Post-blast capacity evaluation of concrete-filled steel tubular (CFST) column based on machine learning technique
ADVANCES IN STRUCTURAL ENGINEERING(2023)
摘要
In the present study, the post-blast axial load-bearing capacity of the CFST column under the close-in explosion is investigated. Dynamic response and residual axial load capacity (RALBC) of 1599 circular CFST columns are numerically studied using a validated finite element model. The effects of column dimensions, material properties, blast loading, and axial load ratio are discussed. Based on the huge database, prediction models of RALBC are developed using three commonly used machine learning techniques including Extreme Gradient Boosting (known as XGBoost), Artificial Neutral Network (ANN), and Support Vector Regression (SVR). Comparison results show that the prediction model generated by the XGBoost performs the best among the three methods followed by the ANN and SVR methods. The RALBC of the CFST columns can be estimated rapidly and accurately with the prediction model developed in the present study. Finally, the feature importance of the input variables is analyzed using the additive feature attribution method SHAP (Shapley Additive ExPlanation). The results show that geometric parameters are the main factors impacting the RALBC of the CFST columns under close-in explosion.
更多查看译文
关键词
CFST column,blast loading,post-blast axial load bearing capacity,artificial neural network method,extreme gradient boosting method
AI 理解论文
溯源树
样例
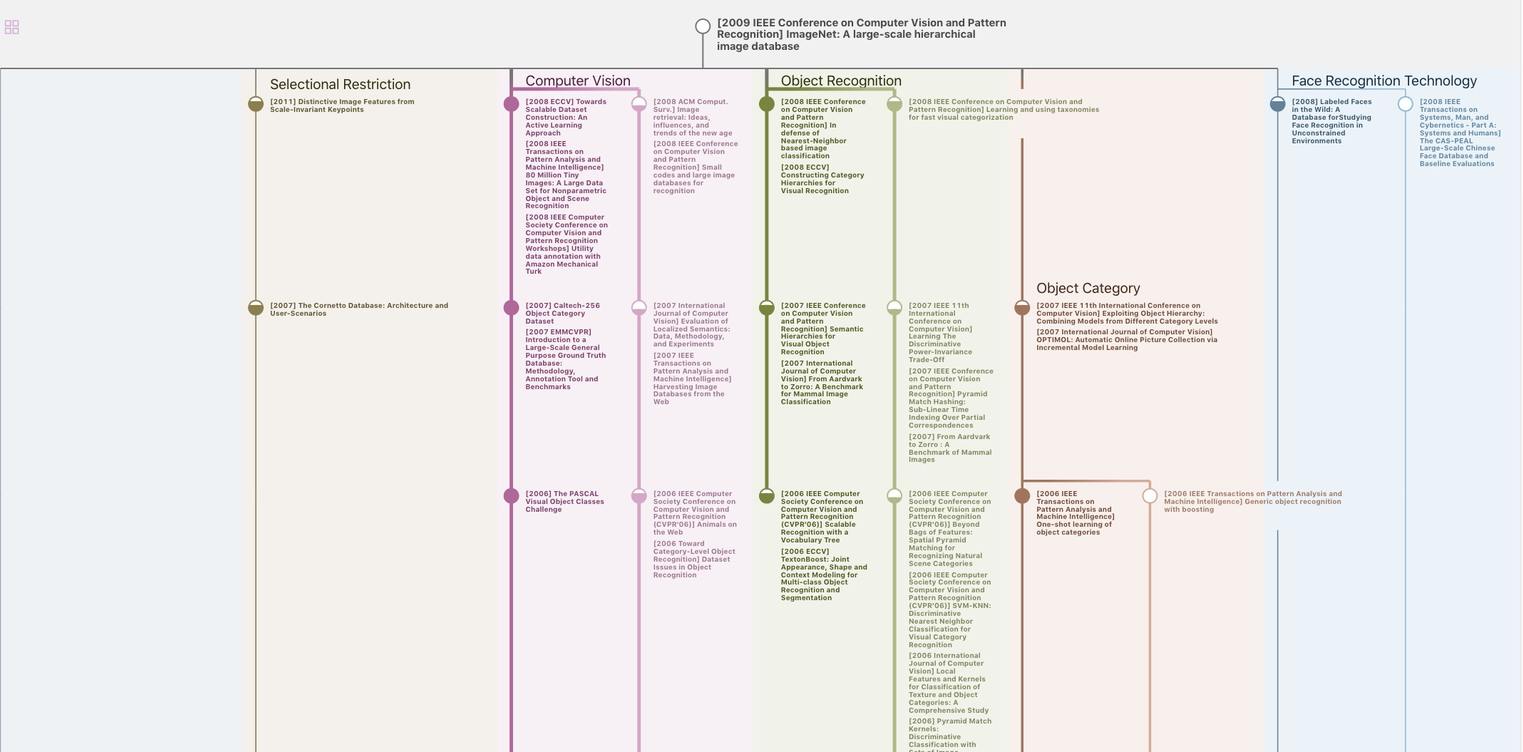
生成溯源树,研究论文发展脉络
Chat Paper
正在生成论文摘要