Radar-based Object Classification in ADAS with Hardware-Aware NAS and Input Region Scaling
2023 IEEE RADAR CONFERENCE, RADARCONF23(2023)
摘要
This paper presents a radar-based object classification algorithm for an ADAS context with contributions on two key aspects: an improved input data selection process and new neural network designs to perform the classification. The network designs are optimized via a customized Hardware-aware Neural Architecture Search process (HA-NAS) that optimizes a network for both task accuracy and execution efficiency on specific hardware. We extend and describe these novel design techniques for adaption towards radar data, which is relatively less explored than for camera or LiDAR data. Our proposed region-of-interest selection process normalises the spatial coverage of object spectra for increased input data consistency. Our NAS algorithm automatically explores variations and combinations of state-of-the-art manual designs and produces Paretocurves of optimal solutions that offer performance trade-offs and design insights. Candidate networks are evaluated automatically on real embedded hardware to measure their efficiency. Our methodology generates architectures that are 2x faster or 2% more accurate than the reference state of the art network.
更多查看译文
关键词
ADAS,Radar Object classification,Neural Architecture Search,Deep Learning
AI 理解论文
溯源树
样例
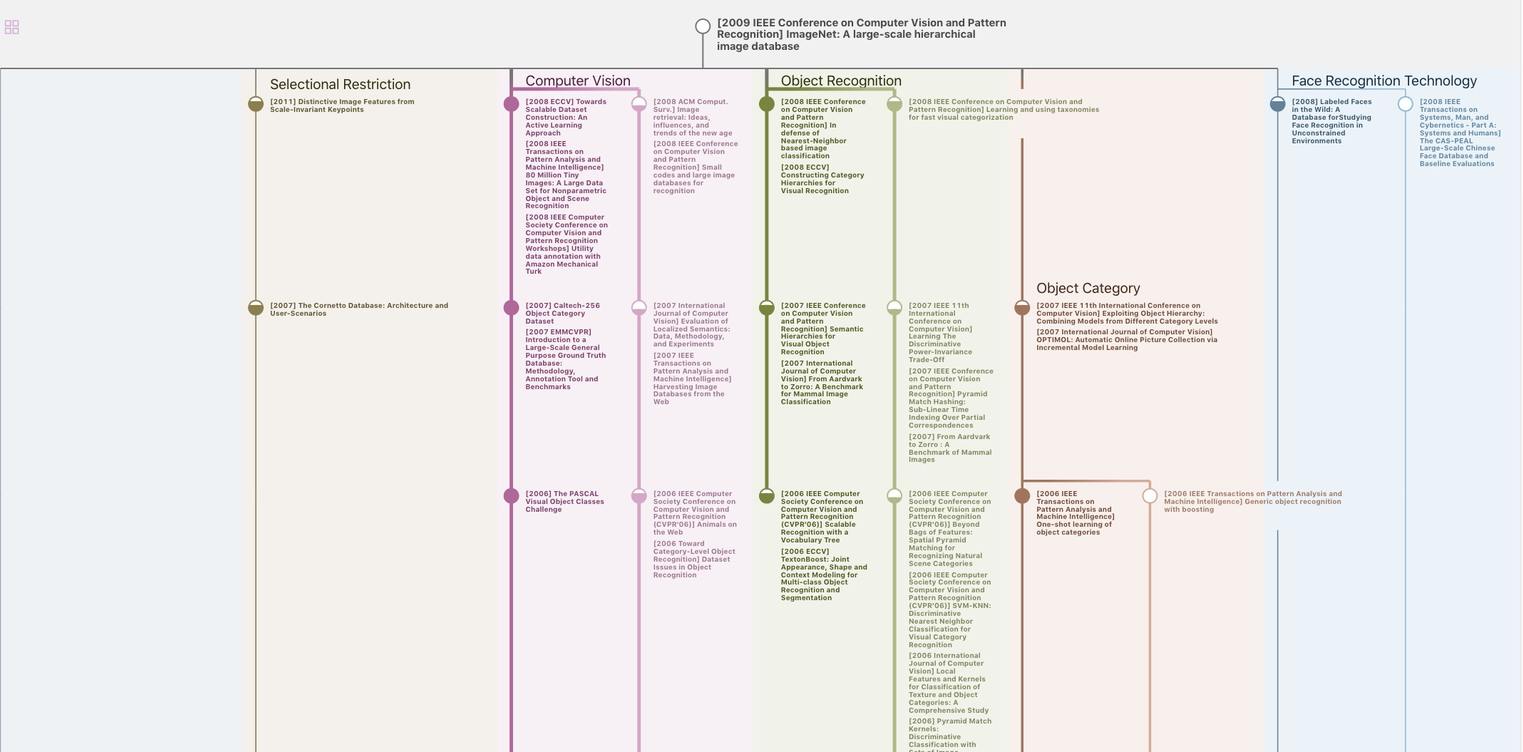
生成溯源树,研究论文发展脉络
Chat Paper
正在生成论文摘要