A Hybrid CNN-LSTM-Based Approach for Pedestrian Dead Reckoning Using Multi-Sensor-Equipped Backpack
ELECTRONICS(2023)
摘要
Researchers in academics and companies working on location-based services (LBS) are paying close attention to indoor localization based on pedestrian dead reckoning (PDR) because of its infrastructure-free localization method. PDR is the fundamental localization technique that utilize human motion to perform localization in a relative sense with respect to the initial position. The size, weight, and power consumption of micromechanical systems (MEMS) embedded into smartphones are remarkably low, making them appropriate for localization and positioning. Traditional pedestrian PDR methods predict position and orientation using stride length and continuous integration of acceleration in step and heading system (SHS)-based PDR and inertial navigation system (INS)-PDR, respectively. However, these two approaches provide accumulations of error and do not effectively leverage the inertial measurement unit (IMU) sequences. The PDR navigation solution relays on the standard of the MEMS, which yields PDR with the acceleration and angular velocity from the accelerometer and gyroscope, respectively. However, low-cost small MEMSs endure enormous error sources such as bias and noise. Hence, MEMS assessments lead to navigation solution drifts when utilized as inputs to the PDR. As a consequence, numerous methods have been proposed to mitigate and model the errors related to MEMS. Deep learning-based dead reckoning algorithms are provided to address aforementioned issues owing to the end-to-end learning framework. This paper proposes a hybrid convolutional neural network (CNN) and long short-term memory network (LSTM)-based inertial PDR system that extracts inertial measurement units (IMU) sequence features. The end-to-end learning framework is introduced to leverage the efficiency of low-cost MEMS because data-driven solutions provide more complete knowledge of the ever-increasing data volume and computational power over the filtering model approach. A CNN-LSTM model was employed to capture local spatial and temporal features. Experiments conducted on odometry datasets collected from multi-sensor backpack devices demonstrated that the proposed architecture outperformed previous traditional PDR methods, demonstrating that the root mean square error (RMSE) for the best user was 0.52 m. On the handheld smartphone-only dataset the best achieved R-2 metric was 0.49.
更多查看译文
关键词
dead reckoning,pedestrian,cnn-lstm-based,multi-sensor-equipped
AI 理解论文
溯源树
样例
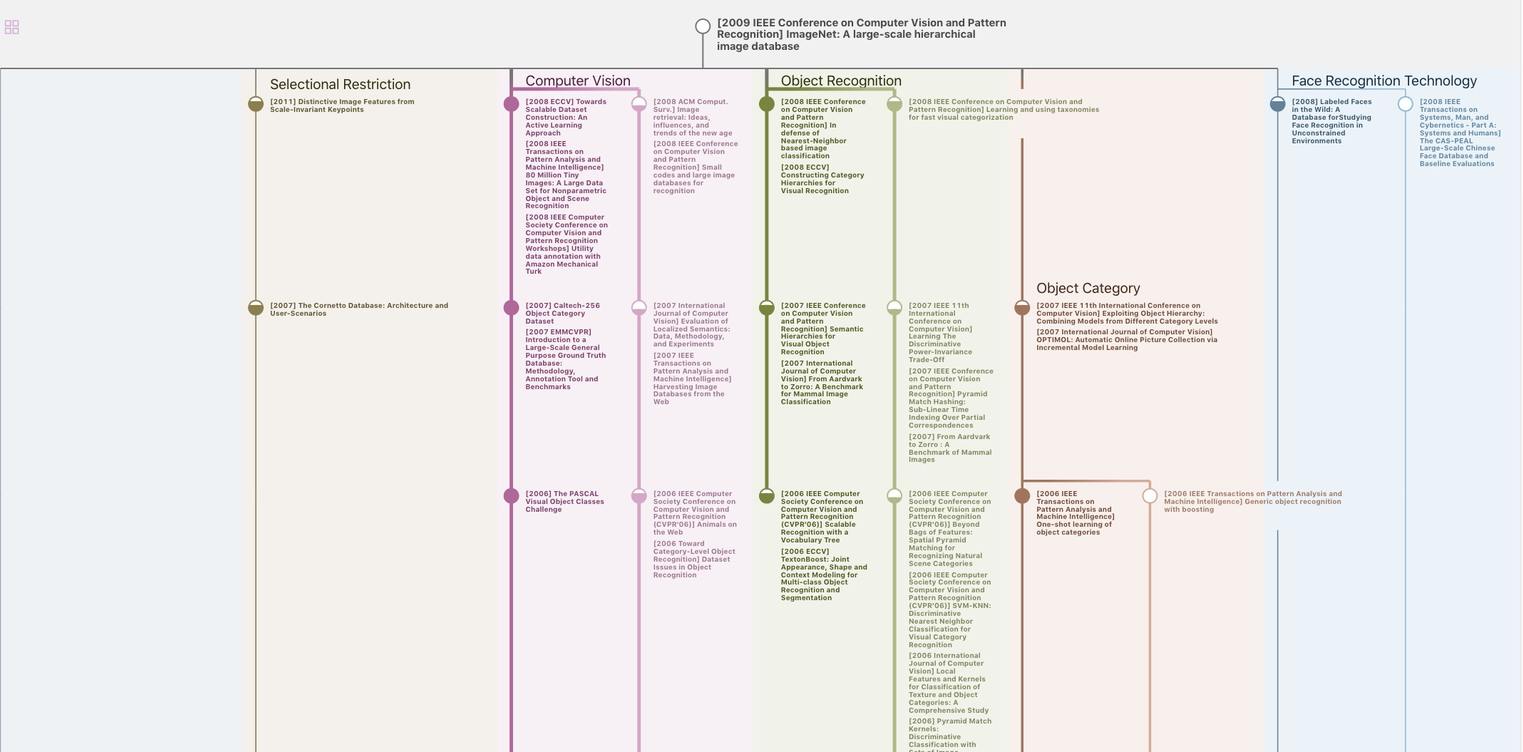
生成溯源树,研究论文发展脉络
Chat Paper
正在生成论文摘要