Anomaly Detection for Cloud Systems with Dynamic Spatiotemporal Learning
INTELLIGENT AUTOMATION AND SOFT COMPUTING(2023)
摘要
As cloud system architectures evolve continuously, the interac-tions among distributed components in various roles become increasingly complex. This complexity makes it difficult to detect anomalies in cloud systems. The system status can no longer be determined through individual key performance indicators (KPIs) but through joint judgments based on syn-ergistic relationships among distributed components. Furthermore, anomalies in modern cloud systems are usually not sudden crashes but rather grad-ual, chronic, localized failures or quality degradations in a weakly available state. Therefore, accurately modeling cloud systems and mining the hidden system state is crucial. To address this challenge, we propose an anomaly detection method with dynamic spatiotemporal learning (AD-DSTL). AD-DSTL leverages the spatiotemporal dynamics of the system to train an end -to-end deep learning model driven by data from system monitoring to detect underlying anomalous states in complex cloud systems. Unlike previous work that focuses on the KPIs of separate components, AD-DSTL builds a model for the entire system and characterizes its spatiotemporal dynamics based on graph convolutional networks (GCN) and long short-term memory (LSTM). We validated AD-DSTL using four datasets from different backgrounds, and it demonstrated superior robustness compared to other baseline algorithms. Moreover, when raising the target exception level, both the recall and precision of AD-DSTL reached approximately 0.9. Our experimental results demon-strate that AD-DSTL can meet the requirements of anomaly detection for complex cloud systems.
更多查看译文
关键词
dynamic spatiotemporal learning,anomaly,cloud systems,detection
AI 理解论文
溯源树
样例
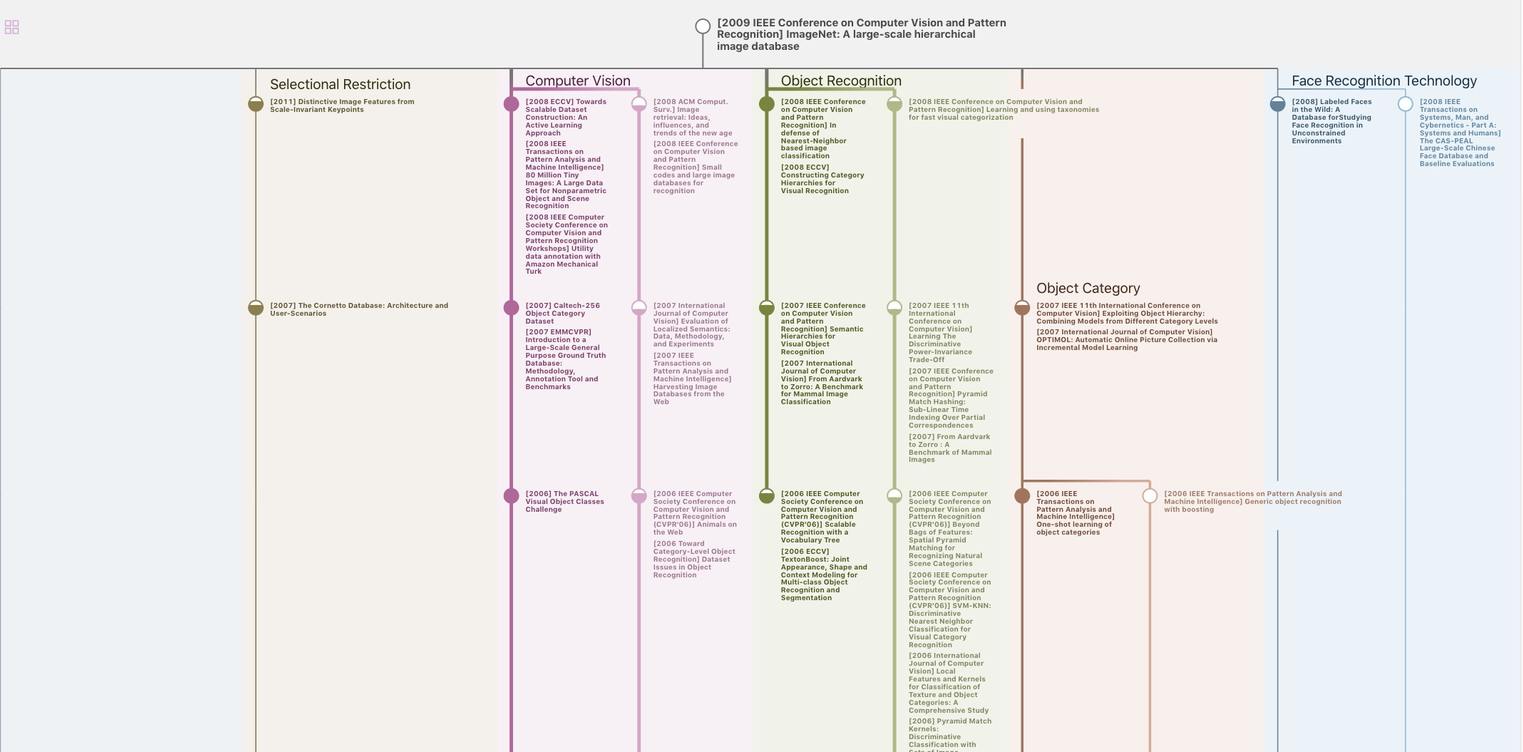
生成溯源树,研究论文发展脉络
Chat Paper
正在生成论文摘要