Unsupervised Learning of non-Hermitian Photonic Bulk Topology
LASER & PHOTONICS REVIEWS(2023)
摘要
Machine-learning has proven useful in distinguishing topological phases. However, there is still a lack of relevant research in the non-Hermitian community, especially from the perspective of the momentum-space. Here, an unsupervised machine-learning method, diffusion maps, is used to study non-Hermitian topologies in the momentum-space. Choosing proper topological descriptors as input datasets, topological phases are successfully distinguished in several prototypical cases, including a line-gapped tight-binding model, a line-gapped Floquet model, and a point-gapped tight-binding model. The datasets can be further reduced when certain symmetries exist. A mixed diffusion kernel method is proposed and developed, which could study several topologies at the same time and give hierarchical clustering results. As an application, a novel phase transition process is discovered in a non-Hermitian honeycomb lattice without tedious numerical calculations. This study characterizes band properties without any prior knowledge, which provides a convenient and powerful way to study topology in non-Hermitian systems.
更多查看译文
关键词
photonic,learning
AI 理解论文
溯源树
样例
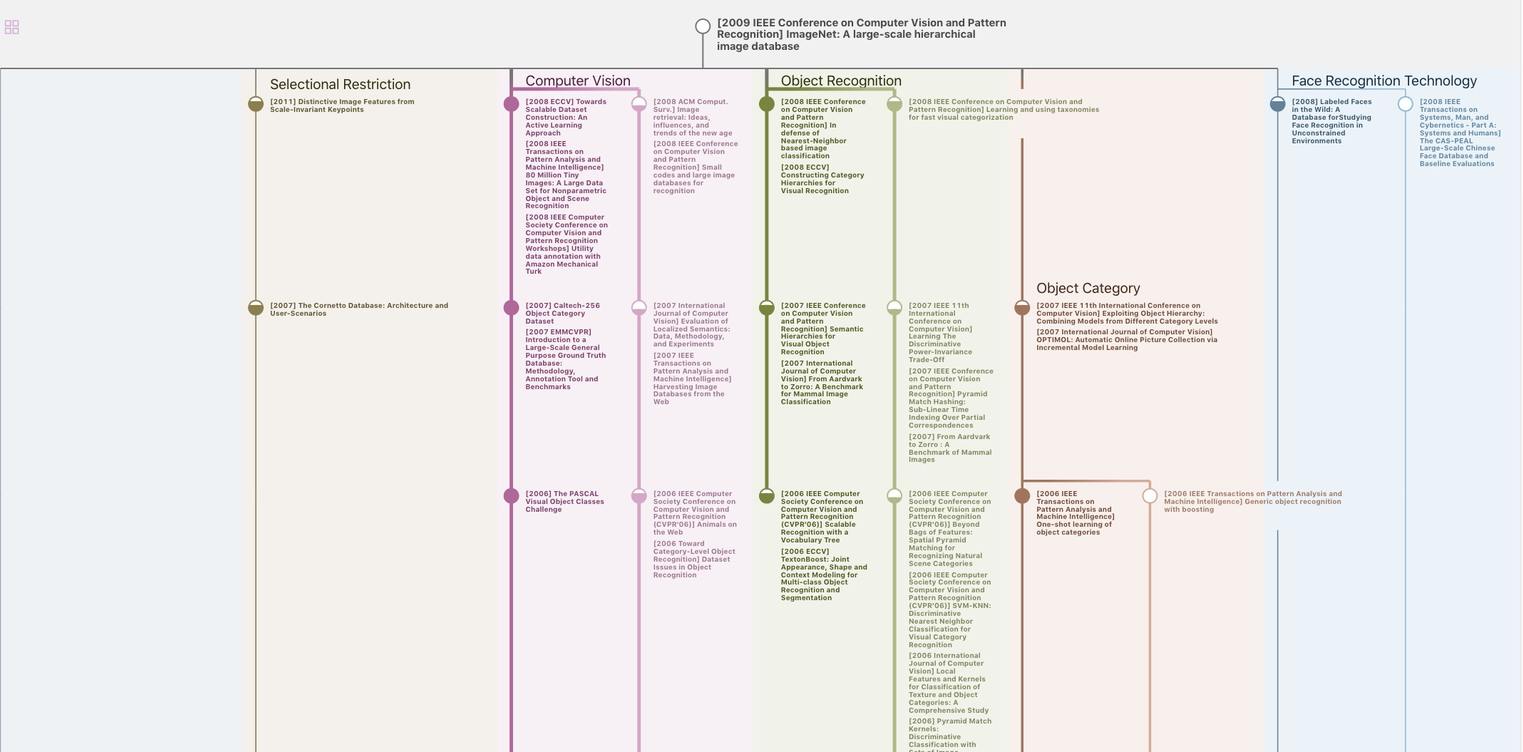
生成溯源树,研究论文发展脉络
Chat Paper
正在生成论文摘要