A Model-Free-Based Control Method for Robot Manipulators: Achieving Prescribed Performance and Ensuring Fixed Time Stability
APPLIED SCIENCES-BASEL(2023)
摘要
This paper addresses three significant challenges in controlling robot manipulators: improving response time, minimizing steady-state errors and chattering, and enhancing controller robustness. It also focuses on eliminating the need for computing the robot's dynamic model and unknown functions, as well as achieving global fixed-time convergence and the prescribed performance for the control system. To achieve these objectives, a fixed-time sliding mode function is designed, which uses transformation errors to achieve prescribed control performance, with adjustments made to the maximum overshoot, convergence time, and tracking errors to keep them within predefined bounds. Additionally, a radial basis function neural network (RBFNN) is used to eliminate the need for knowledge of the robot's dynamical properties and uncertain terms, which also reduces negative chattering. Finally, a novel fixed-time terminal sliding mode control (TSMC) algorithm is developed for robot manipulators without using their dynamical model. The fixed-time stability of the control system is thoroughly demonstrated by applying Lyapunov criteria and conducting simulations on a robot manipulator to showcase its effectiveness.
更多查看译文
关键词
control of robots,neural networks,fixed-time control,prescribed performance control
AI 理解论文
溯源树
样例
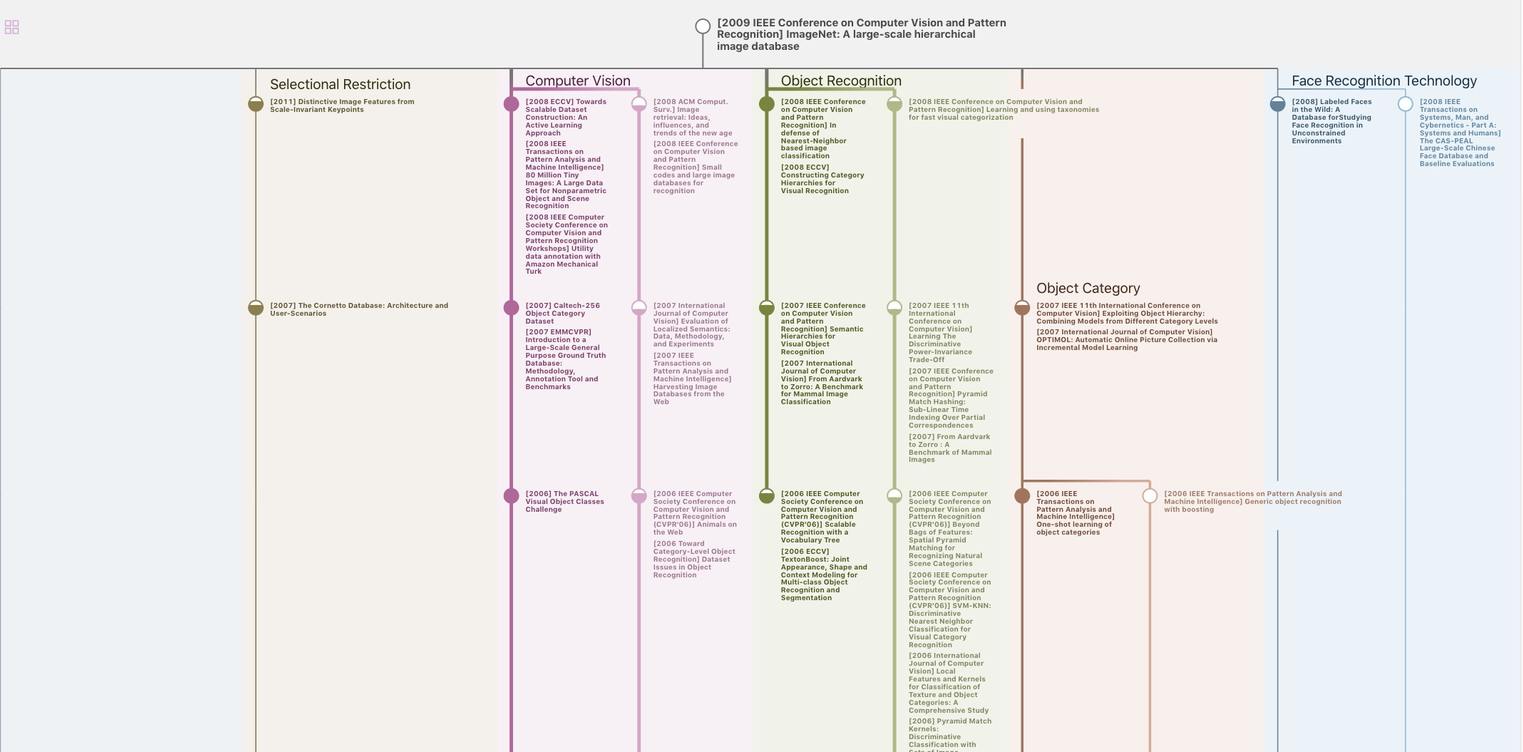
生成溯源树,研究论文发展脉络
Chat Paper
正在生成论文摘要