Jacobian Based Nonlinear Algorithms for Prediction of Optimized RF MEMS Switch Dimensions
TRANSACTIONS ON ELECTRICAL AND ELECTRONIC MATERIALS(2023)
摘要
This communication discusses the role of nonlinear algorithms in training the neural network, which predicts the optimized RF MEMS switch dimensions. A dedicated dataset, i.e., DrTLN-RF-MEMS-SWITCH-DATASET-v1, was created by considering the most appropriate input and output variable suitable to predict the cantilever dimensions, crab leg and serpentine structure-based RF MEMS switches. The distinct artificial neural networks (ANN) performance is analysed using various training methods. The hardware implementation possible neural network algorithms, i.e., Fitting and Cascade Feed Forward Network, are considered for learning and prediction. The ANN algorithm's performance in predicting and optimizing RF MEMS switch is analysed using nonlinear training methods like Levenberg–Marquardt (LM) and Scaled Conjugate Gradient (SCG). The cascaded forward network with LM training combination offers the best performance compared with other varieties. A comprehensive study is performed using neural networks and finite element simulation results. The study revealed that the error percentage is below 15.08% for most of the parameters.
更多查看译文
关键词
jacobian based nonlinear algorithms,switch
AI 理解论文
溯源树
样例
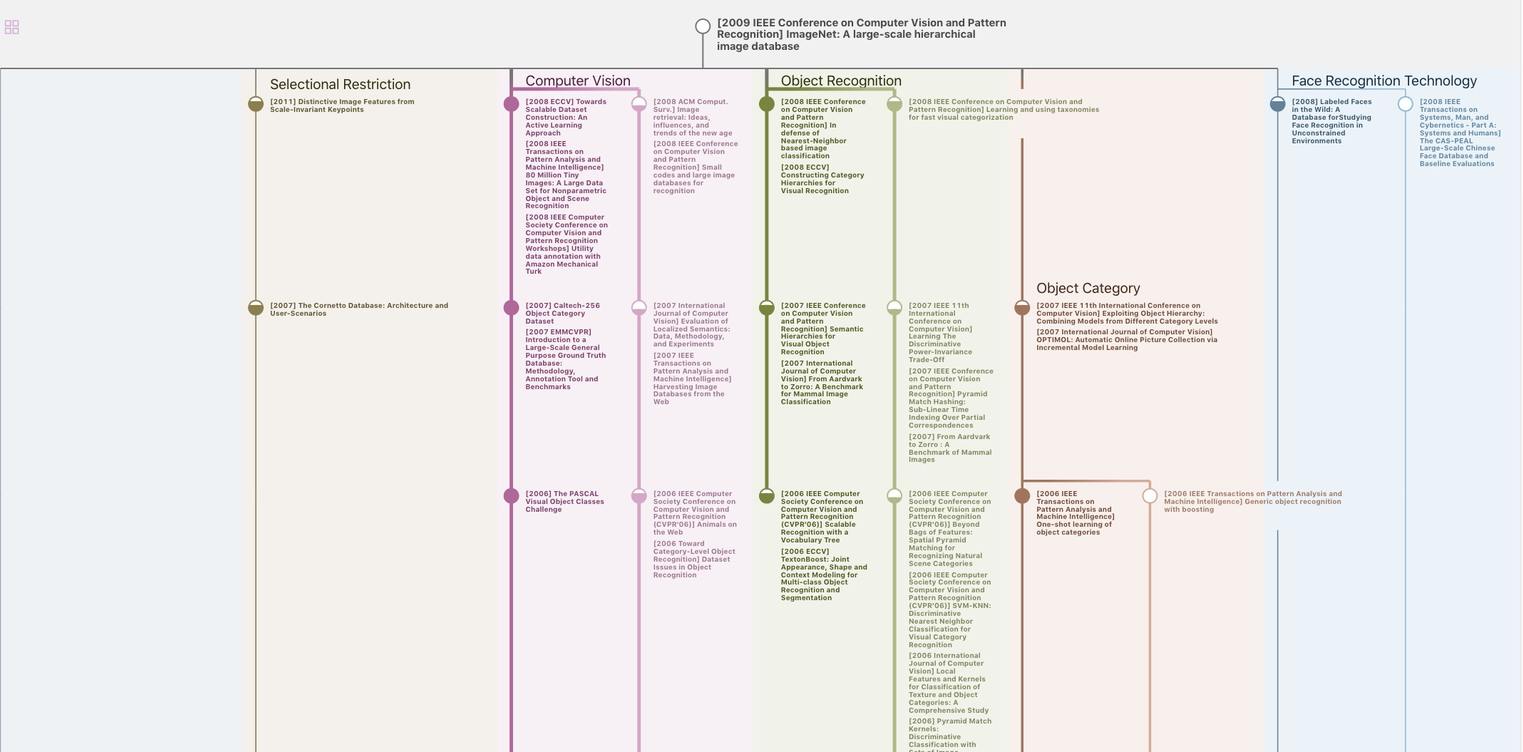
生成溯源树,研究论文发展脉络
Chat Paper
正在生成论文摘要