A multi-algorithm integration machine learning approach for high cycle fatigue prediction of a titanium alloy in aero-engine
ENGINEERING FRACTURE MECHANICS(2023)
摘要
Machine learning (ML) approach based on big-data is a powerful tool of fatigue life prediction and some potential results can be obtained. The present study introduces a multi-algorithm integration ML approach named Auto_Gluon (AG) to predict the high cycle fatigue (HCF) life of a titanium alloy TC17 on the basis of numerous experimental fatigue data. The prediction results from AG are compared with those from the commonly-used ML models including Random Forest (RF) and Support Vector Regression (SVR). Then AG is used to explore the key parameters influencing the fatigue life. The present results show the prediction accuracy of AG is better than other ML models. And the most significant category of factors affecting the fatigue life of TC17 is the specimen properties. The study demonstrates that the present ML approach has great potential in fatigue life prediction.
更多查看译文
关键词
Machine learning,Titanium alloy,Fatigue life prediction,High cycle fatigue
AI 理解论文
溯源树
样例
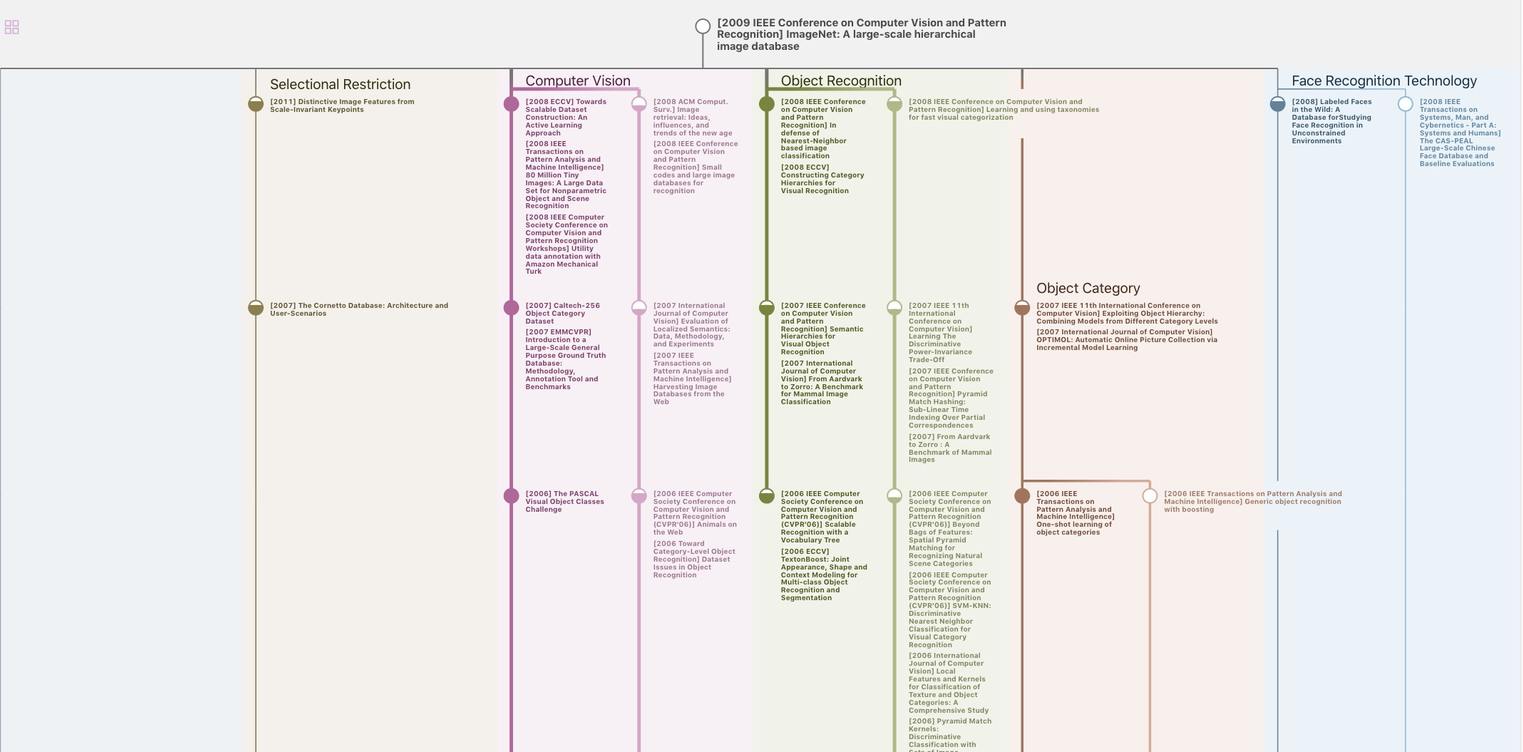
生成溯源树,研究论文发展脉络
Chat Paper
正在生成论文摘要