Deep convolutional autoencoder thermography for artwork defect detection
QUANTITATIVE INFRARED THERMOGRAPHY JOURNAL(2023)
摘要
Infrared thermography is a cost-effective non-destructive evaluation technique that plays a critical role in extracting information about defects in cultural heritage such as works of art. However, in-depth studies on the internal structure of contemporary artworks using infrared thermography remain lacking, and therefore, a deep convolutional autoencoder thermography (DCAT) data analysis method is proposed for defect detection of contemporary artworks. In the proposed method, original data are enhanced by convolution to reduce the noise and inhomogeneous background in the original thermal images; subsequently, a deep autoencoder is used to extract nonlinear features from the enhanced thermographic data, and the results of the hidden layer are visualised. These visualised images highlight information regarding the internal structure and defects of the artwork. The case study on a handmade replica of Picasso's 'La Bouteille de Suze', reproduced exactly as the original, has shown that DCAT significantly improved the accuracy of defect detection when compared with that of commonly used methods.
更多查看译文
关键词
Non-destructive testing, infrared thermography, autoencoder, noise reduction, artwork
AI 理解论文
溯源树
样例
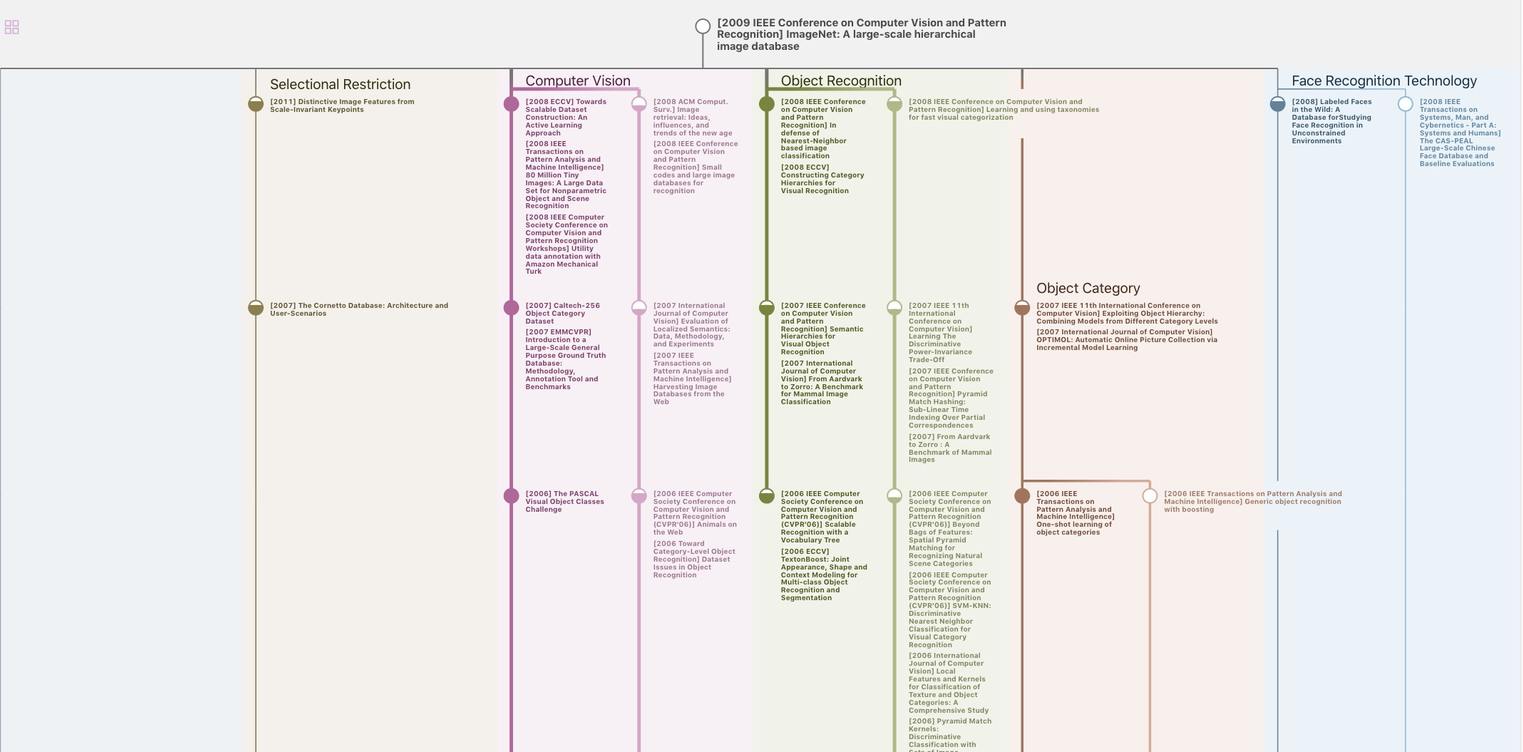
生成溯源树,研究论文发展脉络
Chat Paper
正在生成论文摘要