A Combined Multi-Classification Network Intrusion Detection System Based on Feature Selection and Neural Network Improvement
APPLIED SCIENCES-BASEL(2023)
摘要
Featured Application Increased parallelism in edge network security issues, Feature loss handling. Feature loss in IoT scenarios is a common problem. This situation poses a greater challenge in terms of real-time and accuracy for the security of intelligent edge computing systems, which also includes network security intrusion detection systems (NIDS). Losing some packet information can easily confuse NIDS and cause an oversight of security systems. We propose a novel network intrusion detection framework based on an improved neural network. The new framework uses 23 subframes and a mixer for multi-classification work, which improves the parallelism of NIDS and is more adaptable to edge networks. We also incorporate the K-Nearest Neighbors (KNN) algorithm and Genetic Algorithm (GA) for feature selection, reducing parameters, communication, and memory overhead. We named the above system as Combinatorial Multi-Classification-NIDS (CM-NIDS). Experiments demonstrate that our framework can be more flexible in terms of the parameters of binary classification, has a fairly high accuracy in multi-classification, and is less affected by feature loss.
更多查看译文
关键词
feature selection,neural network improvement,multi-classification
AI 理解论文
溯源树
样例
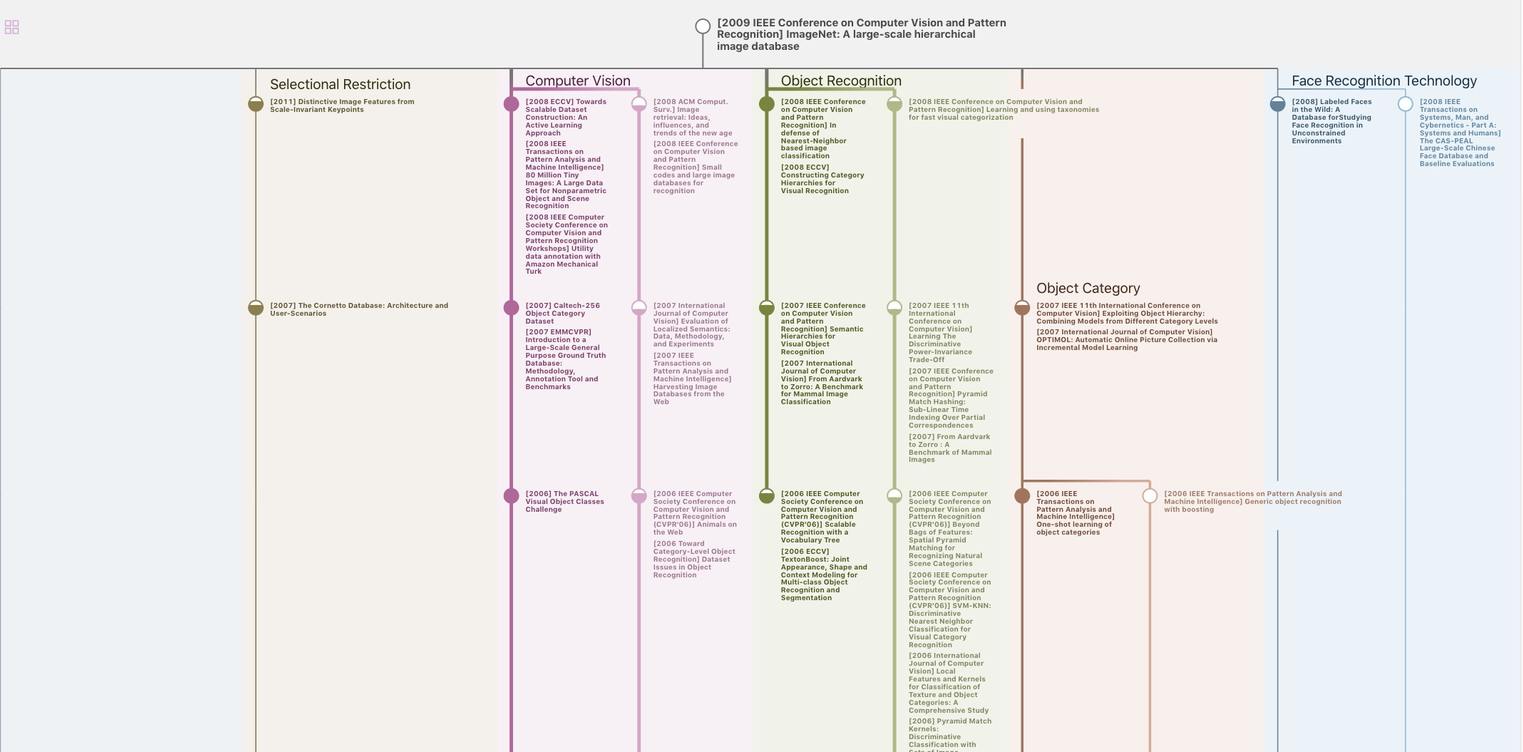
生成溯源树,研究论文发展脉络
Chat Paper
正在生成论文摘要