Feature extraction-reduction and machine learning for fault diagnosis in PV panels
SOLAR ENERGY(2023)
摘要
With the rapid expansion and installation of Photovoltaic (PV) power plants, developing a proper Fault Detection and Diagnosis (FDD) strategy has become a significant issue within the objective to organize and plan for maintenance and reduce repair time, which in turn ensures the availability of operated systems. In this context, supervised Machine Learning (ML) algorithms are undergoing extensive development and their performances are analyzed in terms of different metrics including computation time detection accuracy, precision, recall, and F1 score in addition to False Alarm and missed detection rates. However, such algorithms generally require the extraction of significant features to handle irrelevant and redundant features while reducing the computational burden. Considering that different dimensionality reduction methods directly impact the FDD performances, this paper presents a novel Data Dimensionality Reduction Strategy (DDRS) based on a proposed algorithm for Information Gain (IG) score optimal threshold determination. This strategy also relies on Principal Component Analysis (PCA) to obtain the optimal subset which represents the lowest dimensional feature in the training and testing stages of different supervised ML algorithms. FDD performances are then evaluated by using faulty and non-faulty datasets acquired experimentally from a Grid-connected PV system (GPVS) emulator. The experimental results show the efficiency of the proposed strategy and its ability to improve the FDD based on supervised ML algorithms.
更多查看译文
关键词
Fault diagnosis,PV Plant,Feature filtering,Feature extraction,Dimensionality reduction,Machine Learning
AI 理解论文
溯源树
样例
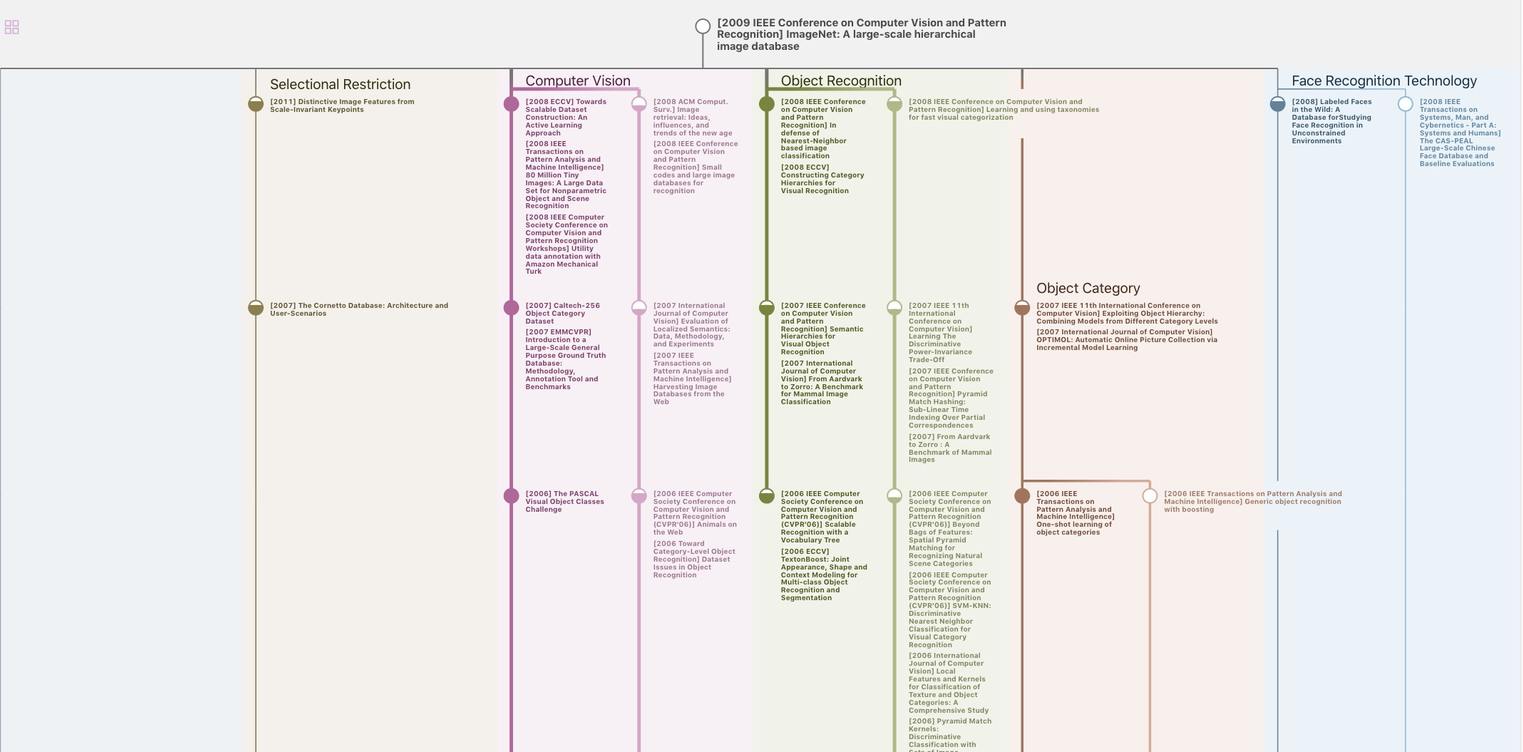
生成溯源树,研究论文发展脉络
Chat Paper
正在生成论文摘要