Enhancing Building Segmentation in Remote Sensing Images: Advanced Multi-Scale Boundary Refinement with MBR-HRNet
REMOTE SENSING(2023)
摘要
Deep learning algorithms offer an effective solution to the inefficiencies and poor results of traditional methods for building a footprint extraction from high-resolution remote sensing imagery. However, the heterogeneous shapes and sizes of buildings render local extraction vulnerable to the influence of intricate backgrounds or scenes, culminating in intra-class inconsistency and inaccurate segmentation outcomes. Moreover, the methods for extracting buildings from very high-resolution (VHR) images at present often lose spatial texture information during down-sampling, leading to problems, such as blurry image boundaries or object sticking. To solve these problems, we propose the multi-scale boundary-refined HRNet (MBR-HRNet) model, which preserves detailed boundary features for accurate building segmentation. The boundary refinement module (BRM) enhances the accuracy of small buildings and boundary extraction in the building segmentation network by integrating edge information learning into a separate branch. Additionally, the multi-scale context fusion module integrates feature information of different scales, enhancing the accuracy of the final predicted image. Experiments on WHU and Massachusetts building datasets have shown that MBR-HRNet outperforms other advanced semantic segmentation models, achieving the highest intersection over union results of 91.31% and 70.97%, respectively.
更多查看译文
关键词
building segmentation,remote sensing images,remote sensing,multi-scale,mbr-hrnet
AI 理解论文
溯源树
样例
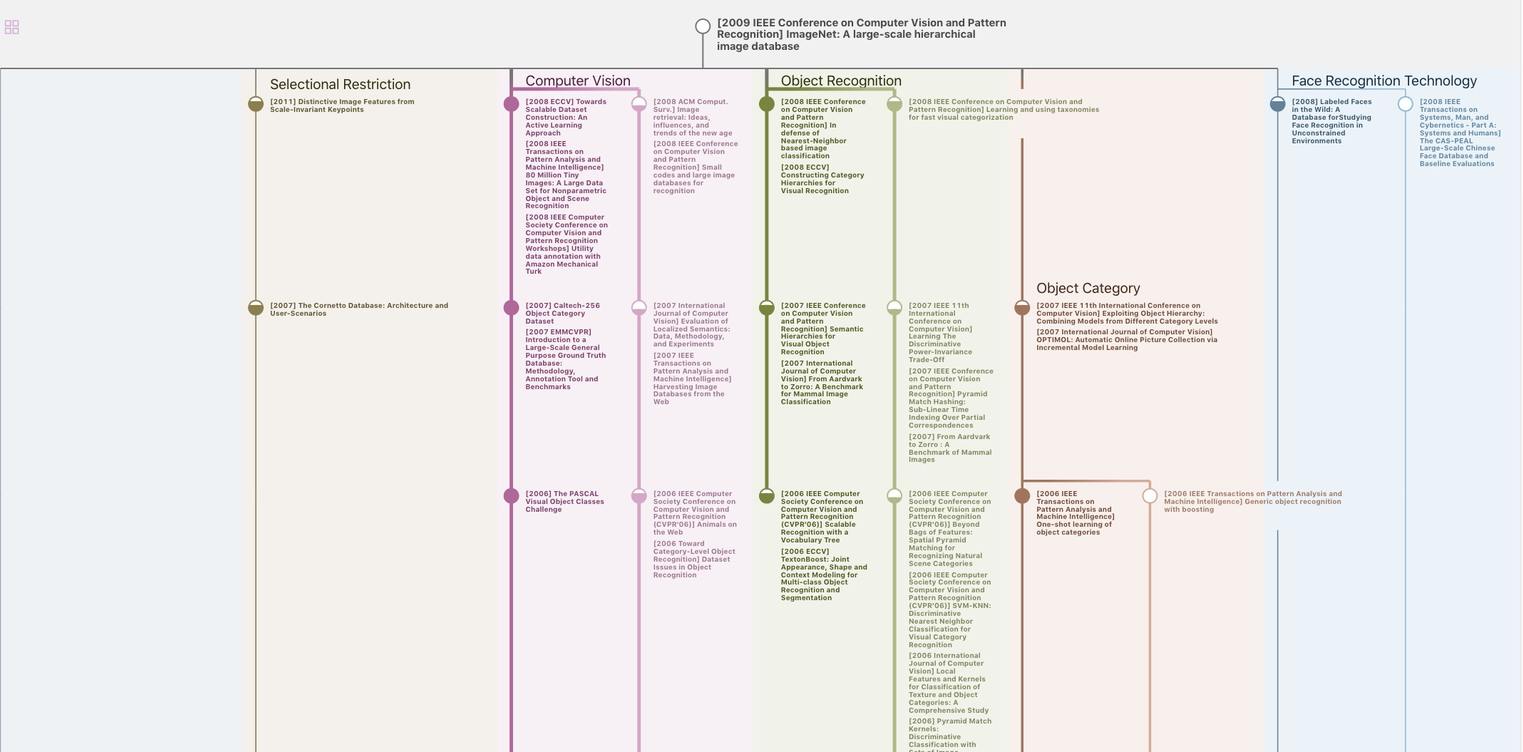
生成溯源树,研究论文发展脉络
Chat Paper
正在生成论文摘要