Learning Sufficient Correlations Among Points for 3D Non-rigid Point Cloud Registration
2023 9TH INTERNATIONAL CONFERENCE ON CONTROL, AUTOMATION AND ROBOTICS, ICCAR(2023)
摘要
Unsupervised end-to-end deep learning-based framework for non-rigid point cloud correspondence has been proposed to conveniently and effectively find the alignment relationship between two unordered point sets. However, due to the lacking of datasets in this field, researchers are still facing the challenge in designing novel neural networks which could sufficiently learn the correct correspondence and displacements of every points. In this paper, we propose a novel end-to-end unsupervised network, which consists of three modules from front to back: the feature embedding module, the correspondence prediction module and the shared deformer module. Specifically, We first use a hierarchical dilated graph convolution network(HDGCN) to boost the feature extraction as it has the potential in finding more correlations among local points. Then those features are fed into the correspondence prediction module to get the correspondence matrix, and we make it learnable in high-dimensional space. Finally, to acquire more precise point-wise displacements, we make the permuted point cloud into the one where each point carrys higher-dimensional information, and we input it to the shared deformer module which outputs the final non-rigid registration results. We name it HCDNet3D(HDGCN+Corr+Deform) and we experimentally prove our HCDNet3D makes better performance on correspondence rate and visual non-rigid registration results, comparing with several existing methods. According to the extensive experiment results, HCDNet3D outperforms state-of-the-art methods on both synthetic and real-world datasets of non-rigid 3D point clouds, including those directly computing point drifts and those taking meshes as input.
更多查看译文
关键词
point cloud non-rigid registration, dense correspondence, graph convolution
AI 理解论文
溯源树
样例
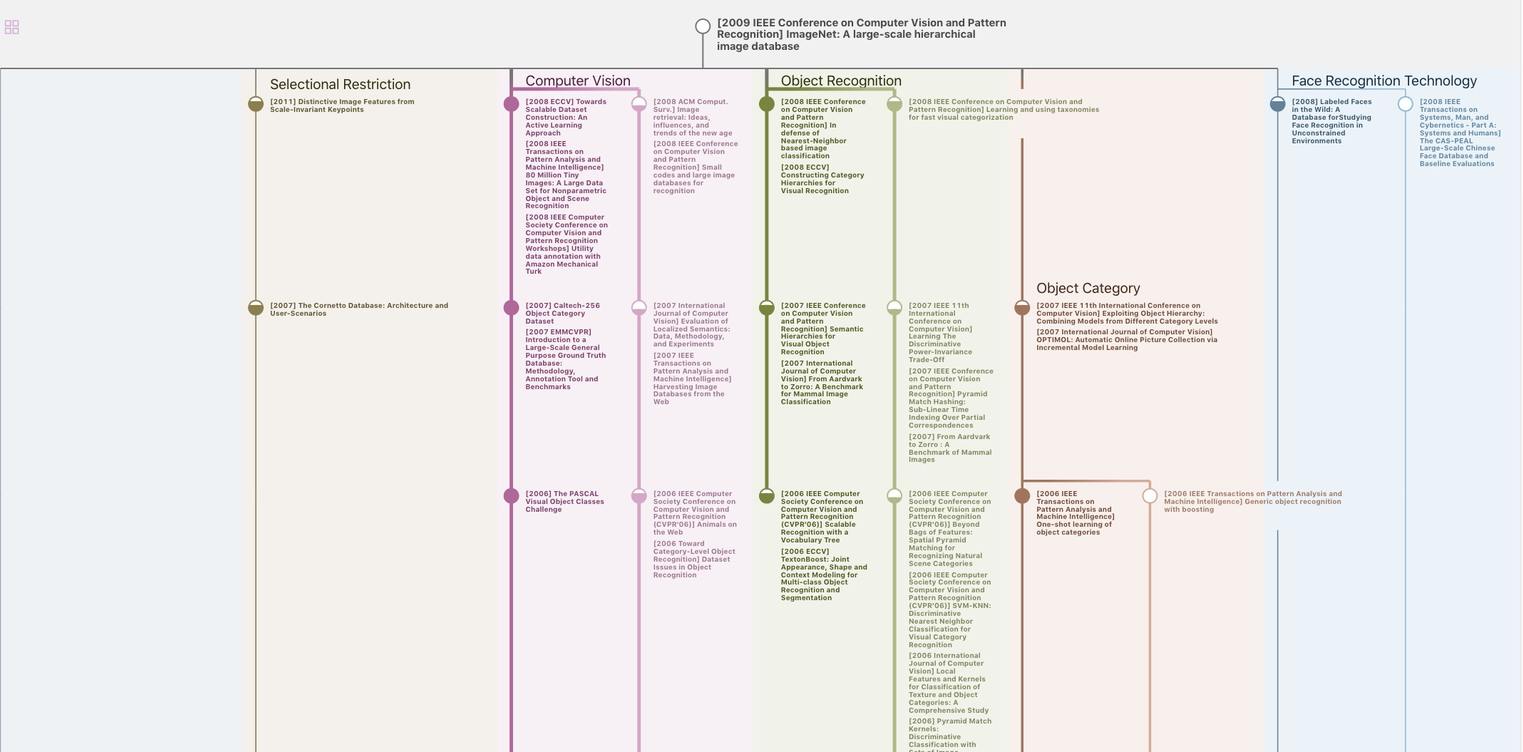
生成溯源树,研究论文发展脉络
Chat Paper
正在生成论文摘要