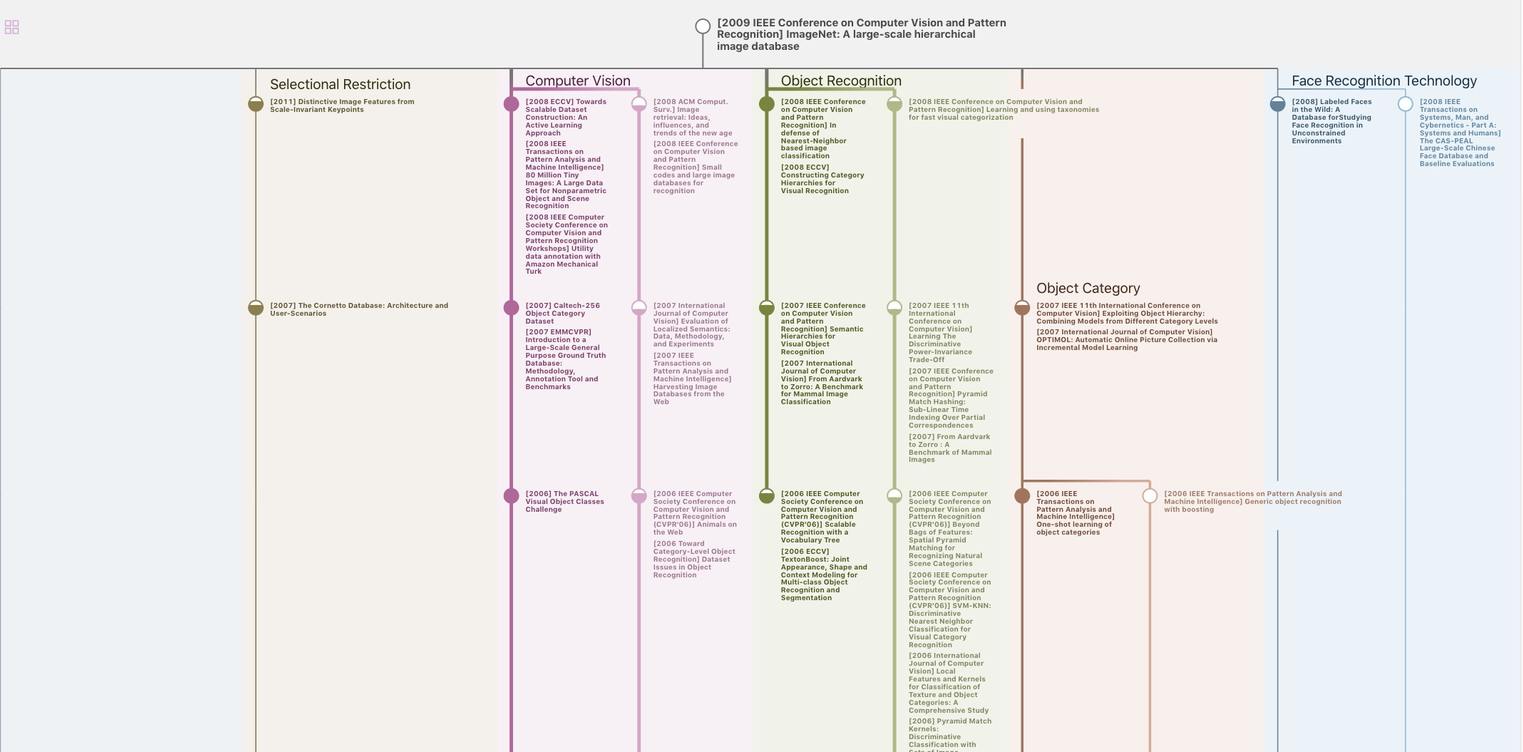
生成溯源树,研究论文发展脉络
Chat Paper
正在生成论文摘要