Self-Supervised Contrastive Learning for Radar-Based Human Activity Recognition
2023 IEEE RADAR CONFERENCE, RADARCONF23(2023)
摘要
Short-range radars are widely used for micro-Doppler-based human activity recognition by using the super-vised training paradigm. However, acquiring labeled trained RF data is not always possible. This paper presents a self-supervised contrastive learning (SSCL) framework that utilizes physics-aware augmented radar micro-Doppler signatures for human activity recognition. The SSCL requires two augmented views of the input data samples. For the first augmented view, the Short-time-Fourier-transform properties have been manipulated to generate Multi-Resolution micro-Doppler (MR-mD) signatures. The Second augmented view has been generated through synthesizing micro-Doppler signatures by a Physics-aware Generative adversarial Network (PhGAN). Experimental result shows that the proposed SSCL framework achieved 4% improvement over conventional unsupervised autoencoder pretraining while classifying 14 ambulatory human activities.
更多查看译文
关键词
Radar micro-Doppler,Activity recognition,Supervised training,Self-supervised learning,Data Augmentation
AI 理解论文
溯源树
样例
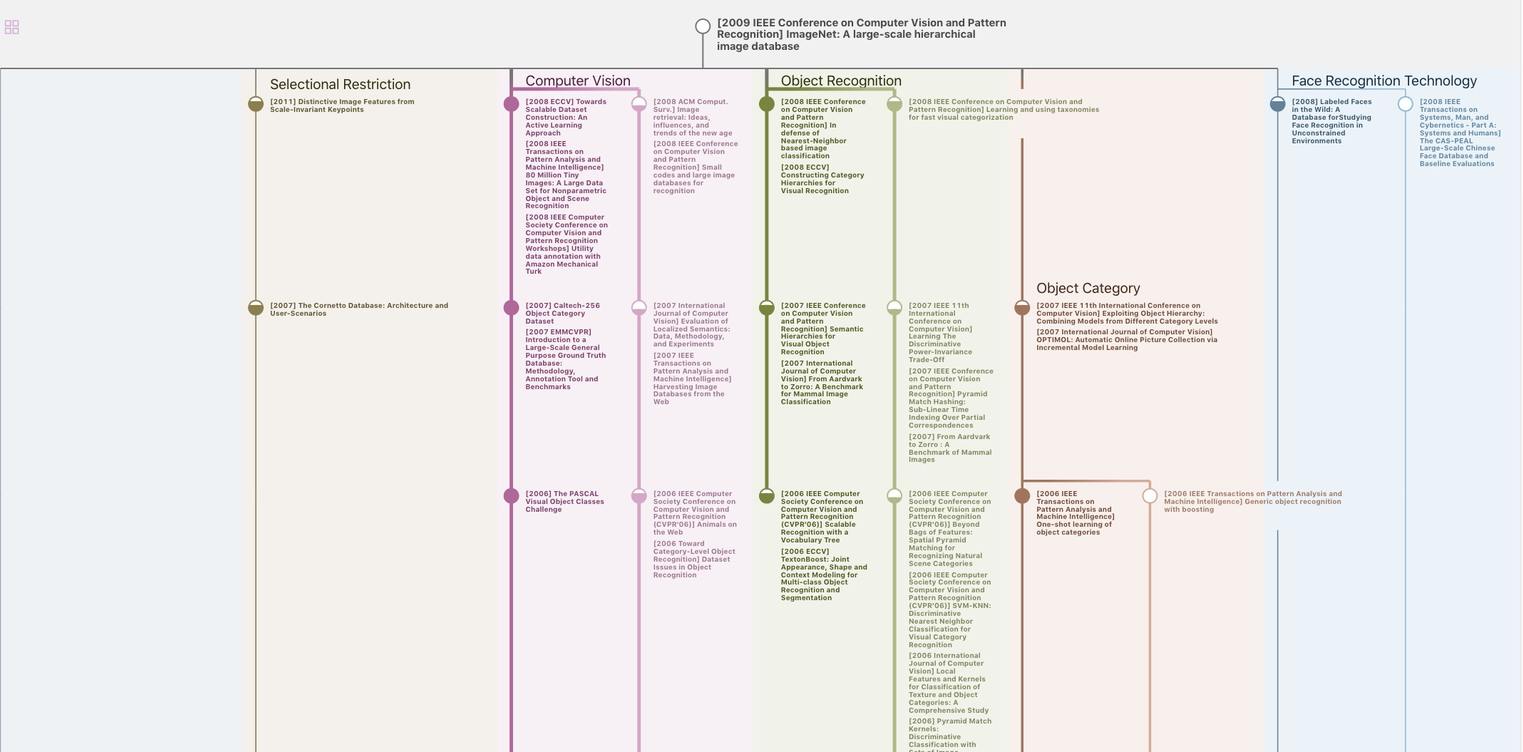
生成溯源树,研究论文发展脉络
Chat Paper
正在生成论文摘要