Spiking Neural Networks for LPI Radar Waveform Recognition with Neuromorphic Computing
2023 IEEE RADAR CONFERENCE, RADARCONF23(2023)
摘要
The ultra-wideband, frequency agile and high duty cycle features of Low Probability of Intercept (LPI) radar pose serious concerns for passive radar detection systems that must efficiently identify LPI radar modulation schemes with very low latency and high accuracy. For these tasks, conventional deep learning techniques have often been employed, though this comes at the cost of increased model complexity and high energy consumption when implemented in tactically relevant scenarios with significant channel deterioration and size, weight, and power (SWaP) constrained platforms. In this paper, we propose a neuromorphic approach based on energy-efficient and robust Spiking Neural Networks (SNNs). To demonstrate the effectiveness of our proposed approach, we perform a challenging LPI radar waveform recognition task with a Spiking Convolutional Neural Network (SCNN). Simulation results show that the SCNN achieves a 13-waveform recognition accuracy of 99.1% at 0dB SNR with 1.51 mu J of total energy per inference. It is found that our system outperforms non-spiking models with a reduction in model size and three orders of magnitude lower energy consumption. To the best of our knowledge, this work presents the first spike-based implementation of an LPI radar waveform recognition system. Moreover, this paper highlights the potential for extremely low SWaP neuromorphic hardware deployment to enable intelligent electronic support systems that demand high throughput efficiency and ultra-low power.
更多查看译文
关键词
spiking neural network,radar waveform recognition,LPI,neuromorphic computing
AI 理解论文
溯源树
样例
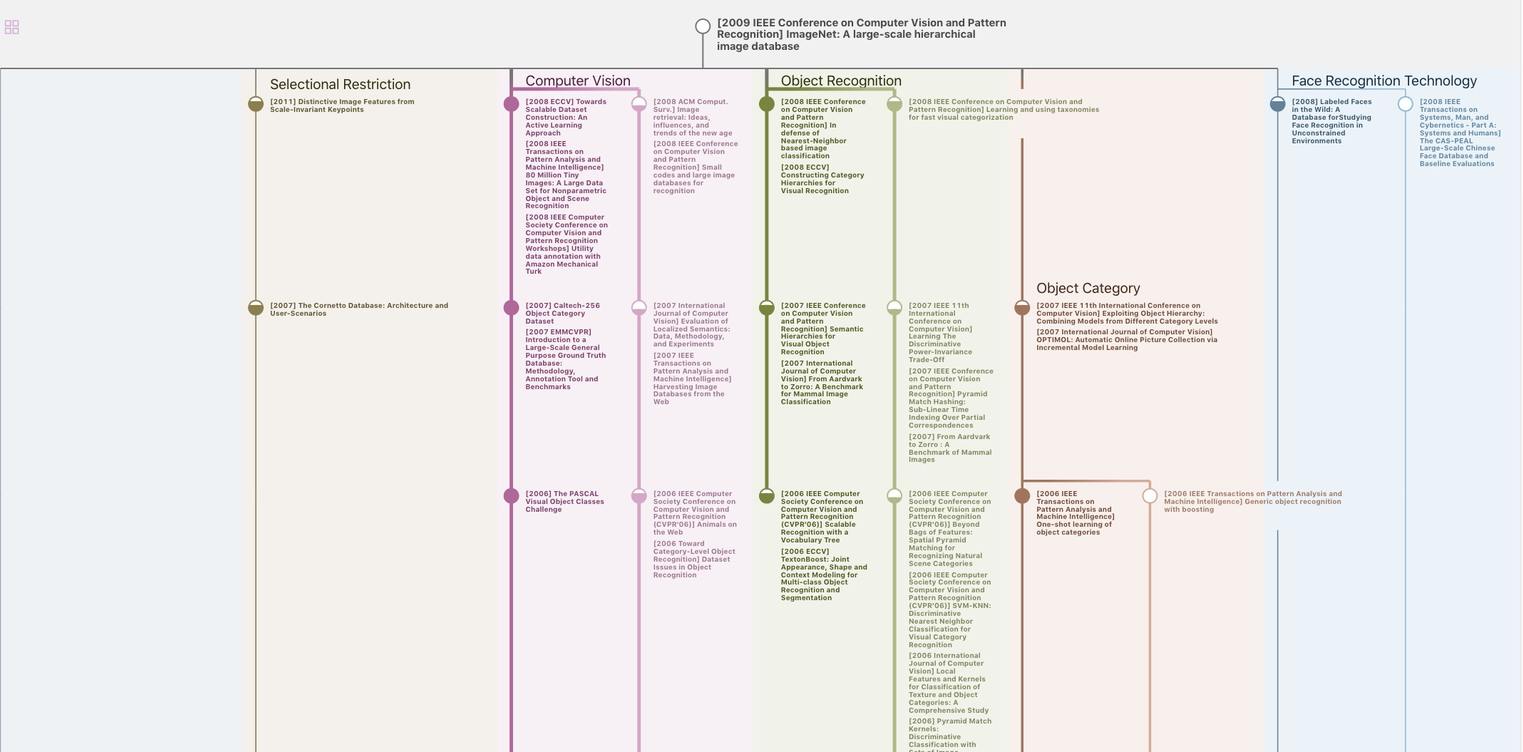
生成溯源树,研究论文发展脉络
Chat Paper
正在生成论文摘要