Physics-informed deep learning to forecast (M)over-cap(max) during hydraulic fracturing
SCIENTIFIC REPORTS(2023)
摘要
Short-term forecasting of estimated maximum magnitude ((M) over cap (max)) is crucial to mitigate risks of induced seismicity during fluid stimulation. Most previous methods require real-time injection data, which are not always available. This study proposes two deep learning (DL) approaches, along with two data partitioning methods, that rely solely on preceding patterns of seismicity. The first approach forecasts (M) over cap (max) directly using DL; the second incorporates physical constraints by using DL to forecast seismicity rate, which is then used to estimate (M) over cap (max). These approaches are tested using a hydraulic-fracture monitoring dataset from western Canada. We find that direct DL learns from previous seismicity patterns to provide an accurate forecast, albeit with a time lag that limits its practical utility. The physics-informed approach accurately forecasts changes in seismicity rate, but sometimes under (or over-) estimates (M) over cap (max). We propose that significant exceedance of (M) over cap (max) may herald the onset of runaway fault rupture.
更多查看译文
AI 理解论文
溯源树
样例
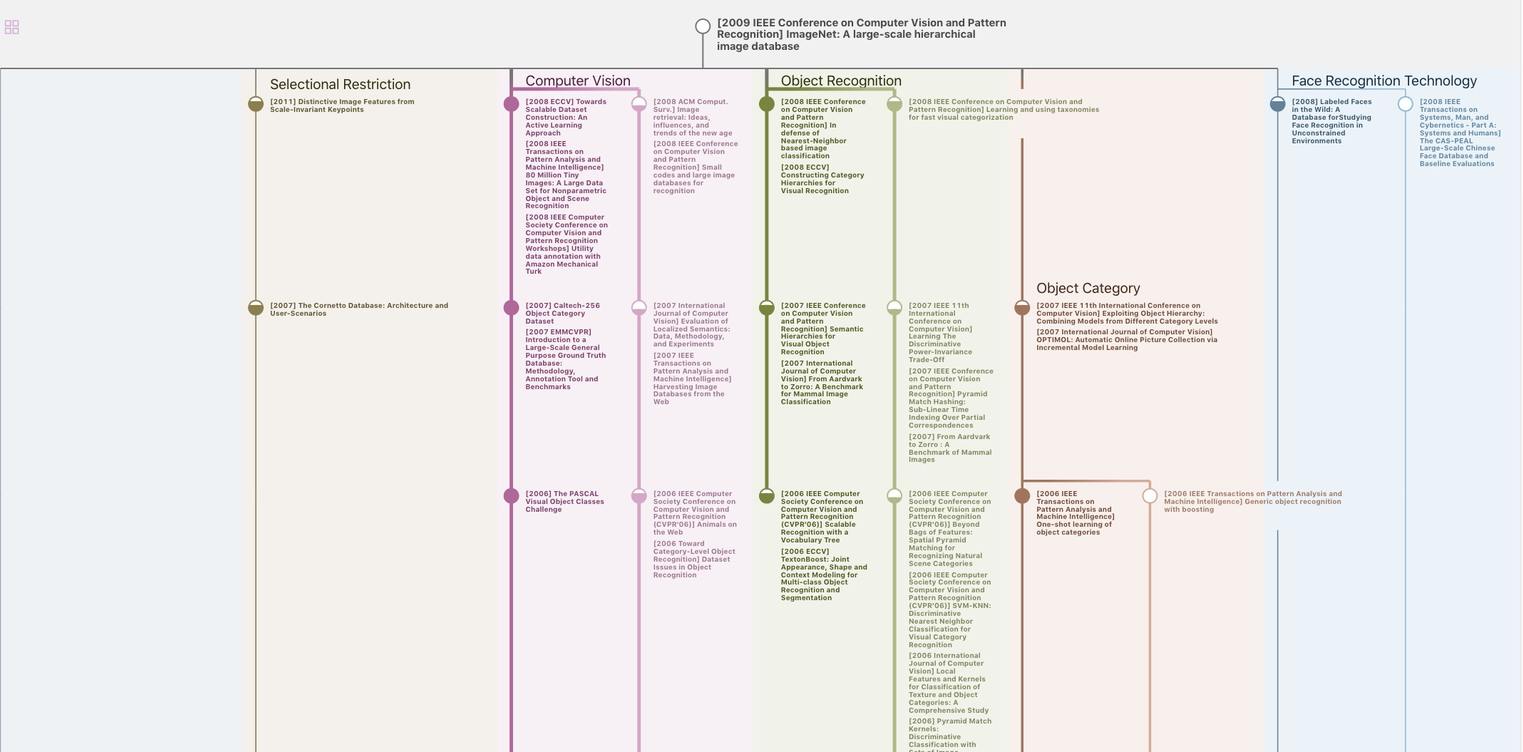
生成溯源树,研究论文发展脉络
Chat Paper
正在生成论文摘要