Matrix Factorization for Globally Consistent Periodic Flow Prediction in Taxi Systems
TRANSPORTATION RESEARCH RECORD(2023)
摘要
Predicting flow in taxi systems can improve taxi operations and reduce passengers' waiting time. With the increasing availability of taxi mobility data sets, more studies have tried to analyze and model taxi demand. However, most existing works merely attempt to predict the number of pick-ups and drop-offs by using local observations. Nevertheless, predicting the passengers' pair-wise flow specifically through simultaneous consideration of local variations and network communities in the taxi systems has been neglected. In this paper, we introduce a globally consistent periodic flow prediction for taxi systems that integrates communities in the matrix factorization model. We apply non-negative tensor factorization to capture the periodic variations in the passenger flow in different areas and predict the flow (and pick-ups and drop-offs) in the next periods by integrating the most recent observation with detected patterns from historical data. The results show an improvement in the prediction accuracy from some baselines and a state-of-the-art method. We also propose a method for visualizing flow that potentially improves taxi operations by assisting drivers with selecting passengers.
更多查看译文
关键词
taxi systems,matrix factorization,prediction
AI 理解论文
溯源树
样例
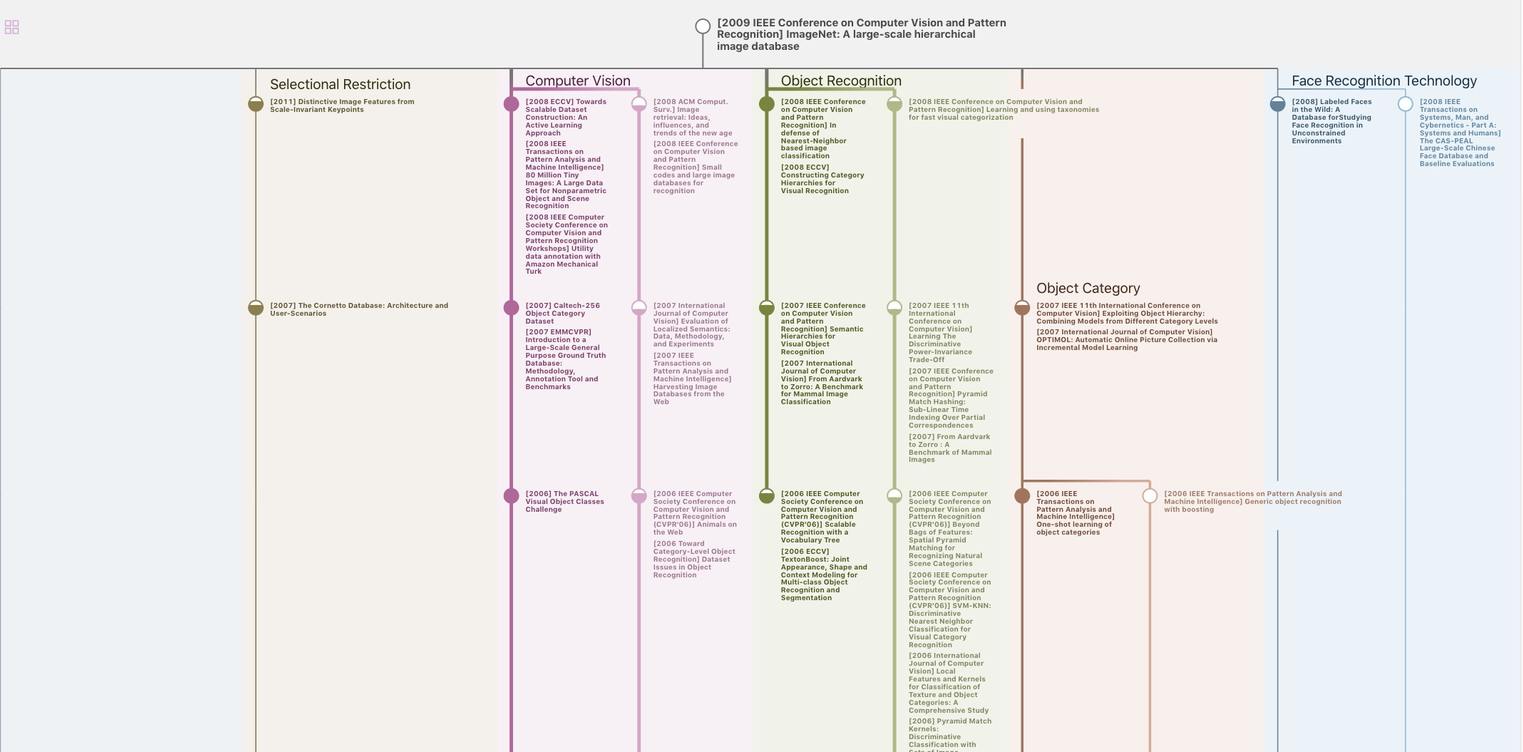
生成溯源树,研究论文发展脉络
Chat Paper
正在生成论文摘要