Parkinson's disease diagnosis using recurrent neural network based deep learning model by analyzing online handwriting
MULTIMEDIA TOOLS AND APPLICATIONS(2024)
摘要
Parkinson's disease is an escalating neurodegenerative disorder that adversely affects movement, muscle flexibility, speech, and writing skills. Early diagnosis can reduce its severity as well as the expenditure incurred for the treatment. Although several works exist using machine learning techniques to detect parkinson's disease, very few have focused to detect this disease by analyzing online handwritten tasks. This article proposes bi-directional long short-term memory [a variant of recurrent neural network (RNN)] based method to develop a parkinson's disease diagnosis system by analyzing online handwritten tasks. The aim of this work is twofold: (a) to find out the best task/tasks capable to discriminate between PD patient and healthy person and (b) to develop a robust method to detect the PD patient. Various kinematics features have been extracted from different handwritten tasks and the extracted features have been studied by three different machine learning techniques named support vector machine (SVM), adaboost classifier, and bagged random forest (BRF) as well as two different variants of deep learning model RNN known as long short-term memory (LSTM) and bi-directional long short-term memory (BLSTM). The parkinson's disease classification performance of the proposed method has been evaluated on a very popular publicly available dataset parkinsion disease handwriting database (PaHaW). The experimental results show that the proposed method outperforms the state-of-the-art methods in this regard.
更多查看译文
关键词
Parkinson's disease,Kinematics features,Deep learning,Recurrent neural network,BLSTM
AI 理解论文
溯源树
样例
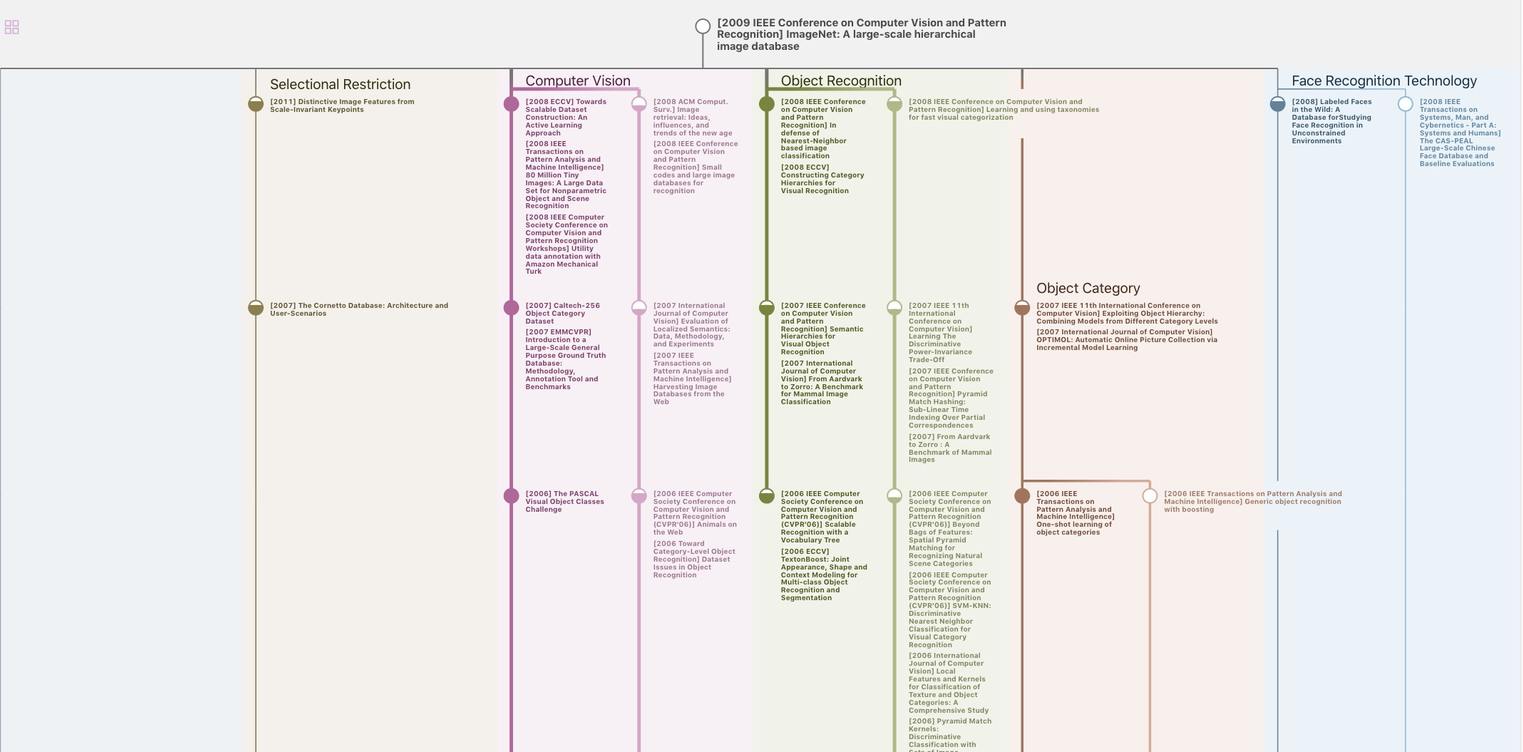
生成溯源树,研究论文发展脉络
Chat Paper
正在生成论文摘要