Complex SincNet for More Interpretable Radar Based Activity Recognition
2023 IEEE RADAR CONFERENCE, RADARCONF23(2023)
摘要
Radio frequency (RF) sensing has been increasingly used in many applications such as fall-motion recognition, human-machine interfacing, gesture controlled home appliances, and American sign language (ASL) recognition. However, most current activity classification techniques employ a two-stage process. First, the micro-Doppler spectrograms or a temporal sequence of range-Doppler (RD) maps are created. Then, in the second stage, the created representation is used with deep learning (DL) or machine learning (ML) techniques. In this paper, we propose a more interpretable complex-valued neural network architecture to directly classify human activities from the complex-valued raw radar data. The one-dimensional (1D) slow-time radar data is used as the raw radar input, and the complex sinc function is used as the first layer of the proposed model. The sinc filter is a windowed band-pass filter in which the model learns only the lower and higher frequencies of the bandpass filter. To verify our model, an American Sign Language (ASL) dataset consisting of 15 activity classes is selected. The proposed complex-valued SincNet (CV-SincNet) provides higher classification accuracy compared to direct application of a convolutional neural network (CNN) or real-valued SincNet. Enhanced results are observed compared to standard CNN models applied on the Micro-Doppler spectrogram images.
更多查看译文
关键词
Radar,RF sensing,FMCW,micro-Doppler signature,CV-SincNet,human activity recognition,ASL
AI 理解论文
溯源树
样例
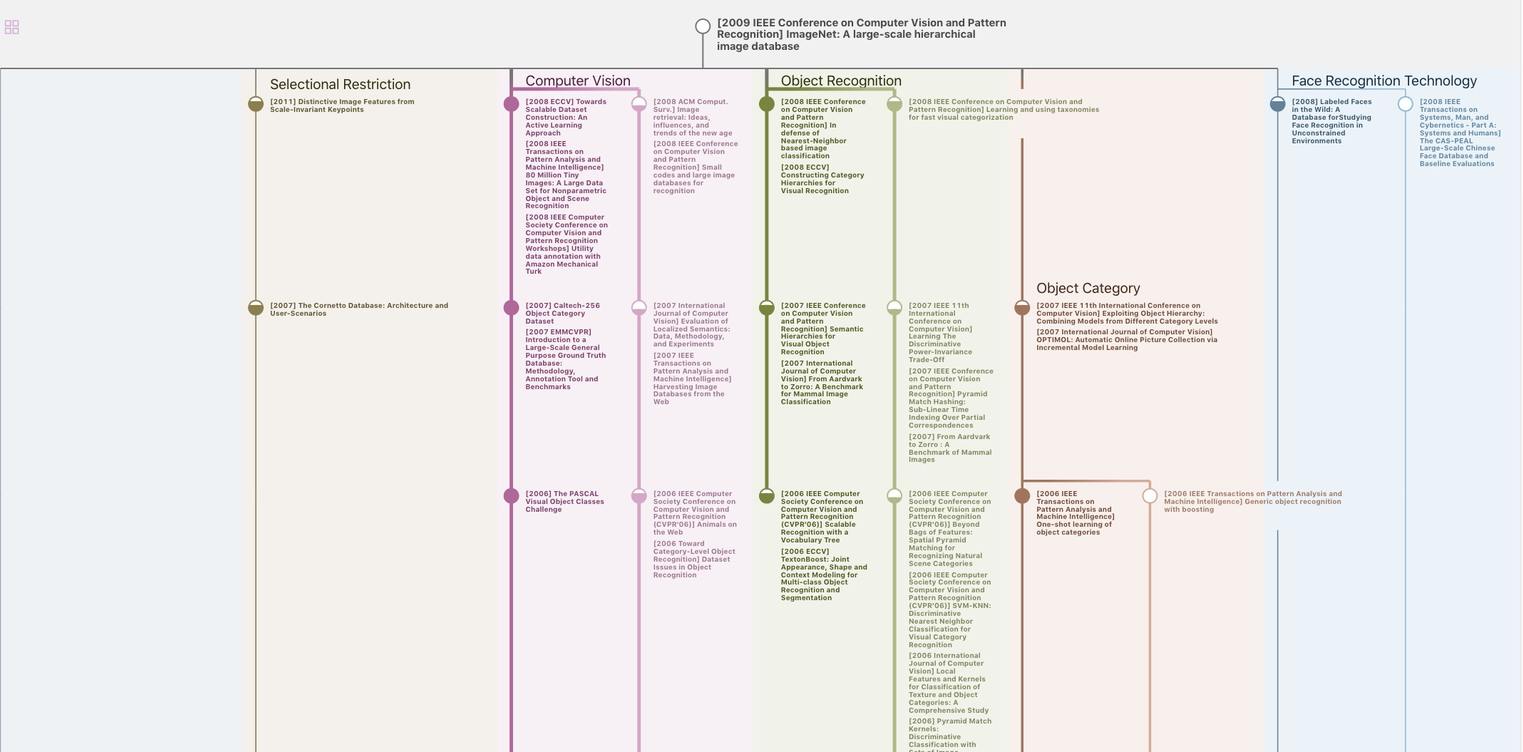
生成溯源树,研究论文发展脉络
Chat Paper
正在生成论文摘要