Lightweight Quality Evaluation of Generated Samples and Generative Models
APSIPA TRANSACTIONS ON SIGNAL AND INFORMATION PROCESSING(2023)
摘要
Although there are metrics to evaluate the performance of generative models, little research is conducted on the quality evaluation of individual generated samples. A lightweight generated sample quality evaluation (LGSQE) method is proposed in this work. LGSQE trains a binary classifier to differentiate real and synthetic images from a generative model and, then, uses it to assign a soft label between zero and one to a generated sample as its quality index. LGSQE can reject poor generations and serve as a post-processing module for quality control. Furthermore, by aggregating quality indices of a large number of generated samples, LGSQE offers four metrics (i.e., classification accuracy (Acc), the area under the curve (AUC), precision, and recall) to evaluate the performance of a generative model as a byproduct. LGSQE demands a significantly smaller memory size and faster evaluation time while preserving the same rank order predicted by the Frechet Inception Distance (FID). Extensive experiments are conducted to demonstrate the effectiveness and efficiency of LGSQE.
更多查看译文
关键词
generated samples,quality,evaluation,models
AI 理解论文
溯源树
样例
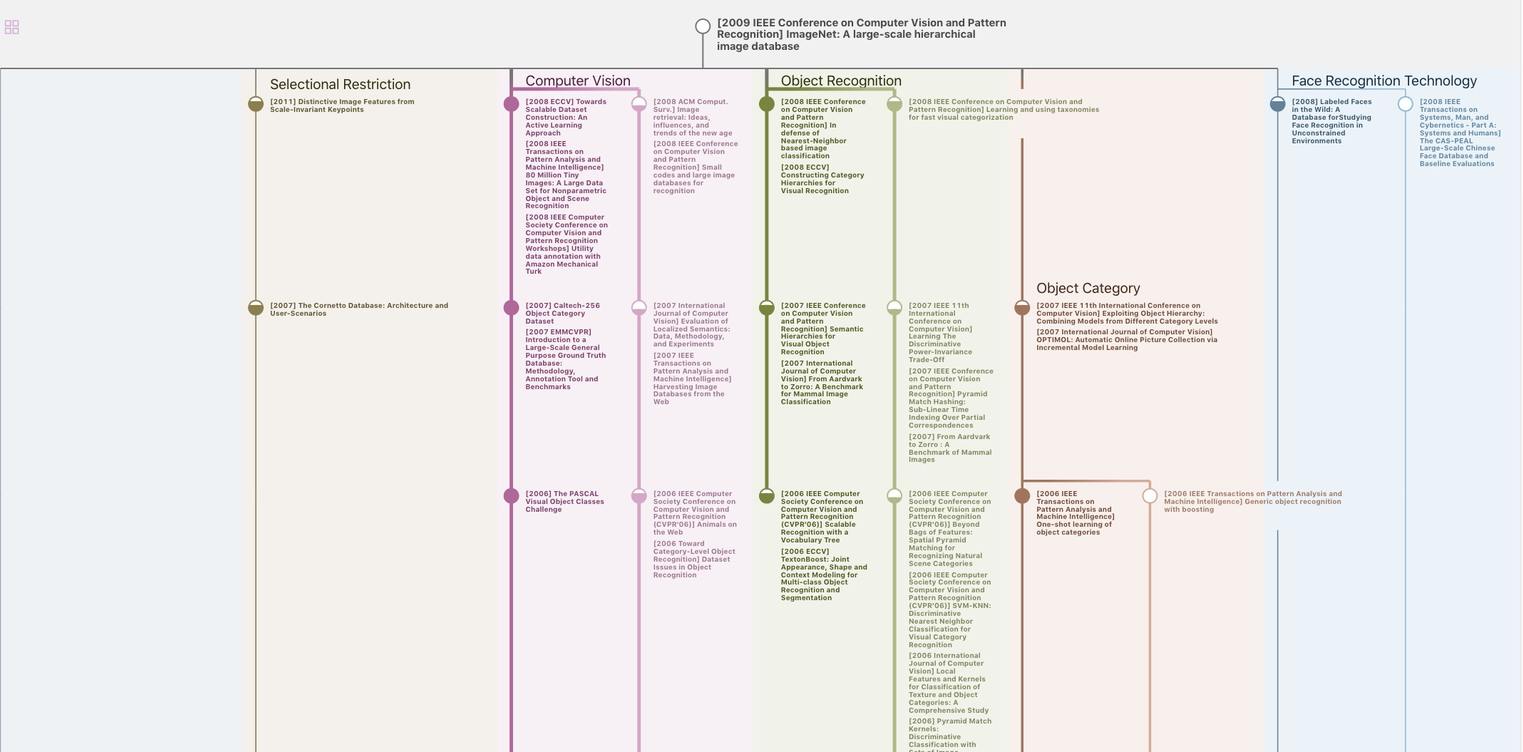
生成溯源树,研究论文发展脉络
Chat Paper
正在生成论文摘要