Spectral-Temporal Transformer for Hyperspectral Image Change Detection
REMOTE SENSING(2023)
摘要
Deep-Learning-based (DL-based) approaches have achieved remarkable performance in hyperspectral image (HSI) change detection (CD). Convolutional Neural Networks (CNNs) are often employed to capture fine spatial features, but they do not effectively exploit the spectral sequence information. Furthermore, existing Siamese-based networks ignore the interaction of change information during feature extraction. To address this issue, we propose a novel architecture, the Spectral-Temporal Transformer (STT), which processes the HSI CD task from a completely sequential perspective. The STT concatenates feature embeddings in spectral order, establishing a global spectrum-time-receptive field that can learn different representative features between two bands regardless of spectral or temporal distance, thereby strengthening the learning of temporal change information. Via the multi-head self-attention mechanism, the STT is capable of capturing spectral-temporal features that are weighted and enriched with discriminative sequence information, such as inter-spectral correlations, variations, and time dependency. We conducted experiments on three HSI datasets, demonstrating the competitive performance of our proposed method. Specifically, the overall accuracy of the STT outperforms the second-best method by 0.08%, 0.68%, and 0.99% on the Farmland, Hermiston, and River datasets, respectively.
更多查看译文
关键词
hyperspectral image,change detection,spectral-temporal attention,transformer
AI 理解论文
溯源树
样例
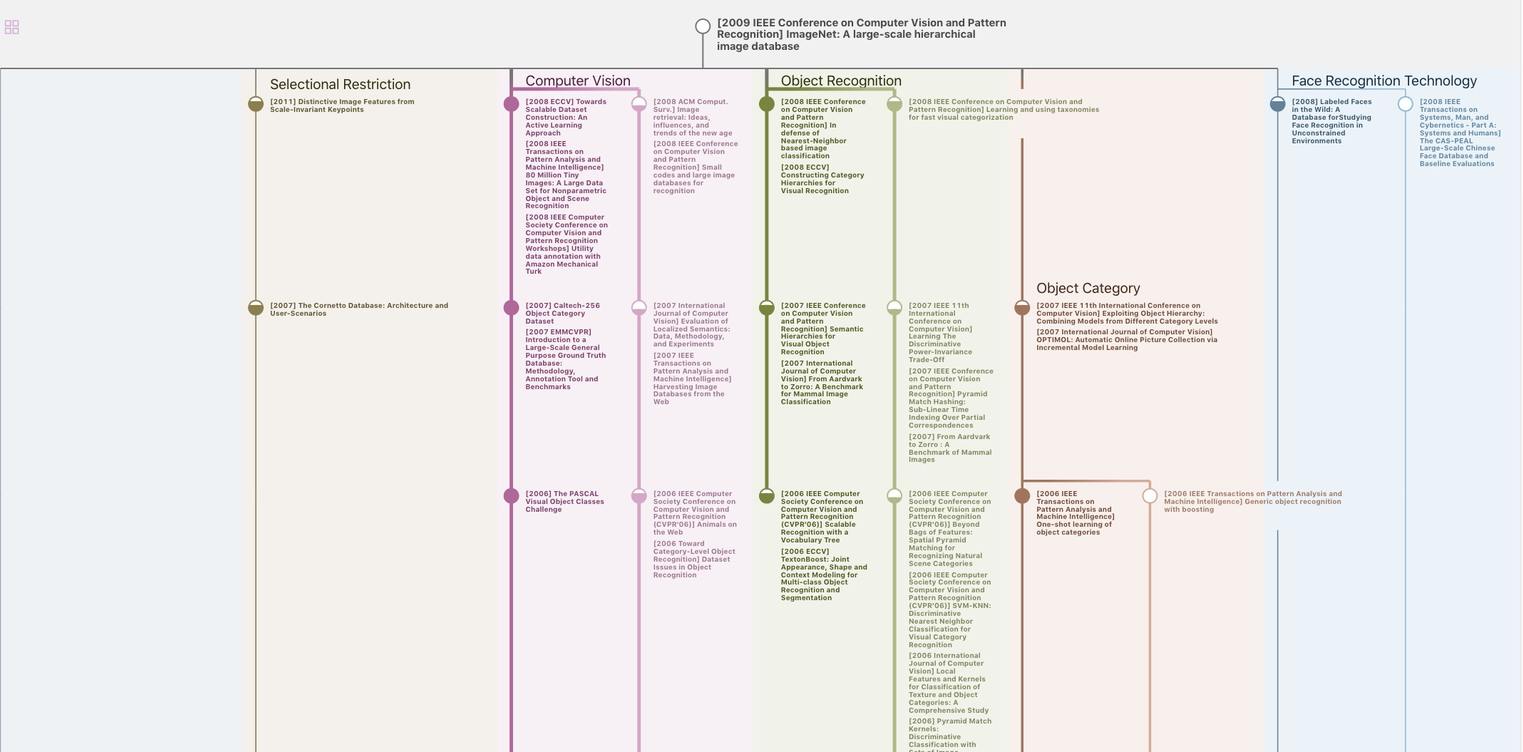
生成溯源树,研究论文发展脉络
Chat Paper
正在生成论文摘要