Reduced-Order Machine-Learning Model for Transmission Loss Prediction in Underwater Acoustics
IEEE JOURNAL OF OCEANIC ENGINEERING(2023)
摘要
Autonomous underwater vehicles (AUVs) typically rely on underwater sound to communicate with sound channel physics defining communication performance. Current acoustic propagation models incorporate channel information and physics but are computationally expensive to run onboard an AUV. This work explores reduced-order proxies for these models through a machine-learning approach that can use in-situ measurements of observed sound-speed profile (SSP) to quickly provide transmission loss (TL) propagation information for vehicles to optimize communication performance through repositioning behaviors. The vertical structure from a year-long time series of mooring observations from offshore San Diego is decomposed with empirical orthogonal functions to reduce the SSPs to three unique amplitudes that explain 95.6% of the total variance. The acoustic propagation model Bellhop is used to estimate TL for a given SSP and different thresholds of TL are used to represent communications performance. Machine-learning models are trained with binarized Bellhop outputs to indicate locations of improved underwater acoustic communications. Amplitude values, desired TL, vehicle depth, and range are used as inputs into machine-learning models for optimizing TL. The best trained model was a random forest (ensemble) network with a 93.3% dice score. The decision tree had a faster prediction speed, relative to Bellhop, with similar prediction accuracy as the ensemble. This work aims to improve sound awareness of AUVs by generating SSP-informed communication performance maps.
更多查看译文
关键词
Autonomous underwater vehicles (AUVs),machine learning acoustics,underwater acoustic communications
AI 理解论文
溯源树
样例
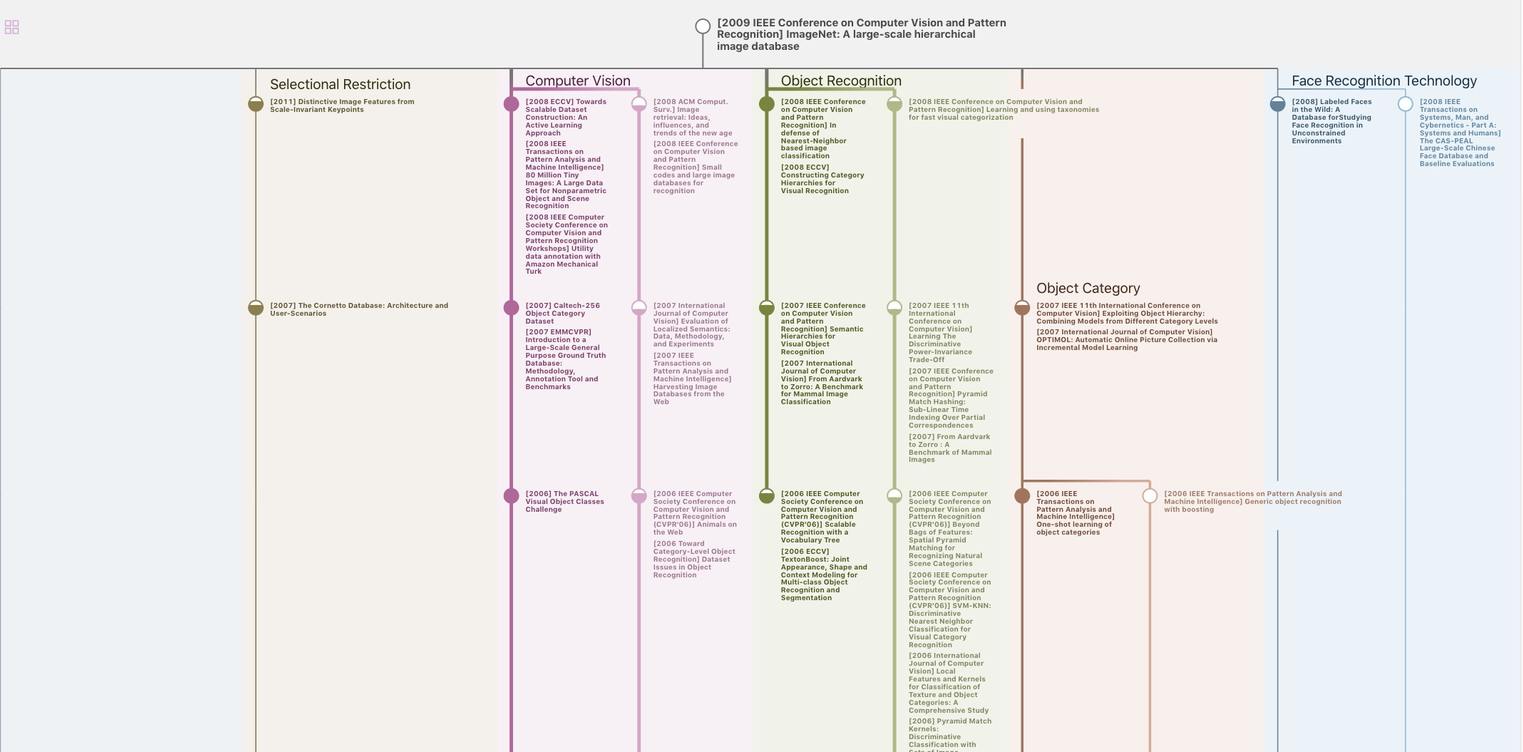
生成溯源树,研究论文发展脉络
Chat Paper
正在生成论文摘要