Surface roughness prediction of large shaft grinding via attentional CNN-LSTM fusing multiple process signals
INTERNATIONAL JOURNAL OF ADVANCED MANUFACTURING TECHNOLOGY(2023)
摘要
Surface roughness is an important indicator for shaft grinding, and its prediction is always a core issue in practice. The surface roughness of each part for a large shaft would be different with same processing parameters, which leads to traditional processing parameter-based prediction being ineffective; thus, the surface roughness prediction of large shaft grinding is more difficult. This paper proposes a surface roughness prediction method for large shaft grinding based on deep learning, and three kinds of process signals are selected as the inputs, including spindle current, vibration, and acoustic emission. An experiment considering the contribution degree of each processing parameter is designed to generate the dataset on a large shaft grinding platform, and the appropriate surface roughness intervals are divided. The multiple process signals are fused through an attentional CNN-LSTM architecture, in which the CNN is used to extract the features of the process signals, the LSTM deals with the sequential output of CNN, and the self-attention mechanism is utilized to realize the automatic weight allocation. Moreover, the model degradation problem after long-term use of the grinding platform is solved by the parameters fine-tuning approach. The experimental results show that the proposed method achieves the best accuracy than all comparative items, and the model performance can be recovered through parameters fine-tuning; thus, the proposed method is effective.
更多查看译文
关键词
Surface roughness prediction,Large shaft grinding,Attentional CNN-LSTM,Multiple process signals
AI 理解论文
溯源树
样例
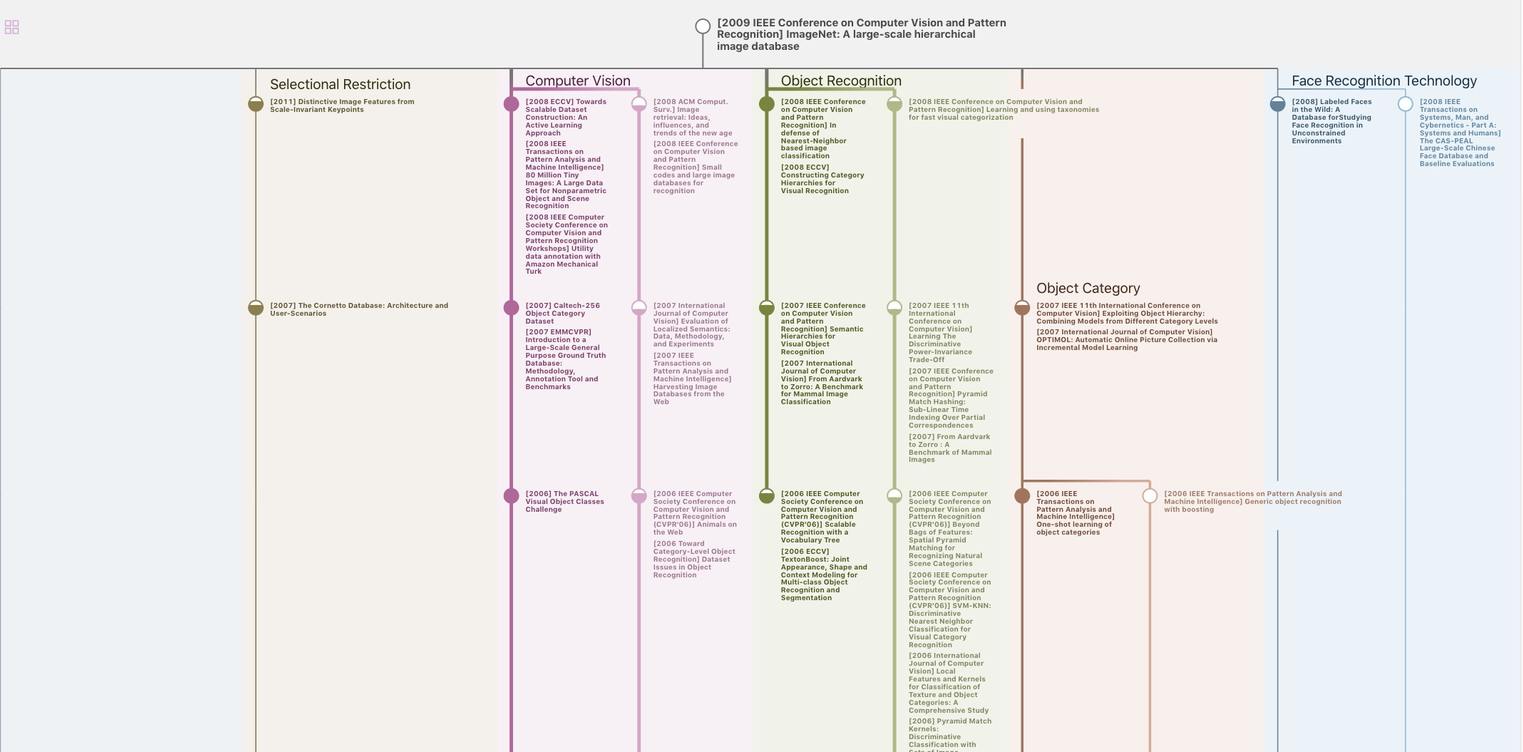
生成溯源树,研究论文发展脉络
Chat Paper
正在生成论文摘要