Optimizing Hyperparameters for Thai Cuisine Recognition via Convolutional Neural Networks
TRAITEMENT DU SIGNAL(2023)
摘要
Automated food logging is an essential component of modern dietary management, and food recognition plays a crucial role in this process. However, the recognition of dishes and food items unique to specific cultures or regions remains a less explored area. In this study, we focus on the automatic recognition of Thai cuisine, employing transfer learning techniques and comparing the performance of 20 state-of-the-art convolutional neural networks. We investigate the impact of hyperparameters, such as batch size and image resolution, as well as image preprocessing methods on classification accuracy and training time for the topperforming models. Our evaluation, using the THFOOD-50 dataset consisting of 15,688 images across 50 classes, demonstrates that the optimal model achieves top-1 and top-5 classification accuracies of 90.44% and 99.97%, respectively, representing a significant improvement over previous results. We find that increasing image resolution substantially enhances accuracy, while batch size exerts a negligible effect. Moreover, cropping the edges of images can further improve accuracy, but this technique is only effective when employing low image resolution. Our findings contribute to the development of advanced food recognition algorithms, with potential applications in dietary management and nutrition planning.
更多查看译文
关键词
food computing image recognition object,recognition Thai food
AI 理解论文
溯源树
样例
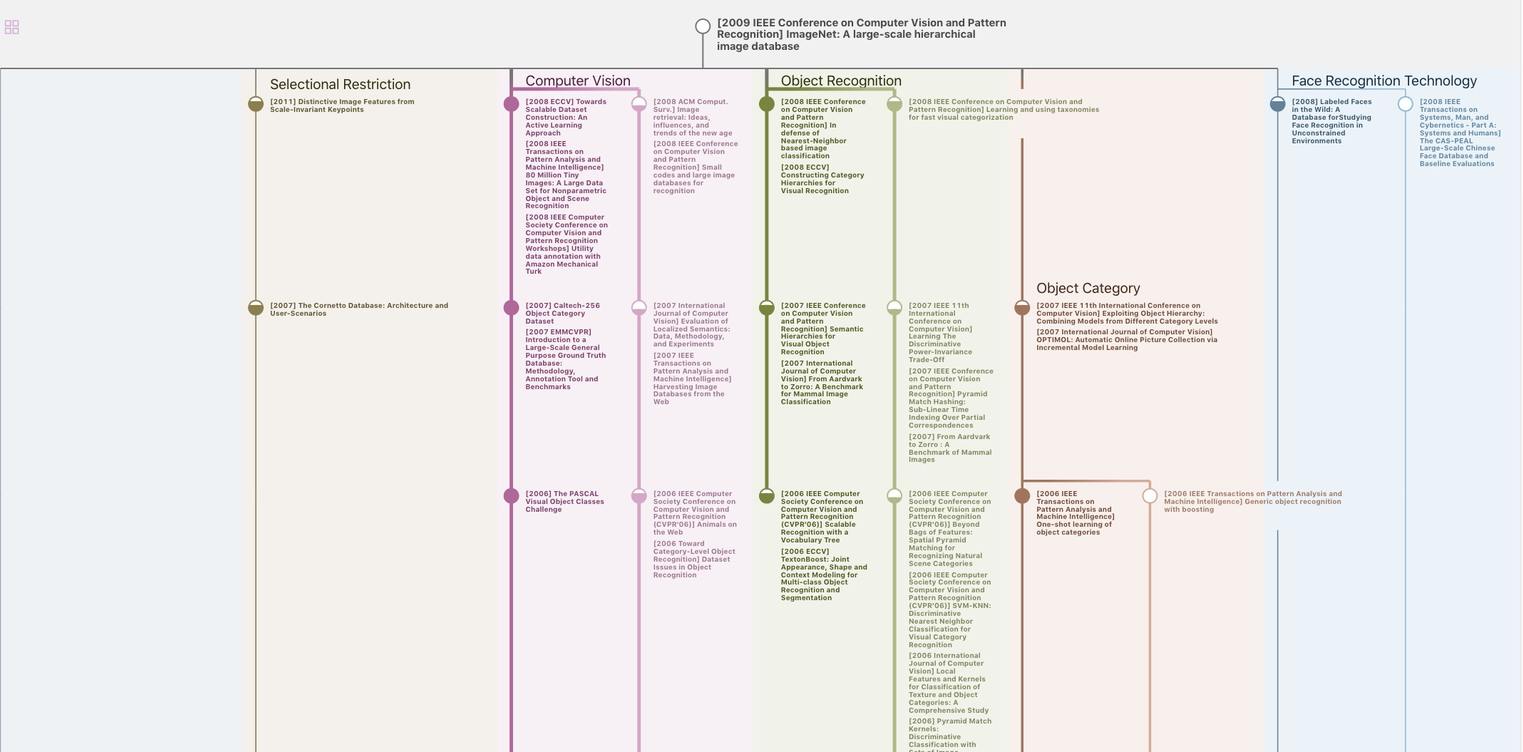
生成溯源树,研究论文发展脉络
Chat Paper
正在生成论文摘要