A feature-interaction knowledge graph completion algorithm based on information selection
JOURNAL OF NONLINEAR AND CONVEX ANALYSIS(2023)
摘要
Knowledge graph completion is the task of inferring missing facts from existing data in a knowledge graph. Currently, most domain knowledge completion models model the relationships between entities and relations. It has been found that enhancing the feature interaction between entities and relations can improve completion performance. However, most feature interaction models rely on the recombination of features which has a series of shortcomings that include insufficient interaction modeling and damage to the spatial structural information. Moreover, the importance degrees of features in the knowledge graph are easily ignored. In order to address the above problems, this study proposes a feature-interaction knowledge graph completion algorithm based on information selection. The proposed knowledge graph model, G-FiBiNet, combining a gate structure and bilinear feature interaction, can dynamically learn the importance degrees of features and model fine-grained feature interaction. The gating mechanism is used for selecting the important information from features (i.e., information selection), while the bilinear structure increases the fusion of features between entities and relations so as to enhance the model's representation ability and knowledge graph completion performance. It is demonstrated that the proposed G-FiBiNet model achieves notable performance in knowledge graph completion tasks.
更多查看译文
关键词
Knowledge graph completion, CNN, feature interactionn, link prediction
AI 理解论文
溯源树
样例
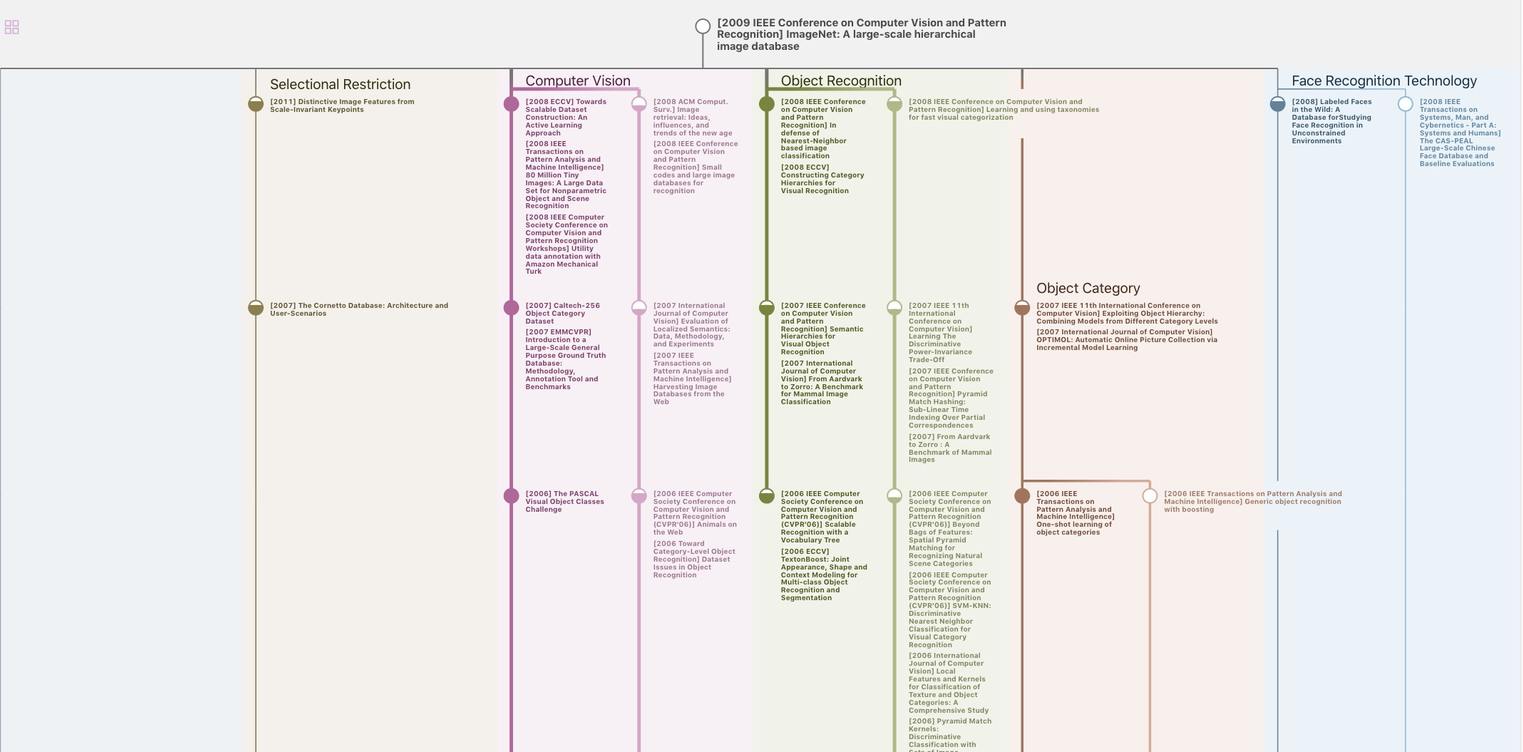
生成溯源树,研究论文发展脉络
Chat Paper
正在生成论文摘要