Machine Learning-Based Efficient Discovery of Software Vulnerability for Internet of Things
INTELLIGENT AUTOMATION AND SOFT COMPUTING(2023)
摘要
With the development of the 5th generation of mobile communication (5G) networks and artificial intelligence (AI) technologies, the use of the Internet of Things (IoT) has expanded throughout industry. Although IoT networks have improved industrial productivity and convenience, they are highly dependent on nonstandard protocol stacks and open-source-based, poorly validated software, resulting in several security vulnerabilities. However, conventional AI-based software vulnerability discovery technologies cannot be applied to IoT because they require excessive memory and computing power. This study developed a technique for optimizing training data size to detect software vulnerabilities rapidly while maintaining learning accuracy. Experimental results using a software vulnerability classification dataset showed that different optimal data sizes did not affect the learning performance of the learning models. Moreover, the minimal data size required to train a model without performance degradation could be determined in advance. For example, the random forest model saved 85.18% of memory and improved latency by 97.82% while maintaining a learning accuracy similar to that achieved when using 100% of data, despite using only 1%.
更多查看译文
关键词
software vulnerability,efficient discovery,learning-based
AI 理解论文
溯源树
样例
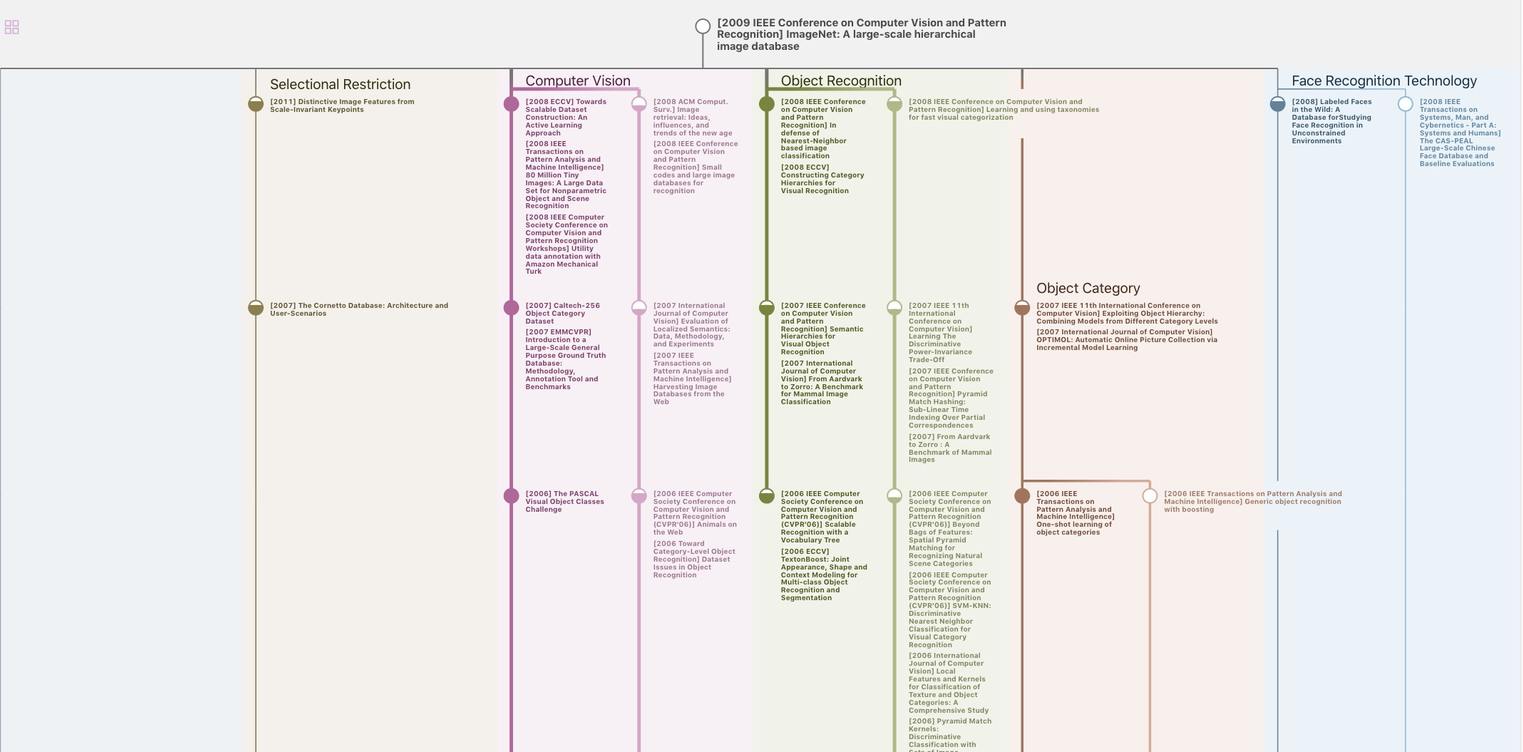
生成溯源树,研究论文发展脉络
Chat Paper
正在生成论文摘要